Arguments to Key Points Mapping with Prompt-based Learning
arxiv(2022)
摘要
Handling and digesting a huge amount of information in an efficient manner has been a long-term demand in modern society. Some solutions to map key points (short textual summaries capturing essential information and filtering redundancies) to a large number of arguments/opinions have been provided recently (Bar-Haim et al., 2020). To complement the full picture of the argument-to-keypoint mapping task, we mainly propose two approaches in this paper. The first approach is to incorporate prompt engineering for fine-tuning the pre-trained language models (PLMs). The second approach utilizes prompt-based learning in PLMs to generate intermediary texts, which are then combined with the original argument-keypoint pairs and fed as inputs to a classifier, thereby mapping them. Furthermore, we extend the experiments to cross/in-domain to conduct an in-depth analysis. In our evaluation, we find that i) using prompt engineering in a more direct way (Approach 1) can yield promising results and improve the performance; ii) Approach 2 performs considerably worse than Approach 1 due to the negation issue of the PLM.
更多查看译文
关键词
key points mapping,learning,arguments,prompt-based
AI 理解论文
溯源树
样例
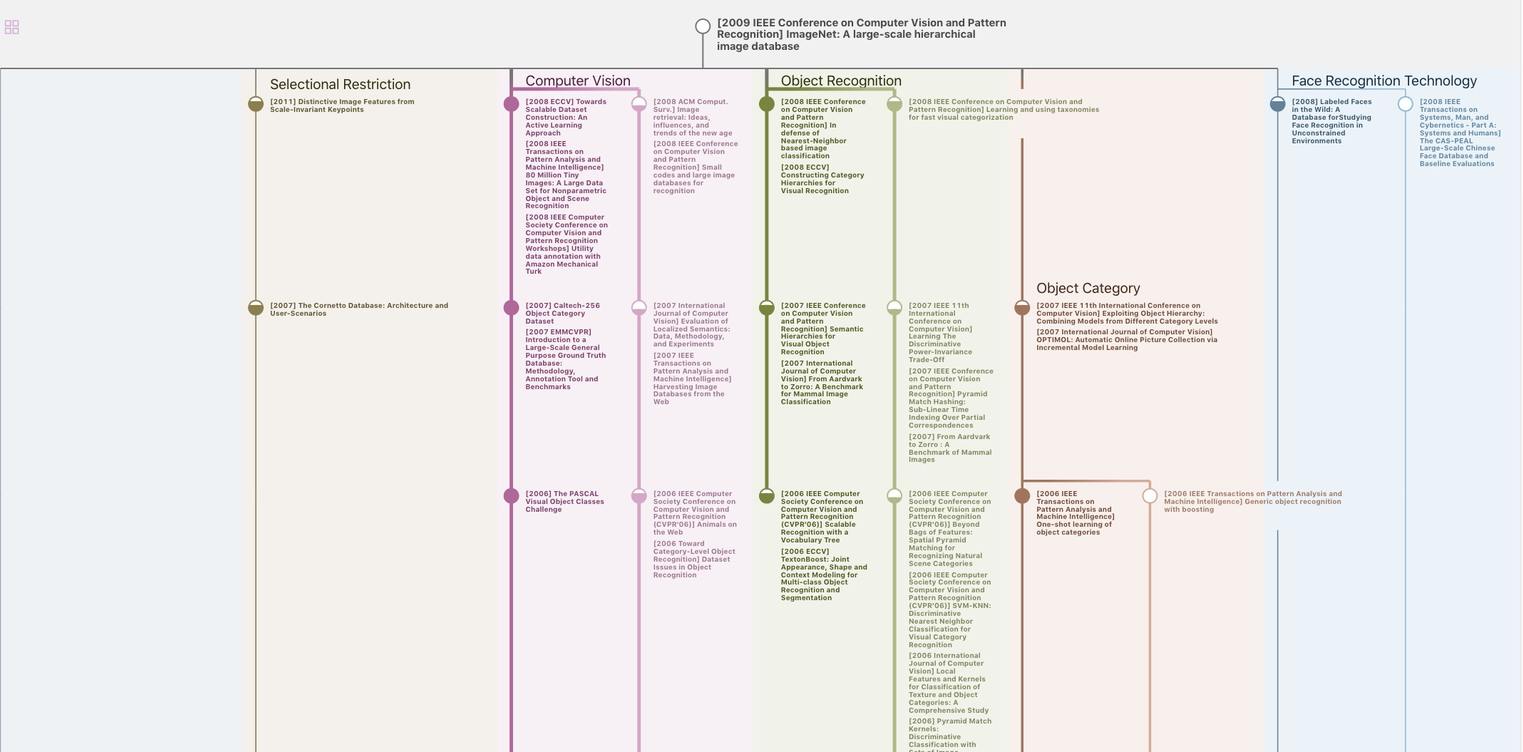
生成溯源树,研究论文发展脉络
Chat Paper
正在生成论文摘要