Data and knowledge co-driving for cancer subtype classification on multi-scale histopathological slides
Knowledge-Based Systems(2022)
摘要
Artificial intelligence-enabled histopathological data analysis has become a valuable assistant to the pathologist. However, existing models lack representation and inference abilities compared with those of pathologists, especially in cancer subtype diagnosis, which is unconvincing in clinical practice. For instance, pathologists typically observe the lesions of a slide from global to local, and then can give a diagnosis based on their knowledge and experience. In this paper, we propose a Data and Knowledge Co-driving (D&K) model to replicate the process of cancer subtype classification on a histopathological slide like a pathologist. Specifically, in the data-driven module, the bagging mechanism in ensemble learning is leveraged to integrate the histological features from various bags extracted by the embedding representation unit. Furthermore, a knowledge-driven module is established based on the Gestalt principle in psychology to build the three-dimensional (3D) expert knowledge space and map histological features into this space for metric. Then, the diagnosis can be made according to the Euclidean distance between them. Extensive experimental results on both public and in-house datasets demonstrate that the D&K model has a high performance and credible results compared with the state-of-the-art methods for diagnosing histopathological subtypes. Code: https://github.com/Dennis-YB/Data-and-Knowledge-Co-driving-for-Cancer-Subtypes-Classification
更多查看译文
关键词
Subtype classification,Histopathological data,Interpretable diagnosis,Multi-scale,Knowledge-driven
AI 理解论文
溯源树
样例
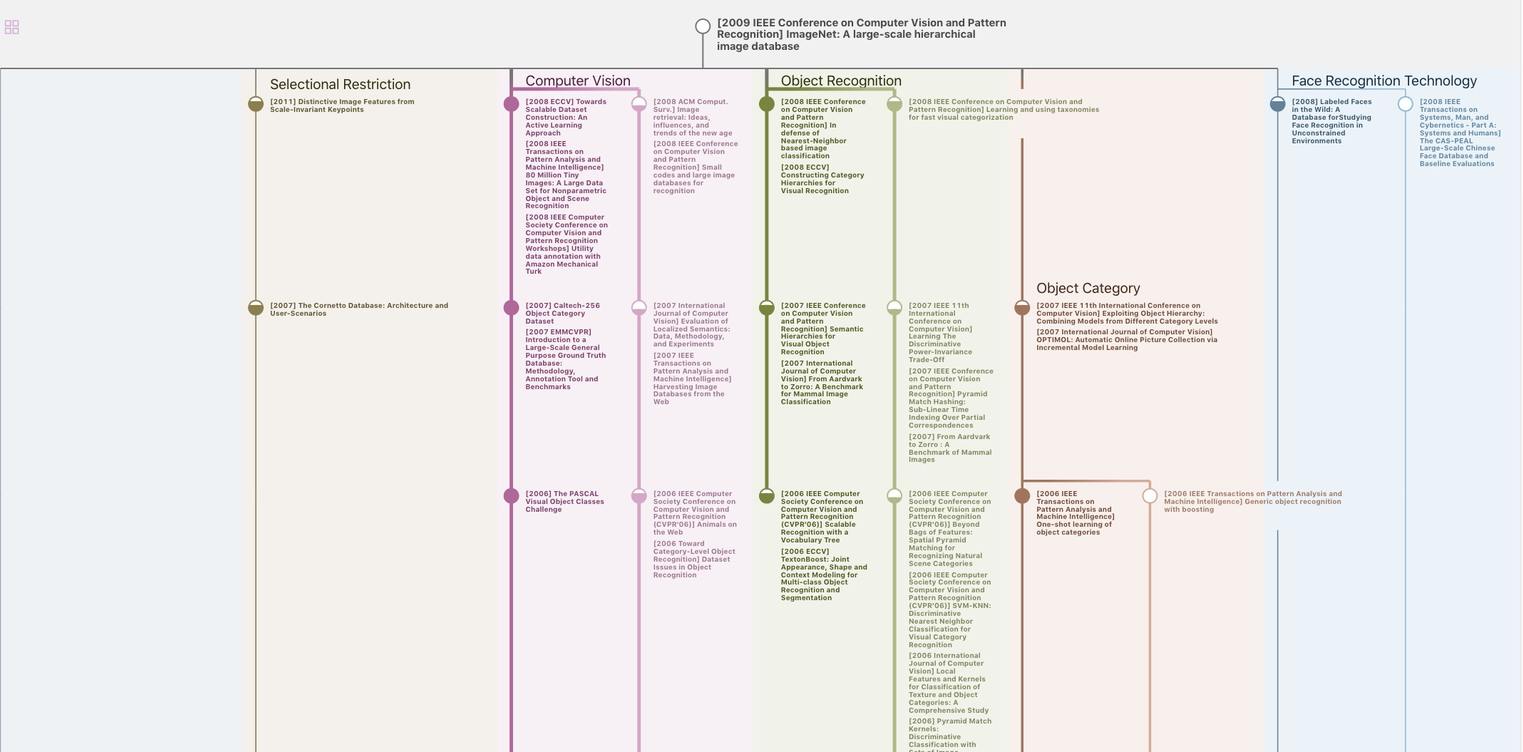
生成溯源树,研究论文发展脉络
Chat Paper
正在生成论文摘要