Mutual Guidance and Residual Integration for Image Enhancement
CoRR(2022)
摘要
Previous studies show the necessity of global and local adjustment for image enhancement. However, existing convolutional neural networks (CNNs) and transformer-based models face great challenges in balancing the computational efficiency and effectiveness of global-local information usage. Especially, existing methods typically adopt the global-to-local fusion mode, ignoring the importance of bidirectional interactions. To address those issues, we propose a novel mutual guidance network (MGN) to perform effective bidirectional global-local information exchange while keeping a compact architecture. In our design, we adopt a two-branch framework where one branch focuses more on modeling global relations while the other is committed to processing local information. Then, we develop an efficient attention-based mutual guidance approach throughout our framework for bidirectional global-local interactions. As a result, both the global and local branches can enjoy the merits of mutual information aggregation. Besides, to further refine the results produced by our MGN, we propose a novel residual integration scheme following the divide-and-conquer philosophy. The extensive experiments demonstrate the effectiveness of our proposed method, which achieves state-of-the-art performance on several public image enhancement benchmarks.
更多查看译文
关键词
image enhancement,residual integration,guidance
AI 理解论文
溯源树
样例
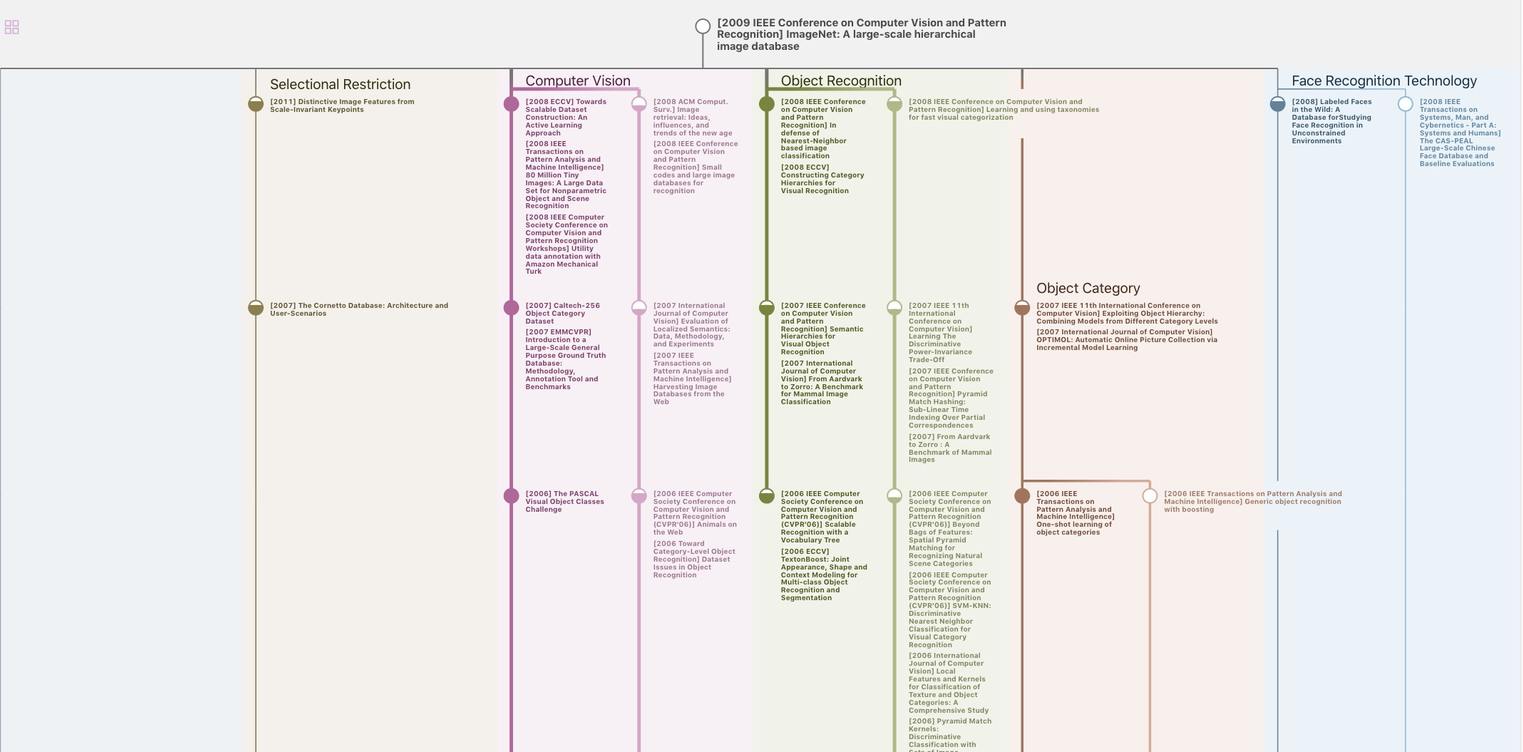
生成溯源树,研究论文发展脉络
Chat Paper
正在生成论文摘要