Detect-Localize-Repair: A Unified Framework for Learning to Debug with CodeT5
Conference on Empirical Methods in Natural Language Processing (EMNLP)(2022)CCF B
Abstract
Automated software debugging is a crucial task for improving the productivity of software developers. Many neural-based techniques have been proven effective for debugging-related tasks such as bug localization and program repair (or bug fixing). However, these techniques often focus only on either one of them or approach them in a stage-wise manner, ignoring the mutual benefits between them. In this work, we propose a novel unified Detect-Localize-Repair framework based on a pretrained programming language model CodeT5 to seamlessly address these tasks, named CodeT5-DLR. Specifically, we propose three objectives to adapt the generic CodeT5 for debugging: a bug detection objective to determine whether a given code snippet is buggy or not, a bug localization objective to identify the buggy lines, and a program repair objective to translate the buggy code to its fixed version. We evaluate it on each of these tasks and their combined setting on two newly collected line-level debugging datasets in Java and Python. Extensive results show that our model significantly outperforms existing baselines from both NLP and software engineering domains.
MoreTranslated text
Key words
Bug Localization,Software Fault Localization,Fault Localization,Software Defect Prediction,Code Clone Detection
PDF
View via Publisher
AI Read Science
AI Summary
AI Summary is the key point extracted automatically understanding the full text of the paper, including the background, methods, results, conclusions, icons and other key content, so that you can get the outline of the paper at a glance.
Example
Background
Key content
Introduction
Methods
Results
Related work
Fund
Key content
- Pretraining has recently greatly promoted the development of natural language processing (NLP)
- We show that M6 outperforms the baselines in multimodal downstream tasks, and the large M6 with 10 parameters can reach a better performance
- We propose a method called M6 that is able to process information of multiple modalities and perform both single-modal and cross-modal understanding and generation
- The model is scaled to large model with 10 billion parameters with sophisticated deployment, and the 10 -parameter M6-large is the largest pretrained model in Chinese
- Experimental results show that our proposed M6 outperforms the baseline in a number of downstream tasks concerning both single modality and multiple modalities We will continue the pretraining of extremely large models by increasing data to explore the limit of its performance
Try using models to generate summary,it takes about 60s
Must-Reading Tree
Example
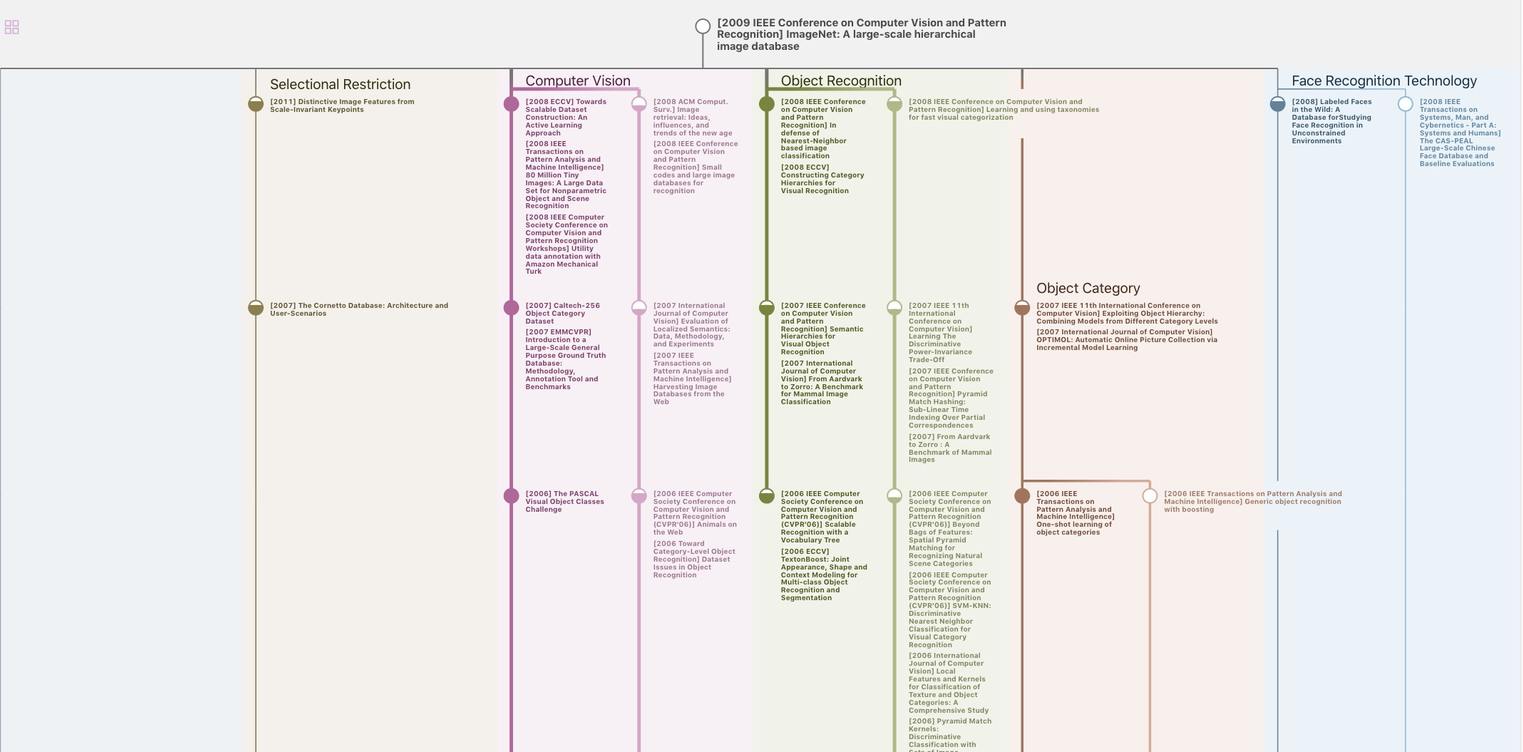
Generate MRT to find the research sequence of this paper
Related Papers
Unifying Defect Prediction, Categorization, and Repair by Multi-Task Deep Learning
2023 38TH IEEE/ACM INTERNATIONAL CONFERENCE ON AUTOMATED SOFTWARE ENGINEERING, ASE 2023
被引用0
When is Tree Search Useful for LLM Planning? It Depends on the Discriminator
Annual Meeting of the Association for Computational Linguistics 2024
被引用2
S3LLM: Large-Scale Scientific Software Understanding with LLMs Using Source, Metadata, and Document
COMPUTATIONAL SCIENCE, ICCS 2024, PT III 2024
被引用0
Data Disclaimer
The page data are from open Internet sources, cooperative publishers and automatic analysis results through AI technology. We do not make any commitments and guarantees for the validity, accuracy, correctness, reliability, completeness and timeliness of the page data. If you have any questions, please contact us by email: report@aminer.cn
Chat Paper
去 AI 文献库 对话