Distributed Averaging in Population Protocols
arxiv(2023)
摘要
We consider two simple asynchronous opinion dynamics on arbitrary graphs where each node $u$ of the graph has an initial value $\xi_u(0)$. In the first process, the $NodeModel$, at each time step $t\ge 0$, a random node $u$ and a random sample of $k$ of its neighbours $v_1,v_2,\cdots,v_k$ are selected. Then $u$ updates its current value $\xi_u(t)$ to $\xi_u(t+1)=\alpha\xi_u(t)+\frac{(1-\alpha)}{k}\sum_{i=1}^k\xi_{v_i}(t)$, where $\alpha\in(0,1)$ and $k\ge1$ are parameters of the process. In the second process, the $EdgeModel$, at each step a random edge $(u,v)$ is selected. Node $u$ updates its value equivalently to the $NodeModel$ with $k=1$ and $v$ as the selected neighbour. For both processes the values of all nodes converge to the same value $F$, which is a random variable depending on the random choices made in each step. For the $NodeModel$ and regular graphs, and for the $EdgeModel$ and arbitrary graphs, the expectation of $F$ is the average of the initial values $\frac{1}{n}\sum_{u\in V}\xi_u(0)$. For the $NodeModel$ and non-regular graphs, the expectation of $F$ is the degree-weighted average of the initial values. Our results are two-fold. We consider the concentration of $F$ and show tight bounds on the variance of $F$ for regular graphs. We show that when the initial load does not depend on the number of nodes, the variance is negligible and the nodes are able to estimate the initial average of the node values. Interestingly, this variance does not depend on the graph structure. For the proof we introduce a duality between our processes and a process of two correlated random walks. We also analyse the convergence time for both models and for arbitrary graphs, showing bounds on the time $T_\varepsilon$ needed to make all node values `$\varepsilon$-close' to each other. Our bounds are asymptotically tight under some assumptions on the distribution of the starting values.
更多查看译文
AI 理解论文
溯源树
样例
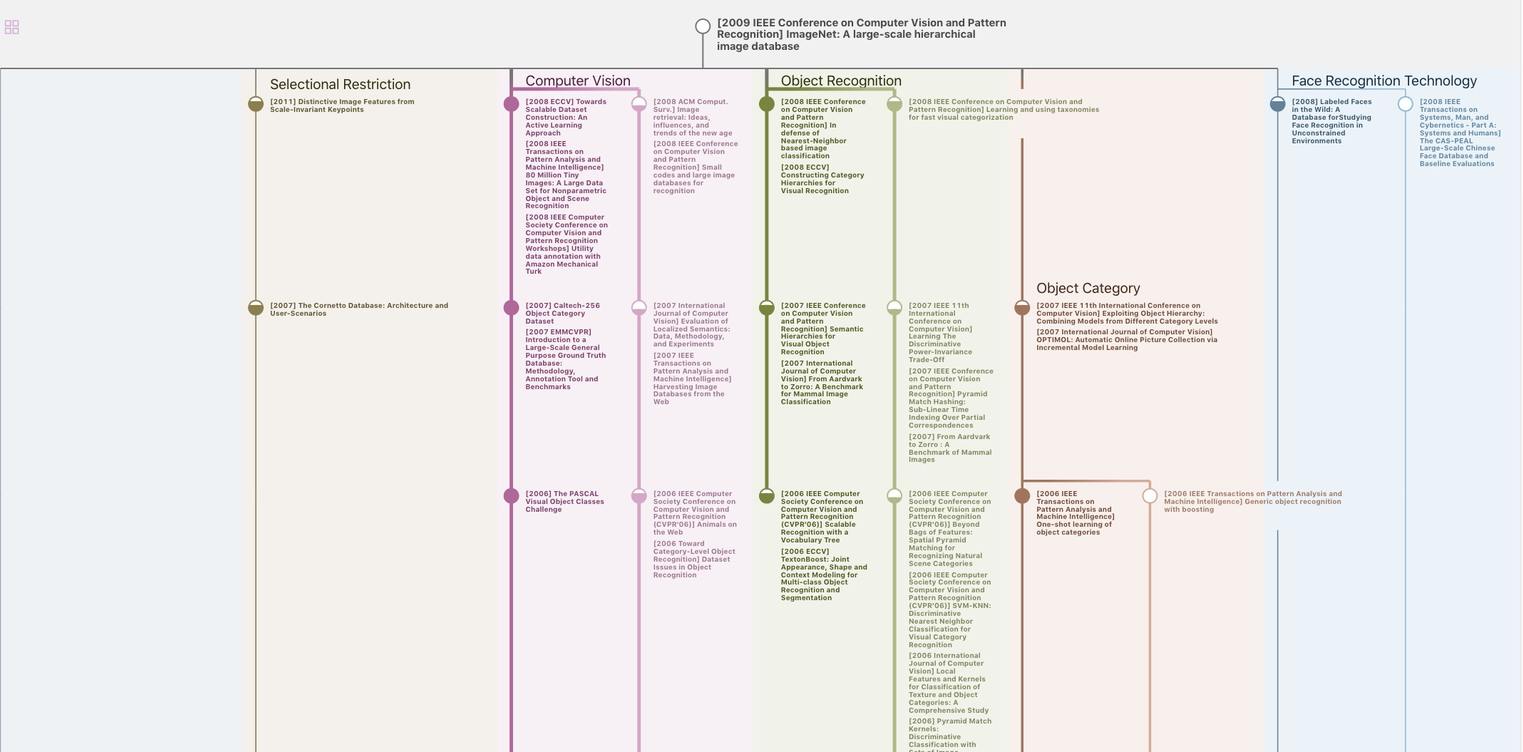
生成溯源树,研究论文发展脉络
Chat Paper
正在生成论文摘要