Towards Dynamic Causal Discovery with Rare Events: A Nonparametric Conditional Independence Test
arxiv(2023)
摘要
Causal phenomena associated with rare events occur across a wide range of engineering problems, such as risk-sensitive safety analysis, accident analysis and prevention, and extreme value theory. However, current methods for causal discovery are often unable to uncover causal links, between random variables in a dynamic setting, that manifest only when the variables first experience low-probability realizations. To address this issue, we introduce a novel statistical independence test on data collected from time-invariant dynamical systems in which rare but consequential events occur. In particular, we exploit the time-invariance of the underlying data to construct a superimposed dataset of the system state before rare events happen at different timesteps. We then design a conditional independence test on the reorganized data. We provide non-asymptotic sample complexity bounds for the consistency of our method, and validate its performance across various simulated and real-world datasets, including incident data collected from the Caltrans Performance Measurement System (PeMS). Code containing the datasets and experiments is publicly available.
更多查看译文
关键词
dynamic causal discovery,rare events,conditional independence test
AI 理解论文
溯源树
样例
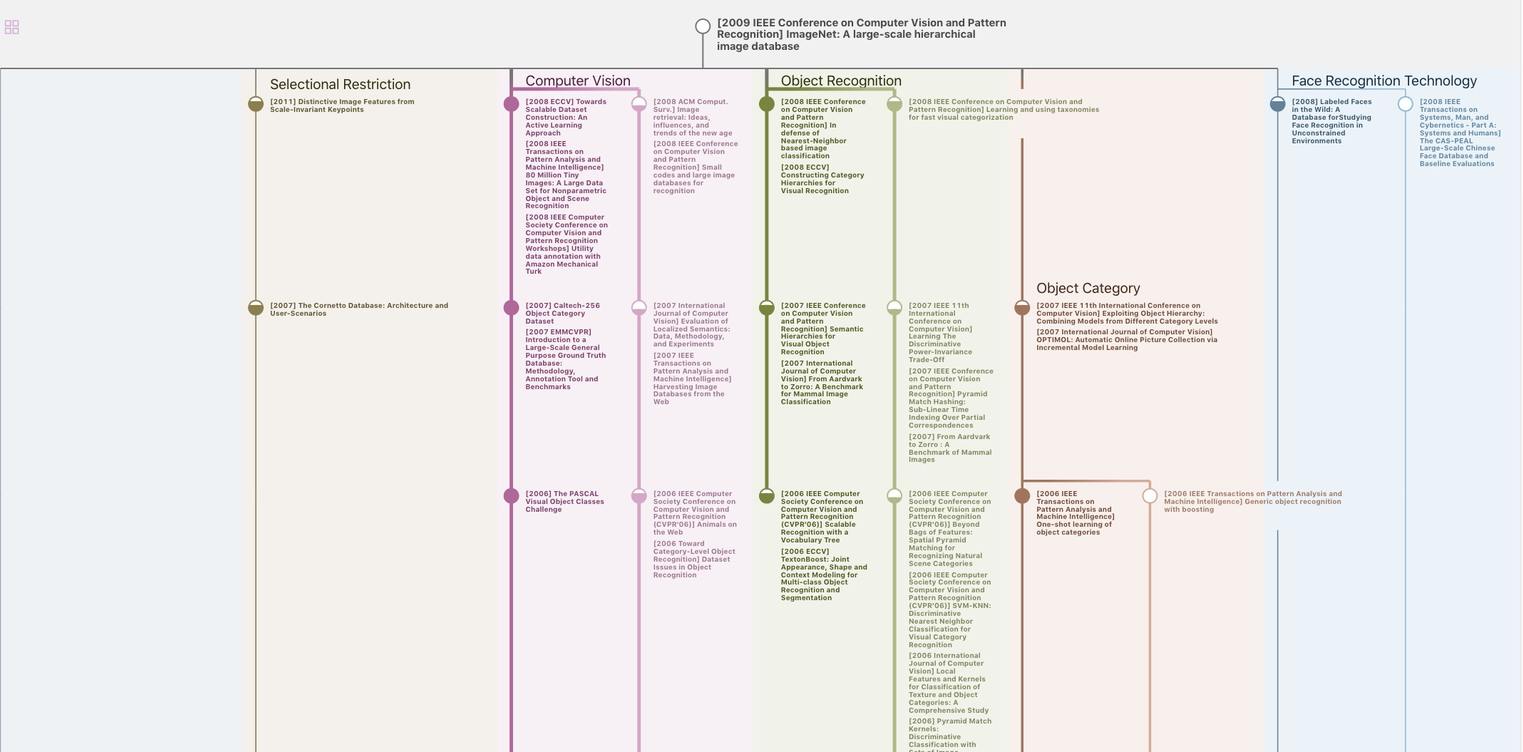
生成溯源树,研究论文发展脉络
Chat Paper
正在生成论文摘要