A physics-informed diffusion model for high-fidelity flow field reconstruction
Journal of Computational Physics(2023)
摘要
Machine learning models are gaining increasing popularity in the domain of fluid dynamics for their potential to accelerate the production of high-fidelity computational fluid dynamics data. However, many recently proposed machine learning models for high-fidelity data reconstruction require low-fidelity data for model training. Such requirement restrains the application performance of these models, since their data reconstruction accuracy would drop significantly if the low-fidelity input data used in model test has a large deviation from the training data. To overcome this restraint, we propose a diffusion model which only uses high-fidelity data at training. With different configurations, our model is able to reconstruct high-fidelity data from either a regular low-fidelity sample or a randomly measured sample, and is also able to gain an accuracy increase by using physics-informed conditioning information from a known partial differential equation when that is available. Experimental results demonstrate that our model can produce accurate reconstruction results for 2d turbulent flows based on different input sources without retraining.(c) 2023 The Author(s). Published by Elsevier Inc. This is an open access article under the CC BY-NC-ND license (http://creativecommons .org /licenses /by-nc -nd /4 .0/).
更多查看译文
关键词
Denoising diffusion probabilistic models,Computational fluid dynamics,Super-resolution
AI 理解论文
溯源树
样例
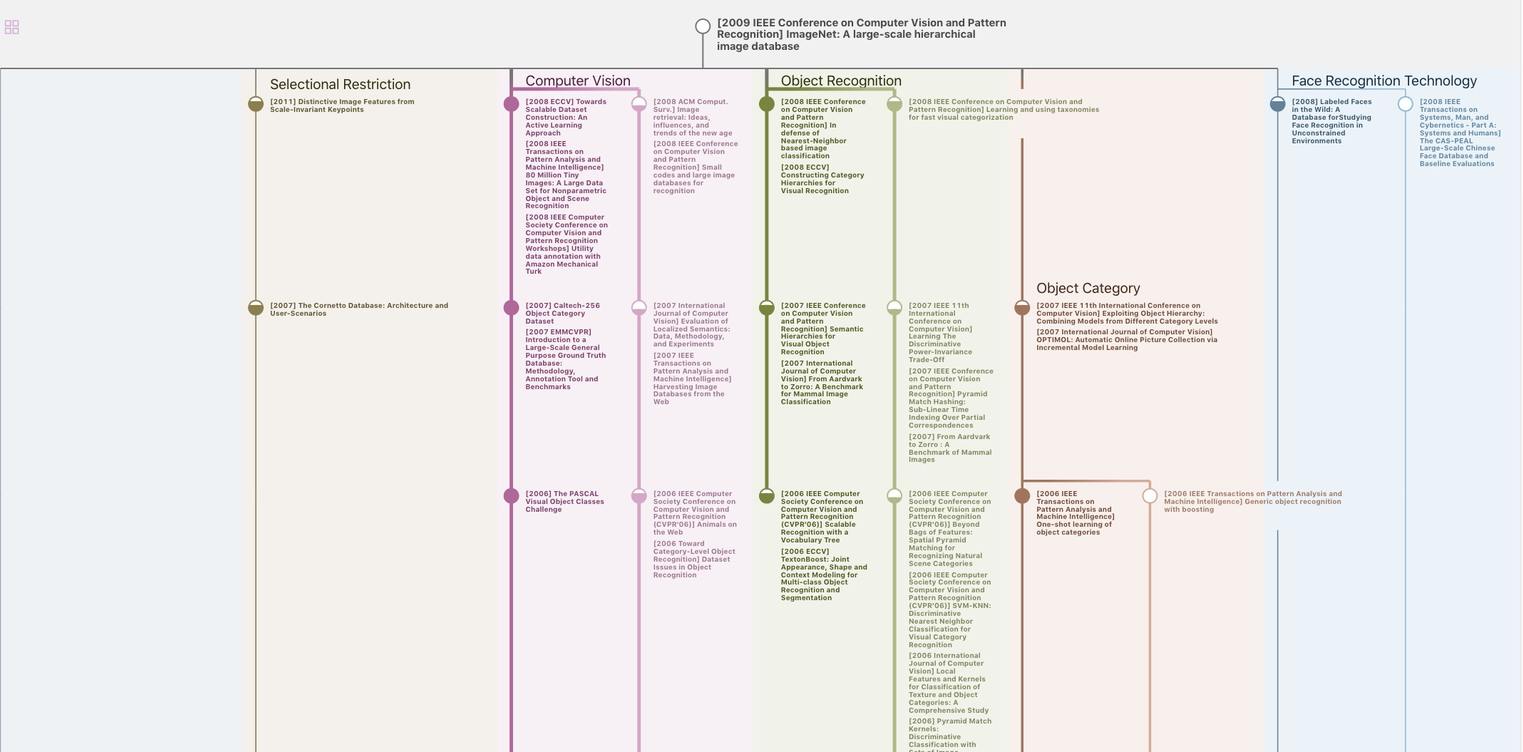
生成溯源树,研究论文发展脉络
Chat Paper
正在生成论文摘要