Optical-SAR Decision Fusion with Markov Random Fields for High-Resolution Large-Scale Land Cover Mapping.
IEEE International Geoscience and Remote Sensing Symposium (IGARSS)(2022)
摘要
Decision fusion allows making a common decision by combining multiple opinions. In the context of remote sensing classification, such techniques are of great importance in all the cases where data collected by multiple sensors are merged into a final decision. Decision fusion may be used to combine the posterior probabilities associated with the output of single classifiers when applied to single sensor data. Meanwhile, techniques such as Markov Random Fields (MRFs) can integrate contextual information in the fusion process and are commonly used in classification. However, in the context of very large scale mapping (e.g., for global climate change monitoring), computation time can be critical and the application of both data fusion and spatial-contextual modeling comes with several constraints. In this paper, we propose a Bayesian decision fusion approach for optical-SAR image classification, integrated with a fast formulation of the iterated conditional modes (ICM) MRF-optimization algorithm based on a convolution operation. he validation on wide areas of Siberia proved the scalability and efficiency of the method for large scale applications.
更多查看译文
关键词
markov random fields,optical-sar,high-resolution,large-scale
AI 理解论文
溯源树
样例
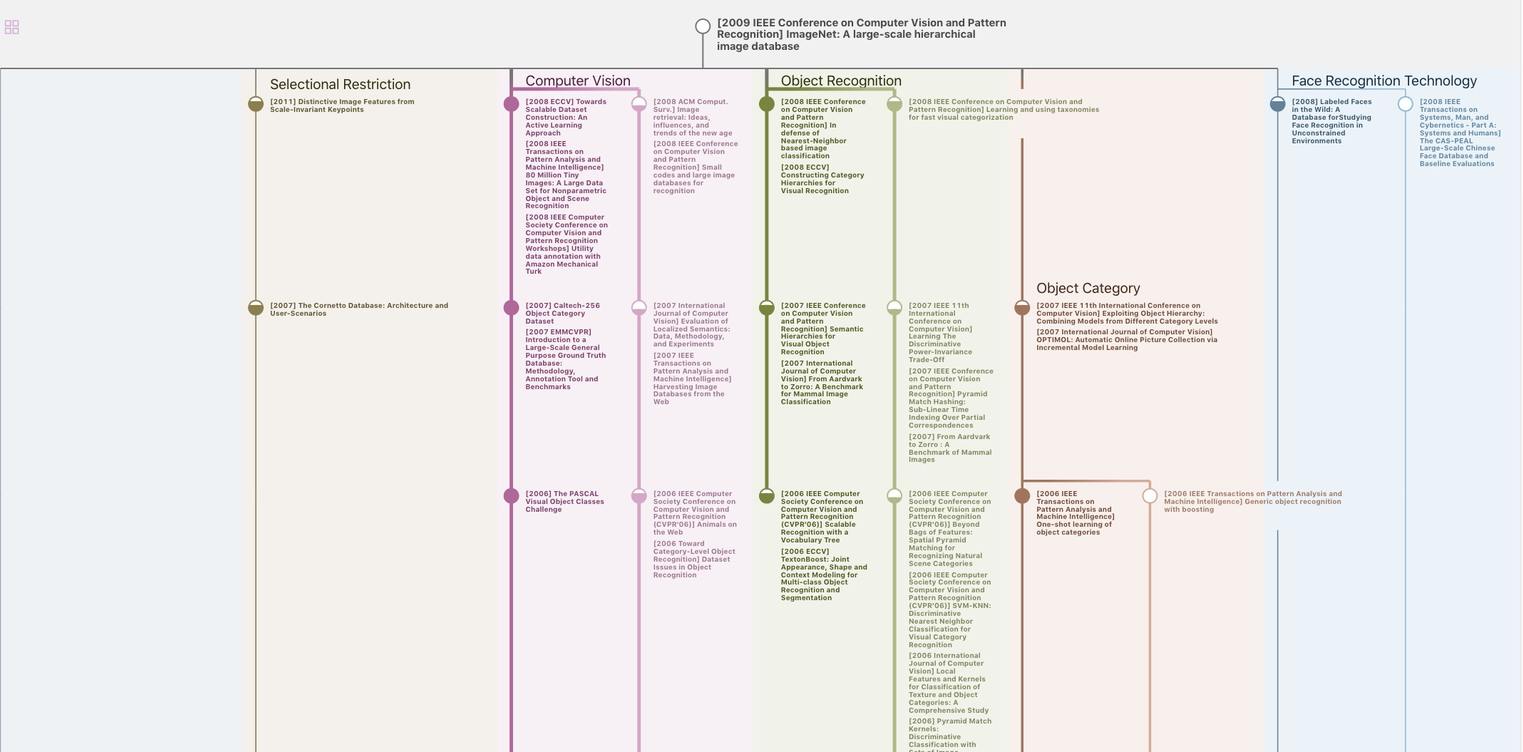
生成溯源树,研究论文发展脉络
Chat Paper
正在生成论文摘要