Malign Overfitting: Interpolation Can Provably Preclude Invariance
arxiv(2022)
摘要
Learned classifiers should often possess certain invariance properties meant to encourage fairness, robustness, or out-of-distribution generalization. However, multiple recent works empirically demonstrate that common invariance-inducing regularizers are ineffective in the over-parameterized regime, in which classifiers perfectly fit (i.e. interpolate) the training data. This suggests that the phenomenon of ``benign overfitting," in which models generalize well despite interpolating, might not favorably extend to settings in which robustness or fairness are desirable. In this work we provide a theoretical justification for these observations. We prove that -- even in the simplest of settings -- any interpolating learning rule (with arbitrarily small margin) will not satisfy these invariance properties. We then propose and analyze an algorithm that -- in the same setting -- successfully learns a non-interpolating classifier that is provably invariant. We validate our theoretical observations on simulated data and the Waterbirds dataset.
更多查看译文
关键词
interpolation,invariance
AI 理解论文
溯源树
样例
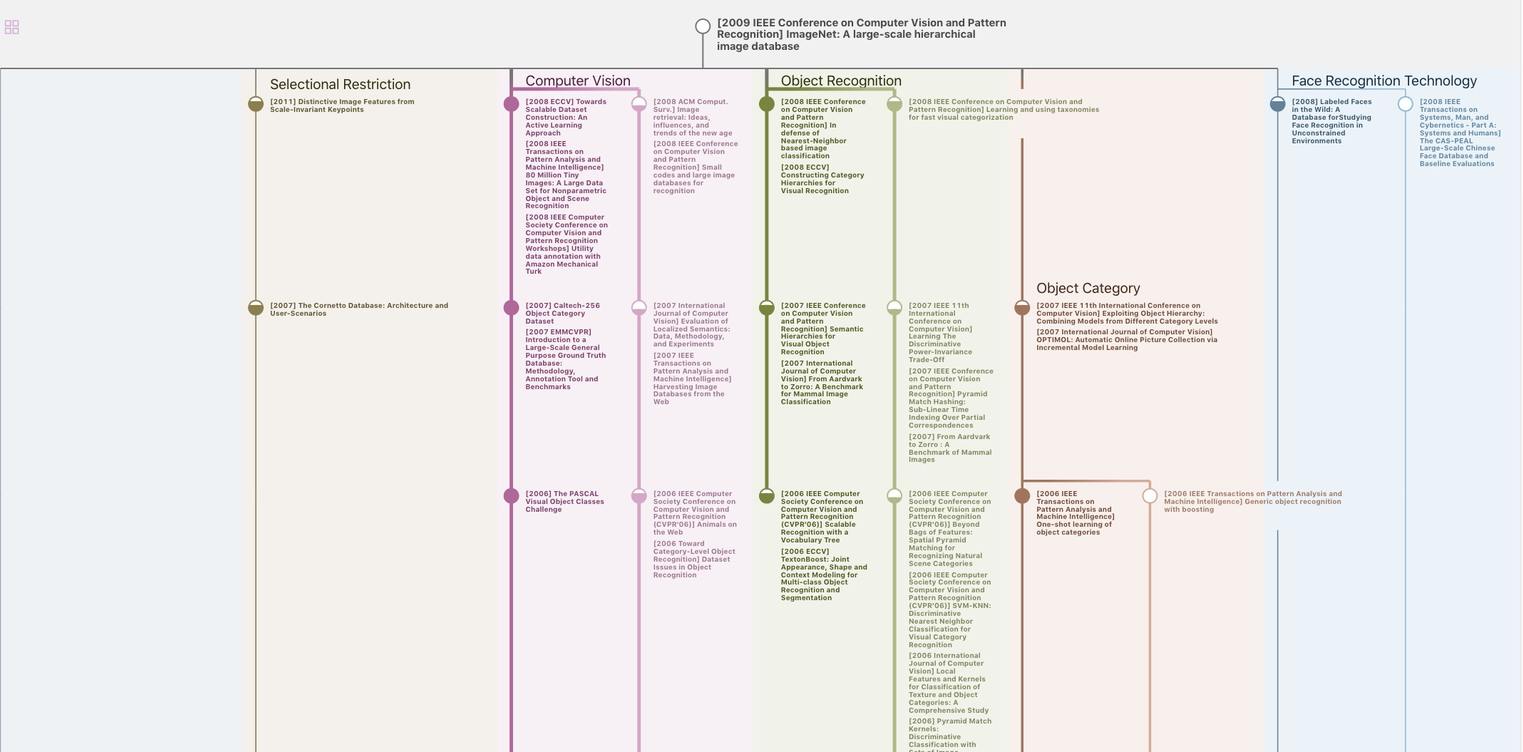
生成溯源树,研究论文发展脉络
Chat Paper
正在生成论文摘要