Action-GPT: Leveraging Large-scale Language Models for Improved and Generalized Action Generation
arxiv(2023)
摘要
We introduce Action-GPT, a plug-and-play framework for incorporating Large Language Models (LLMs) into text-based action generation models. Action phrases in current motion capture datasets contain minimal and to-the-point information. By carefully crafting prompts for LLMs, we generate richer and fine-grained descriptions of the action. We show that utilizing these detailed descriptions instead of the original action phrases leads to better alignment of text and motion spaces. We introduce a generic approach compatible with stochastic (e.g. VAE-based) and deterministic (e.g. MotionCLIP) text-to-motion models. In addition, the approach enables multiple text descriptions to be utilized. Our experiments show (i) noticeable qualitative and quantitative improvement in the quality of synthesized motions, (ii) benefits of utilizing multiple LLM-generated descriptions, (iii) suitability of the prompt function, and (iv) zero-shot generation capabilities of the proposed approach. Project page: https://actiongpt.github.io
更多查看译文
关键词
generalized action-gpt generation,language,large-scale
AI 理解论文
溯源树
样例
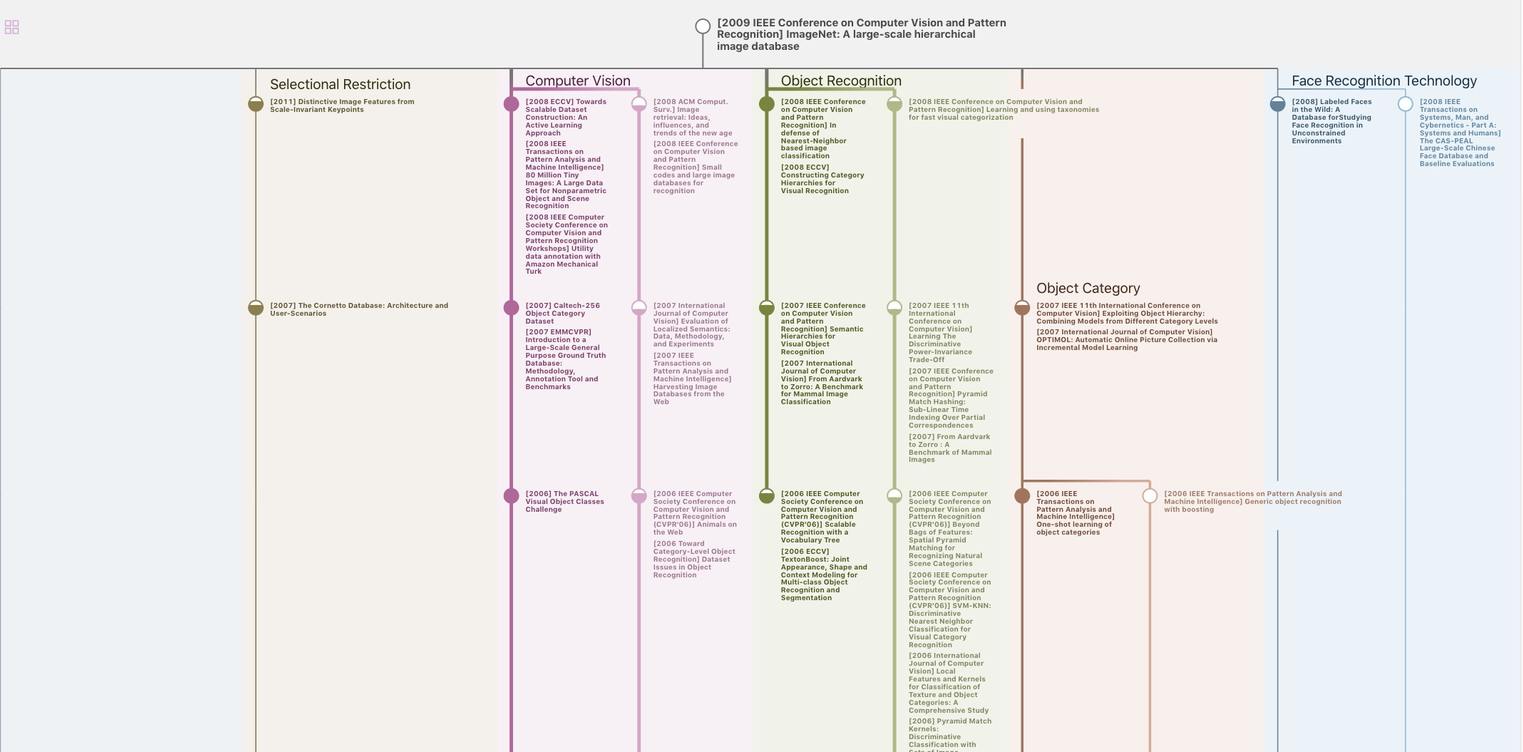
生成溯源树,研究论文发展脉络
Chat Paper
正在生成论文摘要