First order online optimisation using forward gradients in over-parameterised systems
arxiv(2022)
摘要
The success of deep learning over the past decade mainly relies on gradient-based optimisation and backpropagation. This paper focuses on analysing the performance of first-order gradient-based optimisation algorithms, gradient descent and proximal gradient, with time-varying non-convex cost function under (proximal) Polyak-{\L}ojasiewicz condition. Specifically, we focus on using the forward mode of automatic differentiation to compute gradients in the fast-changing problems where calculating gradients using the backpropagation algorithm is either impossible or inefficient. Upper bounds for tracking and asymptotic errors are derived for various cases, showing the linear convergence to a solution or a neighbourhood of an optimal solution, where the convergence rate decreases with the increase in the dimension of the problem. We show that for a solver with constraints on computing resources, the number of forward gradient iterations at each step can be a design parameter that trades off between the tracking performance and computing constraints.
更多查看译文
关键词
online optimisation,forward gradients,over-parameterised
AI 理解论文
溯源树
样例
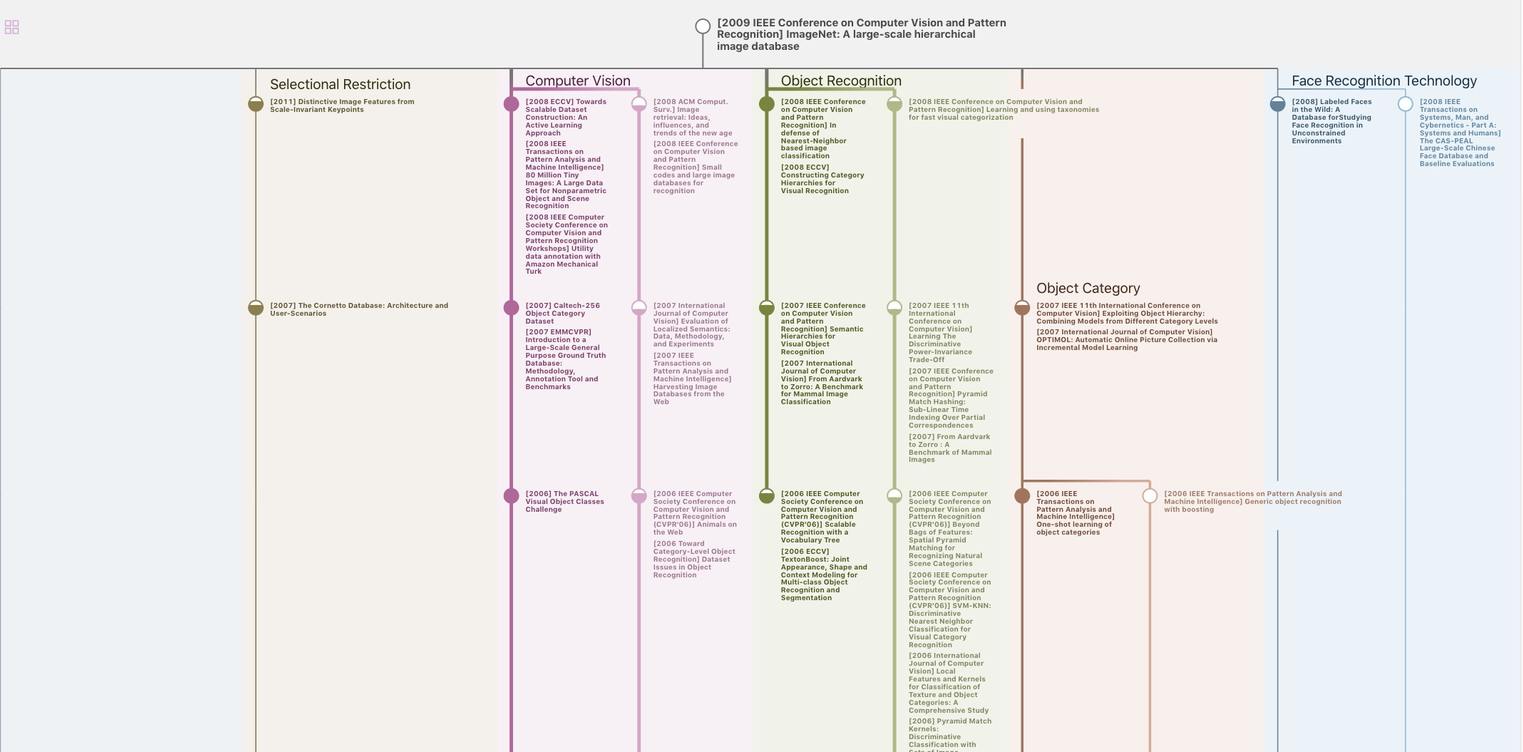
生成溯源树,研究论文发展脉络
Chat Paper
正在生成论文摘要