From Forks to Forceps: A New Framework for Instance Segmentation of Surgical Instruments
WACV(2023)
摘要
Minimally invasive surgeries and related applications demand surgical tool classification and segmentation at the instance level. Surgical tools are similar in appearance and are long, thin, and handled at an angle. The fine-tuning of state-of-the-art (SOTA) instance segmentation models trained on natural images for instrument segmentation has difficulty discriminating instrument classes. Our research demonstrates that while the bounding box and segmentation mask are often accurate, the classification head misclassifies the class label of the surgical instrument. We present a new neural network framework that adds a classification module as a new stage to existing instance segmentation models. This module specializes in improving the classification of instrument masks generated by the existing model. The module comprises multi-scale mask attention, which attends to the instrument region and masks the distracting background features. We propose training our classifier module using metric learning with arc loss to handle low inter-class variance of surgical instruments. We conduct exhaustive experiments on the benchmark datasets EndoVis2017 and EndoVis2018. We demonstrate that our method outperforms all (more than 18) SOTA methods compared with, and improves the SOTA performance by at least 12 points (20%) on the EndoVis2017 benchmark challenge and generalizes effectively across the datasets. Project page with source code is available at nets-iitd.github.io/s3net.
更多查看译文
关键词
instance segmentation,forks
AI 理解论文
溯源树
样例
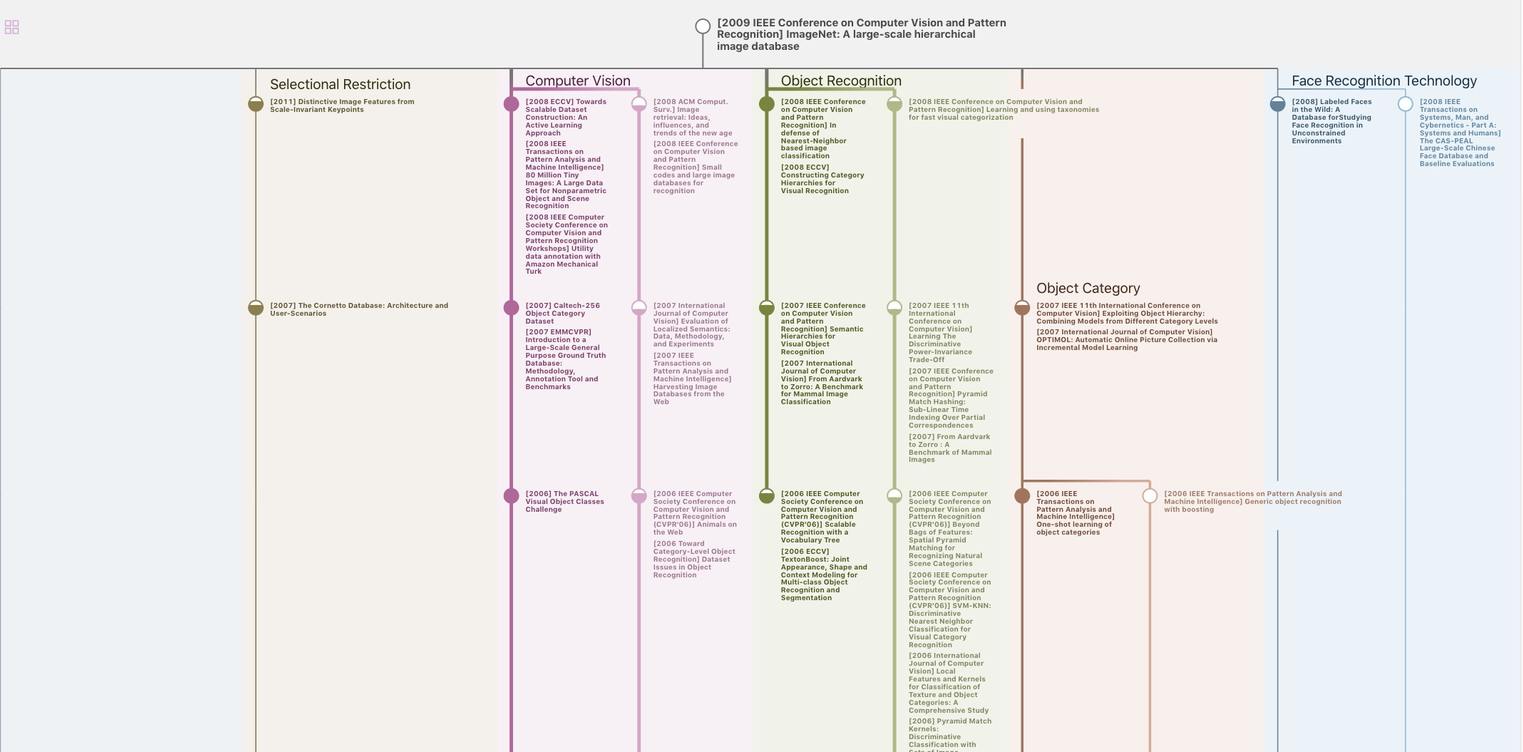
生成溯源树,研究论文发展脉络
Chat Paper
正在生成论文摘要