A Gaussian Process Regression based Dynamical Models Learning Algorithm for Target Tracking
arxiv(2022)
摘要
Maneuvering target tracking is a challenging problem for sensor systems because of the unpredictability of the targets' motions. This paper proposes a novel data-driven method for learning the dynamical motion model of a target. Non-parametric Gaussian process regression (GPR) is used to learn a target's naturally shift invariant motion (NSIM) behavior, which is translationally invariant and does not need to be constantly updated as the target moves. The learned Gaussian processes (GPs) can be applied to track targets within different surveillance regions from the surveillance region of the training data by being incorporated into the particle filter (PF) implementation. The performance of our proposed approach is evaluated over different maneuvering scenarios by being compared with commonly used interacting multiple model (IMM)-PF methods and provides around $90\%$ performance improvement for a multi-target tracking (MTT) highly maneuvering scenario.
更多查看译文
关键词
gaussian process regression,target tracking,dynamical models learning algorithm
AI 理解论文
溯源树
样例
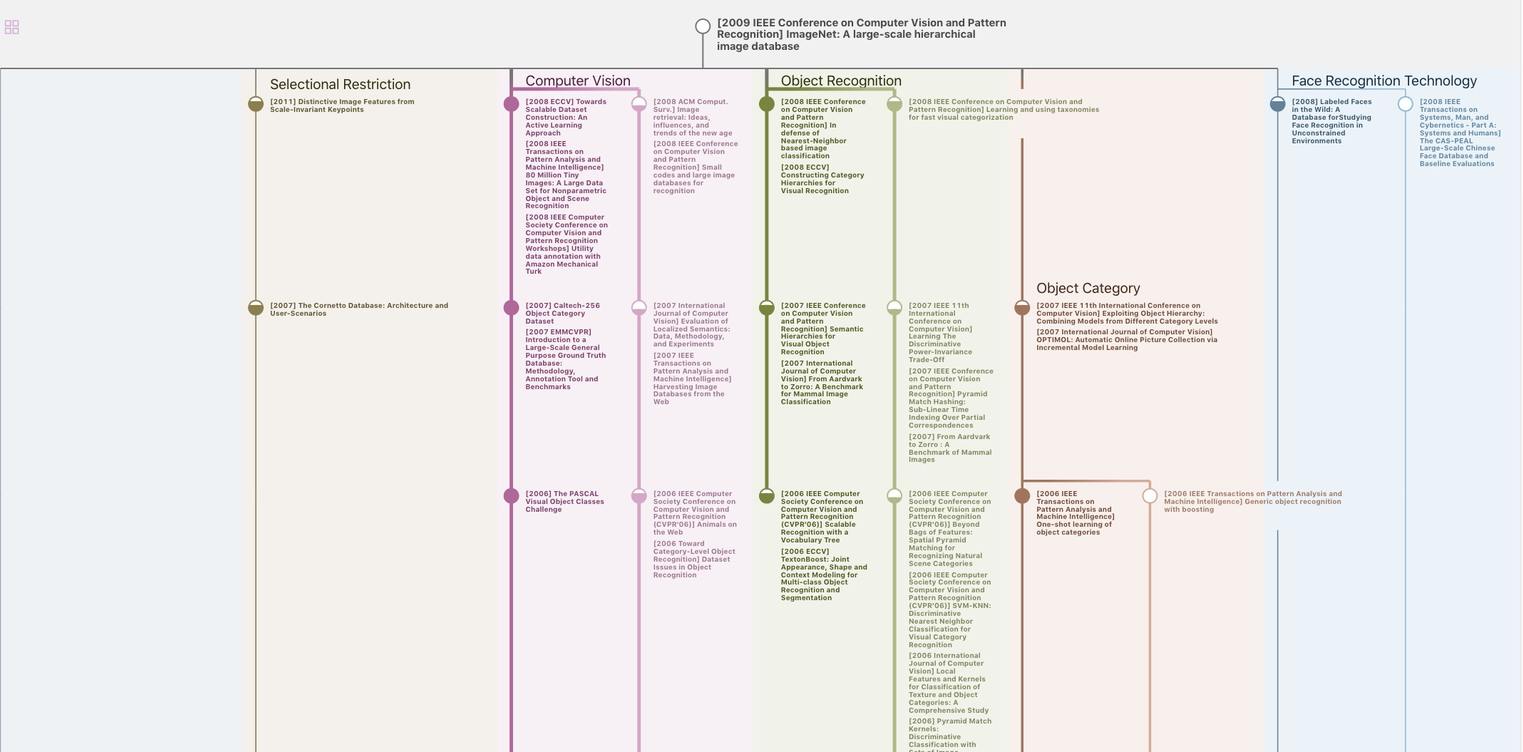
生成溯源树,研究论文发展脉络
Chat Paper
正在生成论文摘要