Cross-platform sequential recommendation with sharing item-level relevance data
Information Sciences(2022)
摘要
Cross-platform sequential recommendations are a typical solution to the problem of sparse data and cold starts in the field of recommendation systems. Specifically, we utilize data from the auxiliary platform to improve the recommendation performance of the target platform. One typical scenario is the fusion of data from two interaction platforms to perform cross-platform recommendation tasks. Existing approaches assume that interaction data from the auxiliary platform can fully share cross-platforms. However, such a hypothesis is unreasonable as, in the real world, different companies may operate these platforms. Records of user interactions with items are sensitive, and the complete sharing of original data could violate business privacy policies and increase the risk of privacy leaks. This paper considers a more realistic scenario for performing cross-platform sequential recommendations. To avoid compromising users’ privacy during data sharing, we contemplate sharing only item-level relevance data and not user-level relevance data. Concretely, we transfer item embedding cross-platform to make it easier for both companies to agree on data sharing (e.g., legal policies), as the data to share is irrelevant to the user and has no explicit semantics. For extracting a valuable signal from the transfer items embedding, we propose a new model, Neural Attention Transfer Sequential Recommendation (abbreviated as NATSR), by exploiting the powerful representation capabilities of neural networks. We perform thorough experiments with two real datasets to verify their performance. The NATSR model achieves the best recommendation performance compared to the traditional cross-platform approach of directly sharing user-level relevance data. We demonstrate further that the NATSR model dramatically mitigates the problem of data sparsity with significant user privacy-preserving.
更多查看译文
关键词
Cross-platform sequential recommendation,Privacy-preserving,Attention mechanism,Transfer learning,Recommendation system
AI 理解论文
溯源树
样例
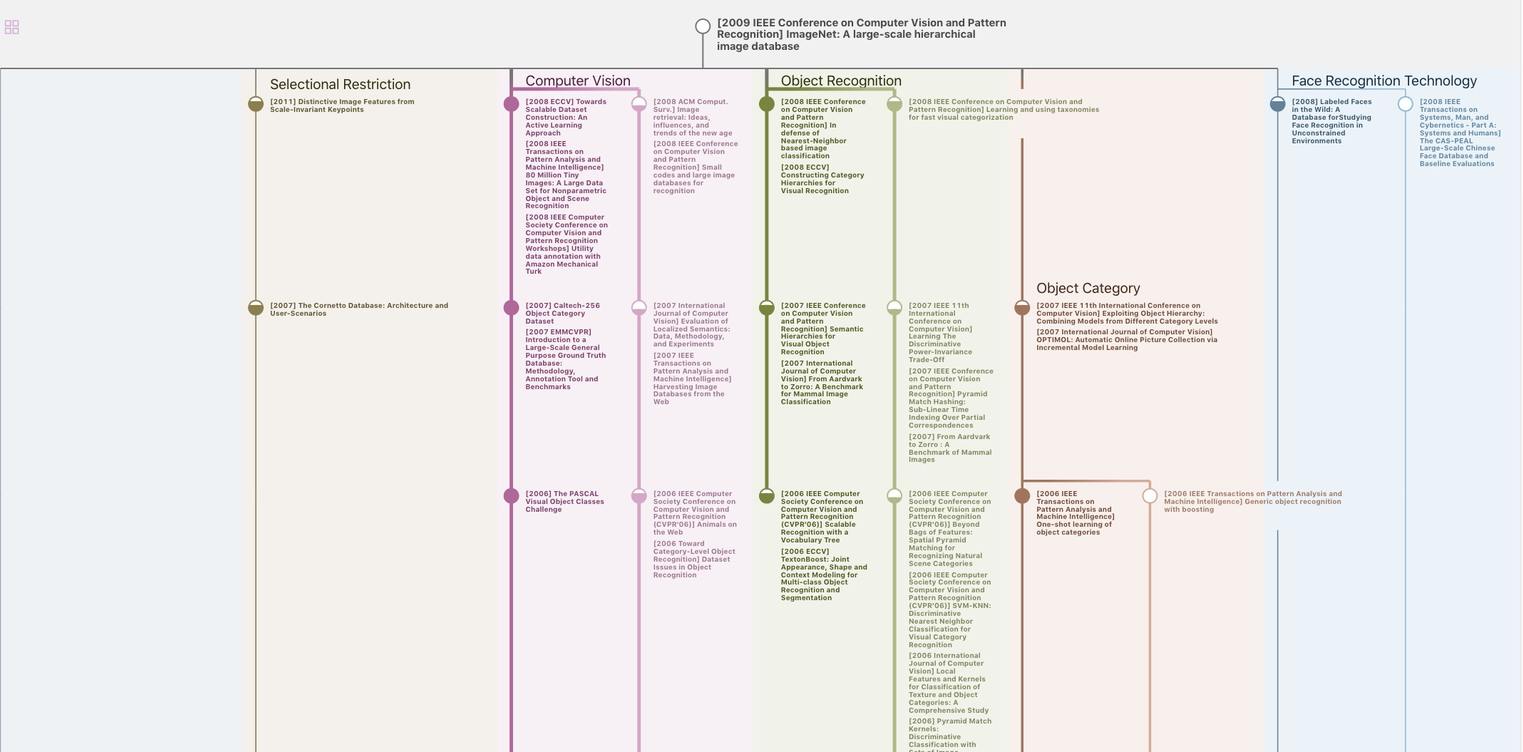
生成溯源树,研究论文发展脉络
Chat Paper
正在生成论文摘要