Bayesian sparse joint dynamic topic model with flexible lead-lag order
Information Sciences(2022)
摘要
Currently, text documents from multiple sources have become available in many fields. It is of great interest to study the relationship between documents from different sources and uncover the underlying causality. Zhu et al. (2021) proposed a joint dynamic topic model (JDTM). They classified all topics into three groups and used the “shared topics” with a fixed time lag order to characterize the shared information between two corpora. Although JDTM is a powerful tool for discovering the lead-lag relationship, there are two potential shortcomings. First, different shared topics should have distinct meanings, which should lead to different time lag orders between the two corpora. Second, for dynamic documents, not all topics are represented in each time slice, and thus topic sparsity should be considered. To address these two problems, we propose a sparse joint dynamic topic model (SJDTM) with a flexible lead-lag order. We assume a birth-and-death mechanism for all topics and a flexible lead-lag order for different shared topics. The performance of SJDTM is evaluated using both synthetic data and two real text corpora consisting of conference papers and journal papers.
更多查看译文
关键词
Dynamic topic model,Lead-lag relationship,Topic evolution,Sparse modeling
AI 理解论文
溯源树
样例
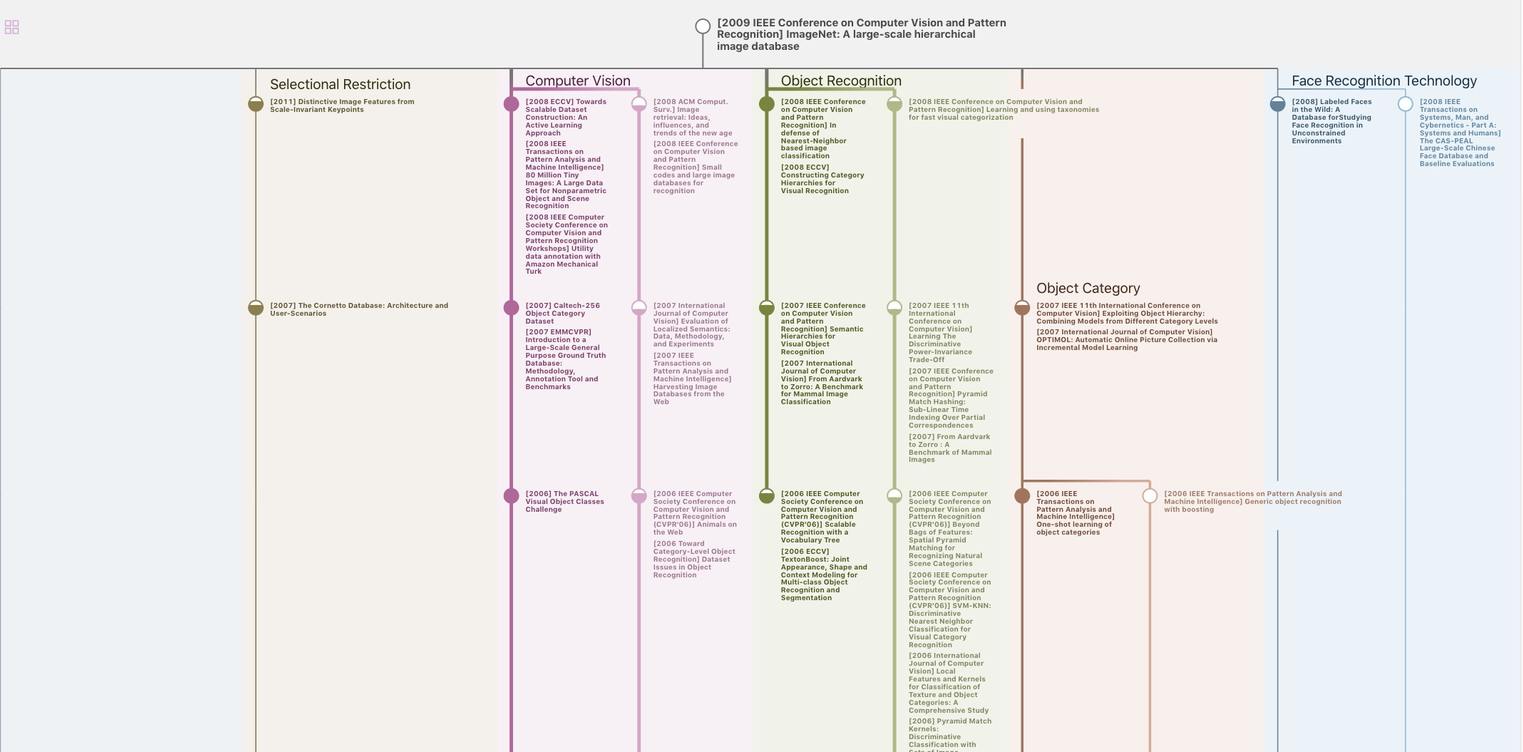
生成溯源树,研究论文发展脉络
Chat Paper
正在生成论文摘要