Detecting changes in the transmission rate of a stochastic epidemic model
STATISTICS IN MEDICINE(2024)
Abstract
Throughout the course of an epidemic, the rate at which disease spreads varies with behavioral changes, the emergence of new disease variants, and the introduction of mitigation policies. Estimating such changes in transmission rates can help us better model and predict the dynamics of an epidemic, and provide insight into the efficacy of control and intervention strategies. We present a method for likelihood-based estimation of parameters in the stochastic susceptible-infected-removed model under a time-inhomogeneous transmission rate comprised of piecewise constant components. In doing so, our method simultaneously learns change points in the transmission rate via a Markov chain Monte Carlo algorithm. The method targets the exact model posterior in a difficult missing data setting given only partially observed case counts over time. We validate performance on simulated data before applying our approach to data from an Ebola outbreak in Western Africa and COVID-19 outbreak on a university campus.
MoreTranslated text
Key words
Bayesian change point detection,COVID-19,data-augmented MCMC,incidence data,likelihood-based inference,stochastic epidemic models
AI Read Science
Must-Reading Tree
Example
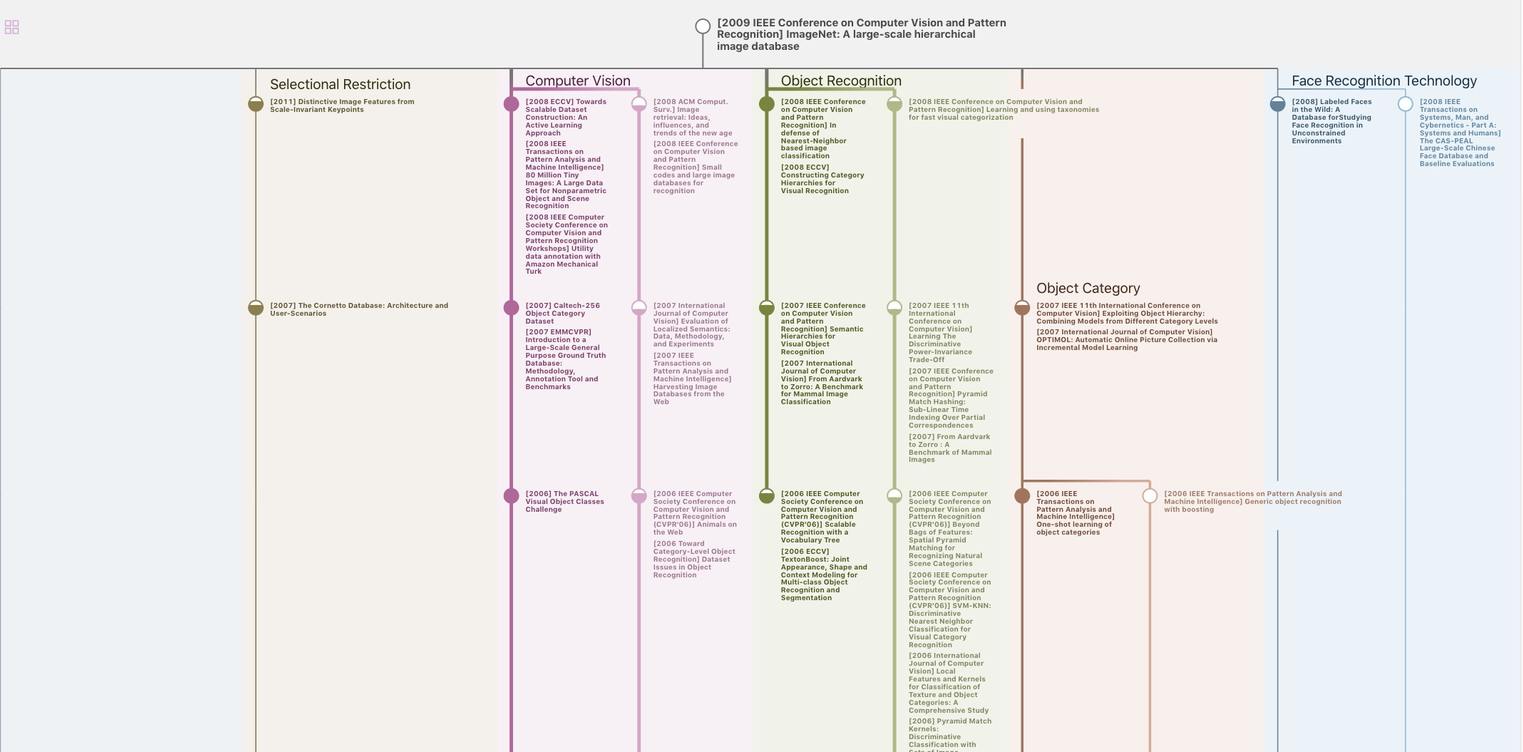
Generate MRT to find the research sequence of this paper
Chat Paper
Summary is being generated by the instructions you defined