G-Net Light: A Lightweight Modified Google Net for Retinal Vessel Segmentation
Photonics(2022)
摘要
In recent years, convolutional neural network architectures have become increasingly complex to achieve improved performance on well-known benchmark datasets. In this research, we have introduced G-Net light, a lightweight modified GoogleNet with improved filter count per layer to reduce feature overlaps, hence reducing the complexity. Additionally, by limiting the amount of pooling layers in the proposed architecture, we have exploited the skip connections to minimize the spatial information loss. The suggested architecture is analysed using three publicly available datasets for retinal vessel segmentation, namely DRIVE, CHASE and STARE datasets. The proposed G-Net light achieves an average accuracy of 0.9686, 0.9726, 0.9730 and F1-score of 0.8202, 0.8048, 0.8178 on DRIVE, CHASE, and STARE datasets, respectively. The proposed G-Net light achieves state-of-the-art performance and outperforms other lightweight vessel segmentation architectures with fewer trainable number of parameters.
更多查看译文
关键词
deep learning,convolutional neural networks,medical image segmentation
AI 理解论文
溯源树
样例
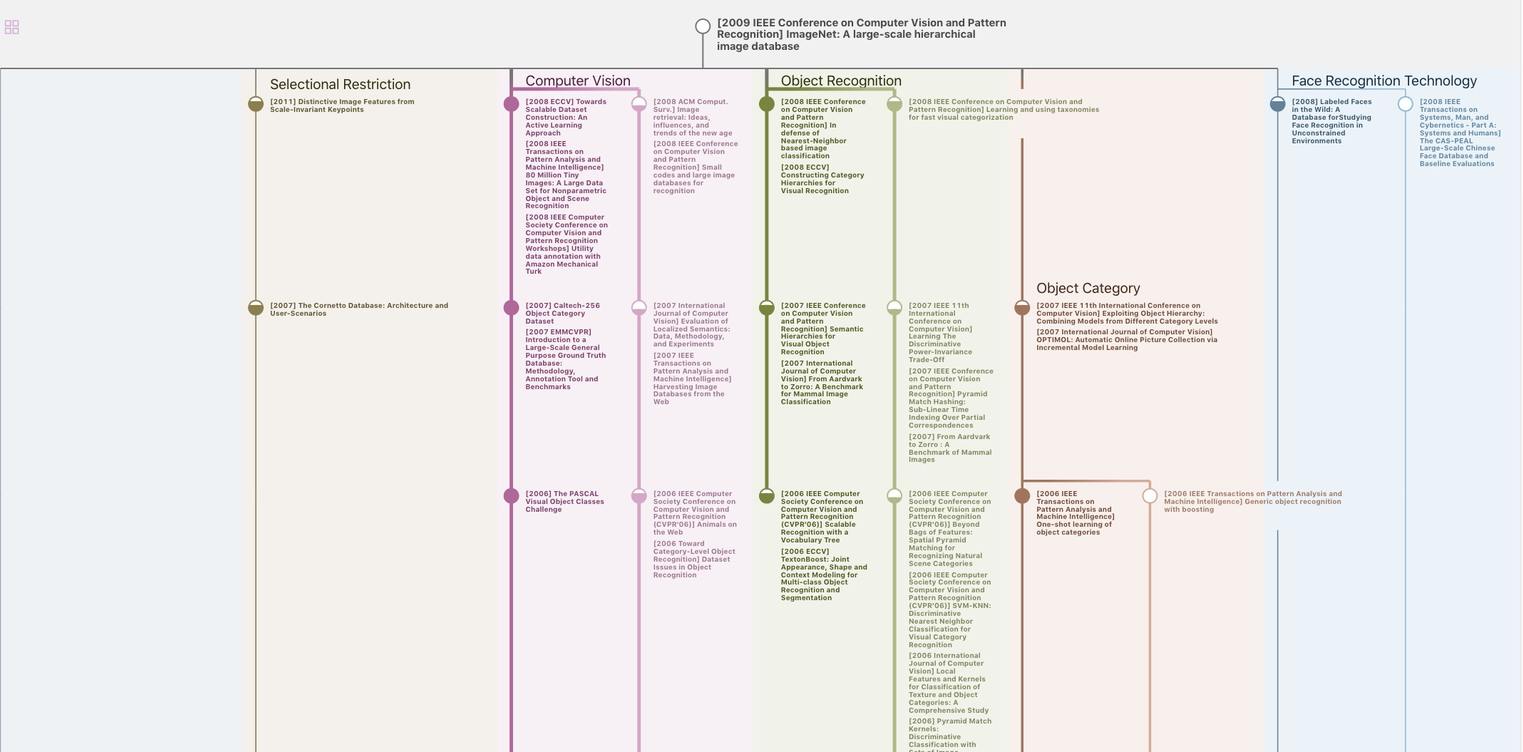
生成溯源树,研究论文发展脉络
Chat Paper
正在生成论文摘要