Early Diagnosis of Alzheimer's Disease Using Deep Learning
Proceedings of the 2nd International Conference on Control and Computer Vision(2019)
摘要
Alzheimer's disease (AD) leads to memory loss and impairment, which may cause further symptoms. It affects lives of patients seriously and is not curable, but early confirmation of AD may be helpful to start proper treatment so as to avoid further brain damage. Over the past decades, machine learning methods have been applied to the classification of AD with results based on manually prepared features and a classifier having a multiple-step architecture. Recently, with the development of deep learning, the end to- end process of neural networks has been employed for pattern classification. In this paper, we focus on early diagnosis of AD based on convolutional neural networks (ConvNets) by using magnetic resonance imaging (MRI). Image slices of gray matter and white matter from MRI have been used as the inputs for classification. Ensemble learning methods have been employed after the convolutional operations for improving the classification by combining outputs of deep learning classifiers [27]. Three base ConvNets were designed, implemented, and compared in this paper. Our method was evaluated based on a dataset from the Alzheimer's Disease Neuroimaging Initiative for the early diagnosis of this illness. In particular, the accuracy rates of our classifications have reached up to 97.65% for AD/mild cognitive impairment and 88.37% for mild cognitive impairment/normal control.
更多查看译文
关键词
Alzheimer's disease, ConvNets, ensemble learning, magnetic resonance imaging
AI 理解论文
溯源树
样例
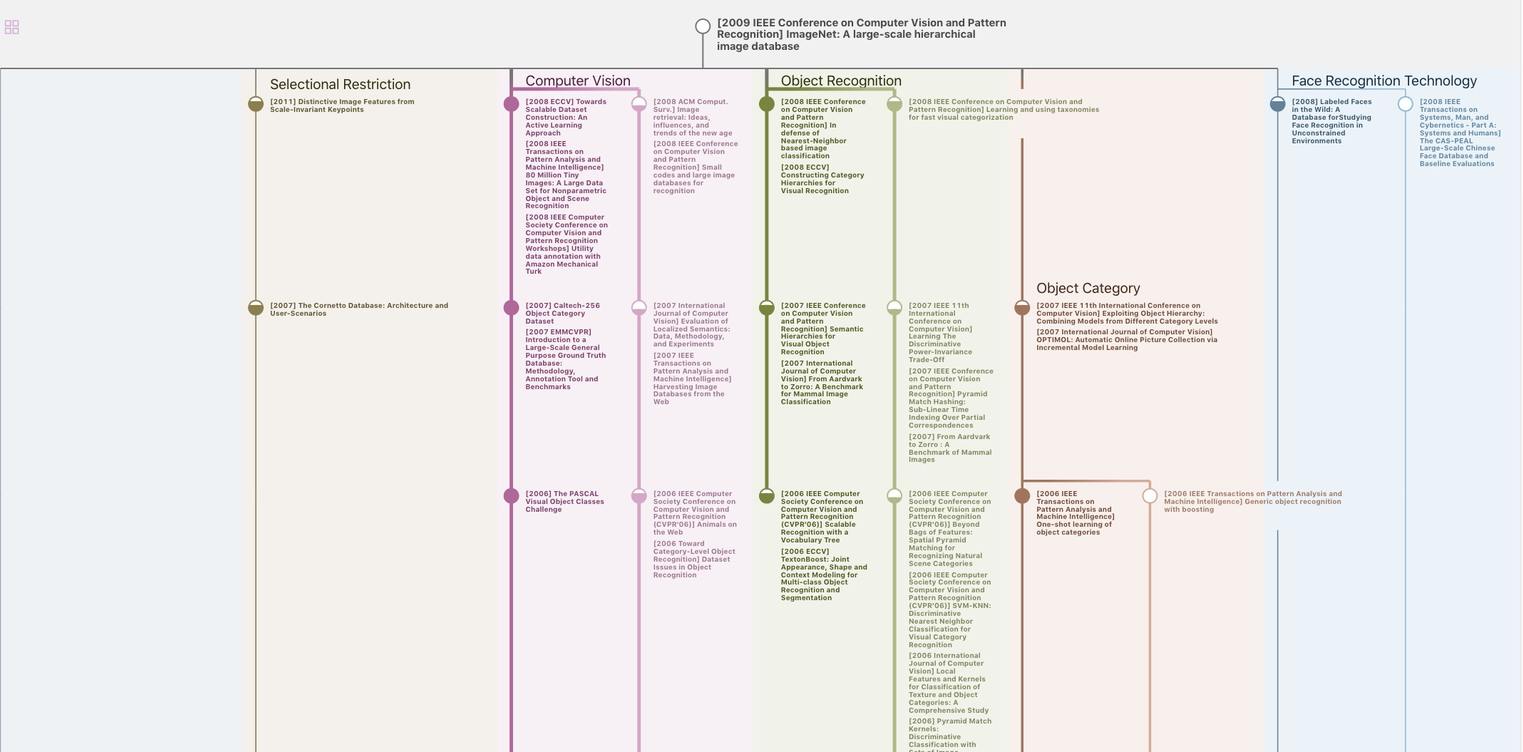
生成溯源树,研究论文发展脉络
Chat Paper
正在生成论文摘要