Optimizing Explanations by Network Canonization and Hyperparameter Search
arxiv(2023)
摘要
Explainable AI (XAI) is slowly becoming a key component for many AI applications. Rule-based and modified backpropagation XAI approaches however often face challenges when being applied to modern model architectures including innovative layer building blocks, which is caused by two reasons. Firstly, the high flexibility of rule-based XAI methods leads to numerous potential parameterizations. Secondly, many XAI methods break the implementation-invariance axiom because they struggle with certain model components, e.g., BatchNorm layers. The latter can be addressed with model canonization, which is the process of re-structuring the model to disregard problematic components without changing the underlying function. While model canonization is straightforward for simple architectures (e.g., VGG, ResNet), it can be challenging for more complex and highly interconnected models (e.g., DenseNet). Moreover, there is only little quantifiable evidence that model canonization is beneficial for XAI. In this work, we propose canonizations for currently relevant model blocks applicable to popular deep neural network architectures,including VGG, ResNet, EfficientNet, DenseNets, as well as Relation Networks. We further suggest a XAI evaluation framework with which we quantify and compare the effect sof model canonization for various XAI methods in image classification tasks on the Pascal-VOC and ILSVRC2017 datasets, as well as for Visual Question Answering using CLEVR-XAI. Moreover, addressing the former issue outlined above, we demonstrate how our evaluation framework can be applied to perform hyperparameter search for XAI methods to optimize the quality of explanations.
更多查看译文
关键词
CLEVR-XAI,complex models,currently relevant model blocks,highly interconnected models,hyperparameter search,innovative layer building blocks,model canonization,modern model architectures,network canonization,popular deep neural network architectures,rule-based XAI methods,XAI approaches,XAI evaluation framework
AI 理解论文
溯源树
样例
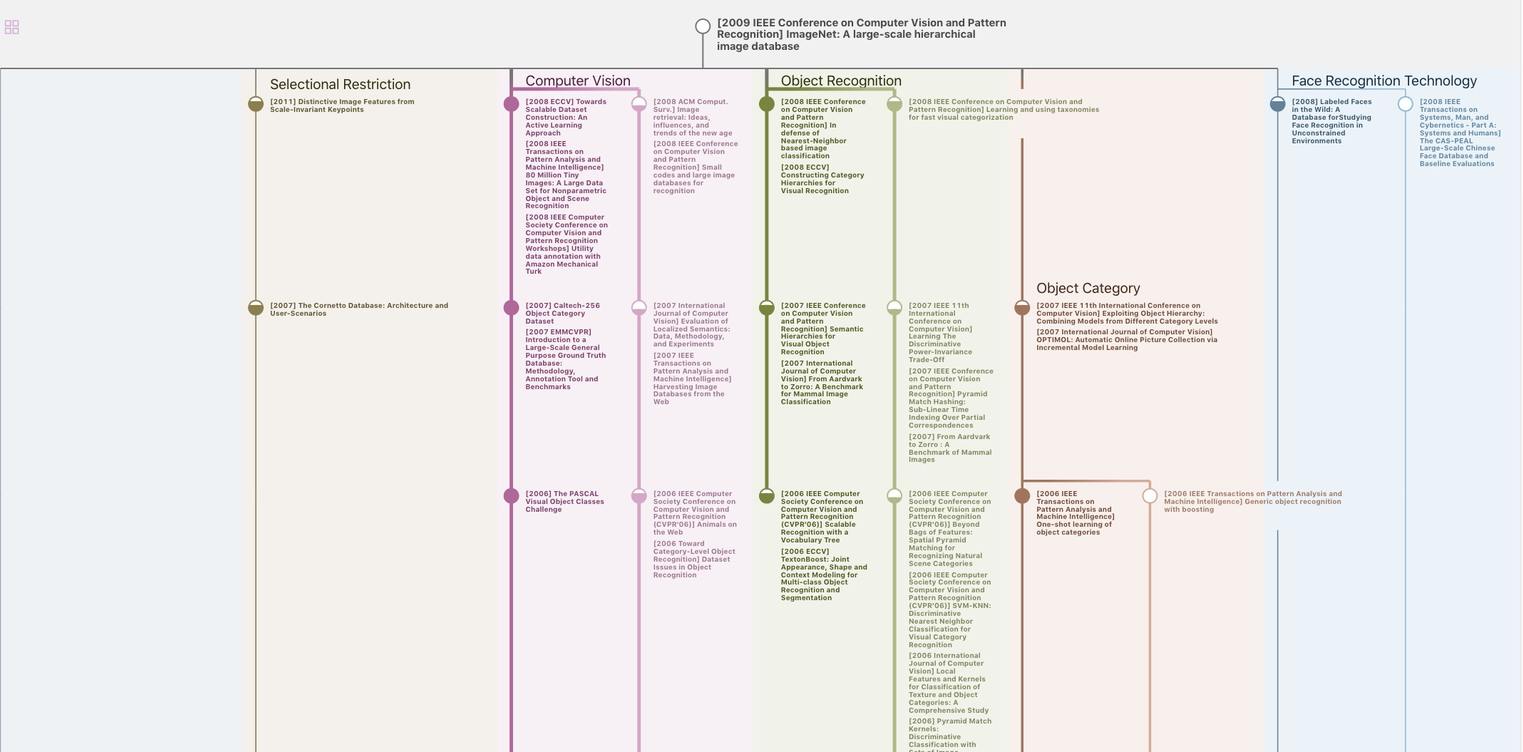
生成溯源树,研究论文发展脉络
Chat Paper
正在生成论文摘要