Ada3Diff: Defending against 3D Adversarial Point Clouds via Adaptive Diffusion
arxiv(2022)
摘要
Deep 3D point cloud models are sensitive to adversarial attacks, which poses threats to safety-critical applications such as autonomous driving. Robust training and defend-by-denoise are typical strategies for defending adversarial perturbations, including adversarial training and statistical filtering, respectively. However, they either induce massive computational overhead or rely heavily upon specified noise priors, limiting generalized robustness against attacks of all kinds. This paper introduces a new defense mechanism based on denoising diffusion models that can adaptively remove diverse noises with a tailored intensity estimator. Specifically, we first estimate adversarial distortions by calculating the distance of the points to their neighborhood best-fit plane. Depending on the distortion degree, we choose specific diffusion time steps for the input point cloud and perform the forward diffusion to disrupt potential adversarial shifts. Then we conduct the reverse denoising process to restore the disrupted point cloud back to a clean distribution. This approach enables effective defense against adaptive attacks with varying noise budgets, achieving accentuated robustness of existing 3D deep recognition models.
更多查看译文
关键词
ada3diff adversarial point clouds,diffusion
AI 理解论文
溯源树
样例
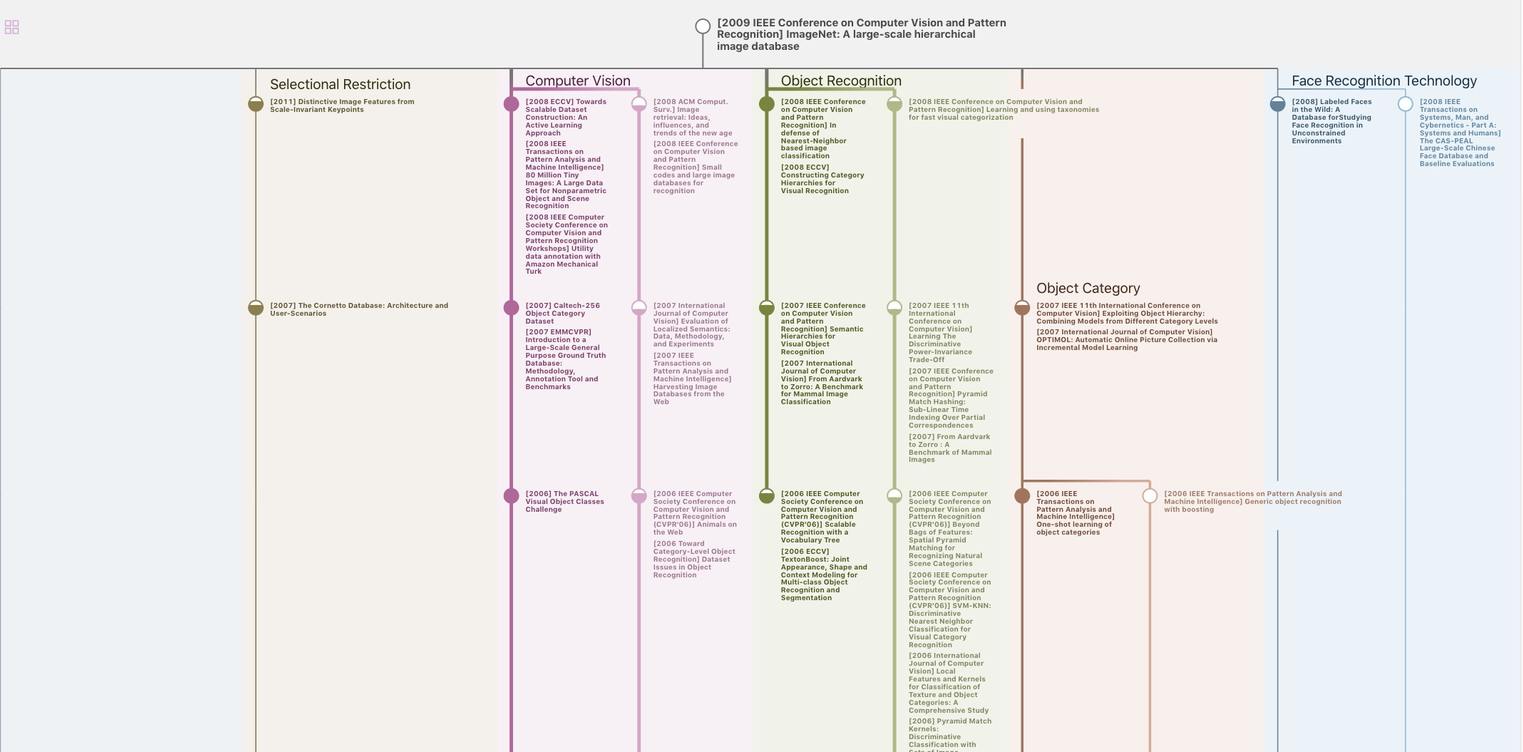
生成溯源树,研究论文发展脉络
Chat Paper
正在生成论文摘要