Predicting the occurrence of Parkinson’s Disease using various Classification Models
2018 International Conference on Advanced Computation and Telecommunication (ICACAT)(2018)
摘要
Parkinson is a disease that directly degrades the functioning of central nervous system, more specifically the motor system. If diagnosed in a later stage, this disease may become incurable. Hence, it is necessary to diagnose the disease at an early stage. Voice frequency plays a vital role in the prediction of Parkinson disease. This paper presents the study for the diagnosis of Parkinson disease using various machine learning algorithms through the amount of voice data attained from UCI repository [1]. The voice dataset consists of voice frequencies of 31 people with early-stage Parkinson's disease recruited to a six-month trial of a telemonitoring device for remote symptom progression monitoring. Various machine learning algorithms were applied on the dataset and among them ANN has shown highest accuracy (94.87%). Random Forest which is a Classification algorithm has shown good accuracy (87.17%) while Naïve Bayes has shown least accuracy (71.79%). We have summarized all the results using the confusion matrix.
更多查看译文
关键词
Voice dataset,SVM,ANN,Random forest
AI 理解论文
溯源树
样例
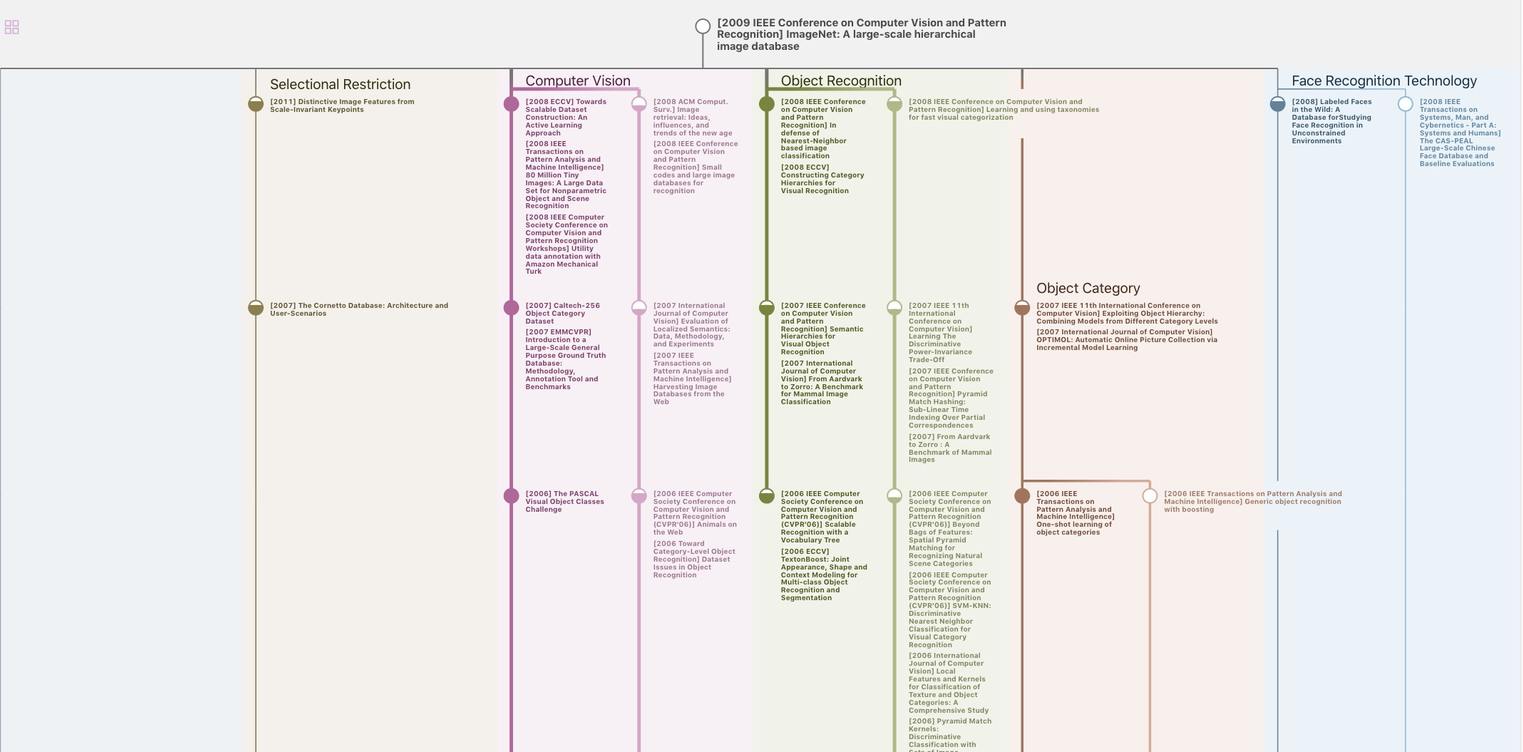
生成溯源树,研究论文发展脉络
Chat Paper
正在生成论文摘要