Deep Neural Network-based Black-box Modeling of Power Electronic Converters Using Transfer Learning
2022 IEEE Energy Conversion Congress and Exposition (ECCE)(2022)
摘要
Black-box modeling of power electronic converters (PECs) is an essential tool for studying commercial converters. Neural-networks-based modeling techniques are data-driven based solely on signal measurements. Recent studies show that Long-Short Term Memory (LSTM) and Gated Recurrent Unit (GRU) structures used in deep neural network (DNN) black-box modeling methods are capable of modeling steady-state and transient behavior of PECs. Training times for such structures can be extremely long. This paper uses a technique called “transfer-learning” to improve the training times of DNN-based black-box modeling techniques based on LSTM and GRU. The models are trained using different data sizes to study the effects of transfer learning thoroughly. The detailed switching models of two synchronous DC/DC buck converters” as well as two HPUC converters” are simulated in MATLAB/Simulink environment for gathering training and test data. The obtained results demonstrate the transfer-learning method's superiority over conventional techniques in terms of improved training times.
更多查看译文
关键词
power electronic converters,network-based,black-box
AI 理解论文
溯源树
样例
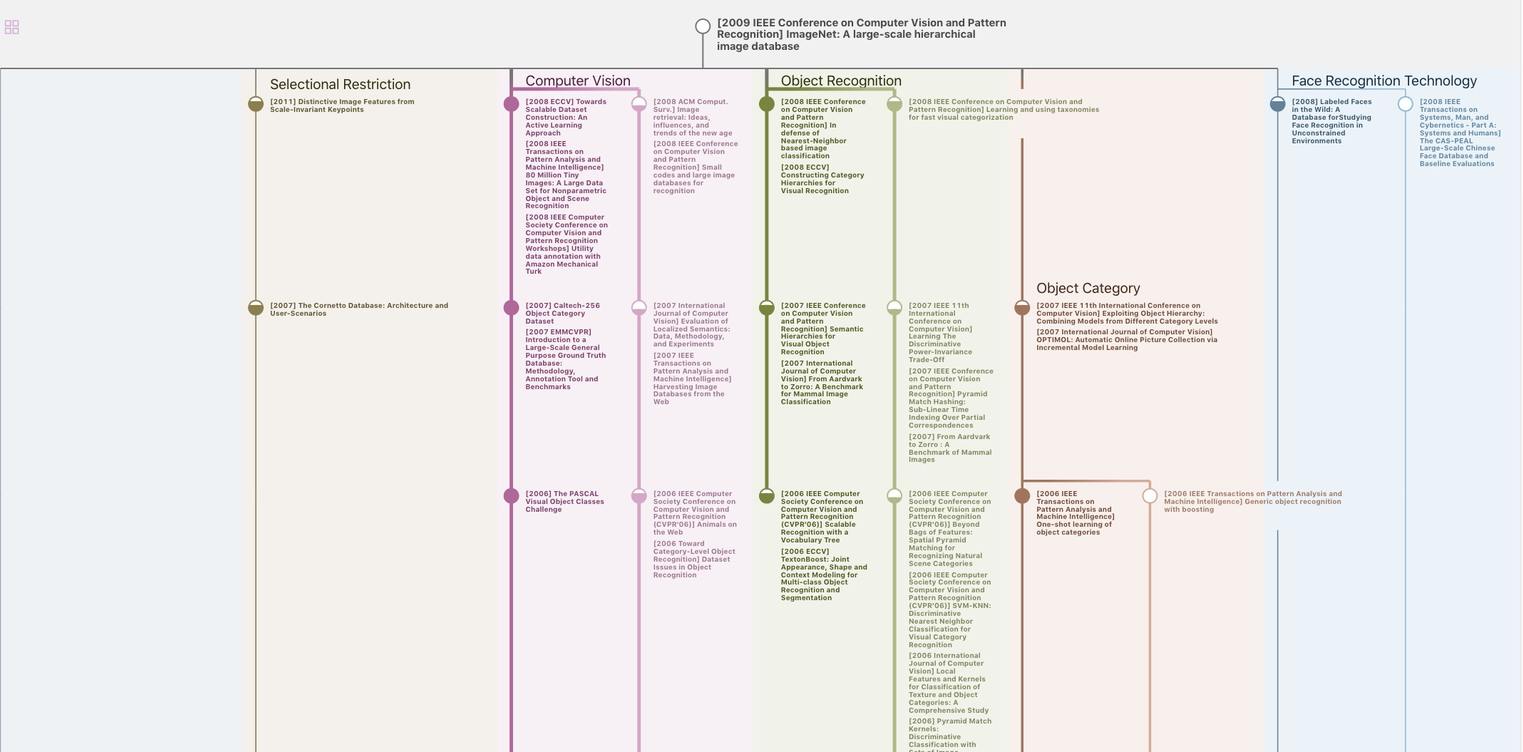
生成溯源树,研究论文发展脉络
Chat Paper
正在生成论文摘要