Efficient Underwater Docking Detection using Knowledge Distillation and Artificial Image Generation
2022 IEEE/OES Autonomous Underwater Vehicles Symposium (AUV)(2022)
摘要
Underwater docking is a staged process in which the detection of the dock is crucial. It allows Autonomous Underwater Vehicles (AUVs) to recharge and transfer data, enabling long-term missions; recent work shows that deep learning can be used to robustly perform docking detection at the expense of a large amount of resources for deployment on embedded devices. This paper proposes a method to efficiently train a Convolutional Neural Network (CNN) to detect a docking station using knowledge distillation under the teacher-student architecture. Additionally, to augment the amount of data available for training, we use two methods to generate synthetic datasets, one utilizing a CycleGAN network and another using an Artistic Style transfer network. Furthermore, we show the benefit of using synthetic data during the training of the CNNs and compare the performance of the teacher and the student networks on actual underwater data.
更多查看译文
关键词
Underwater docking,AUVs,machine learning,knowledge distillation,marine robotics,Convolutional Neural Networks
AI 理解论文
溯源树
样例
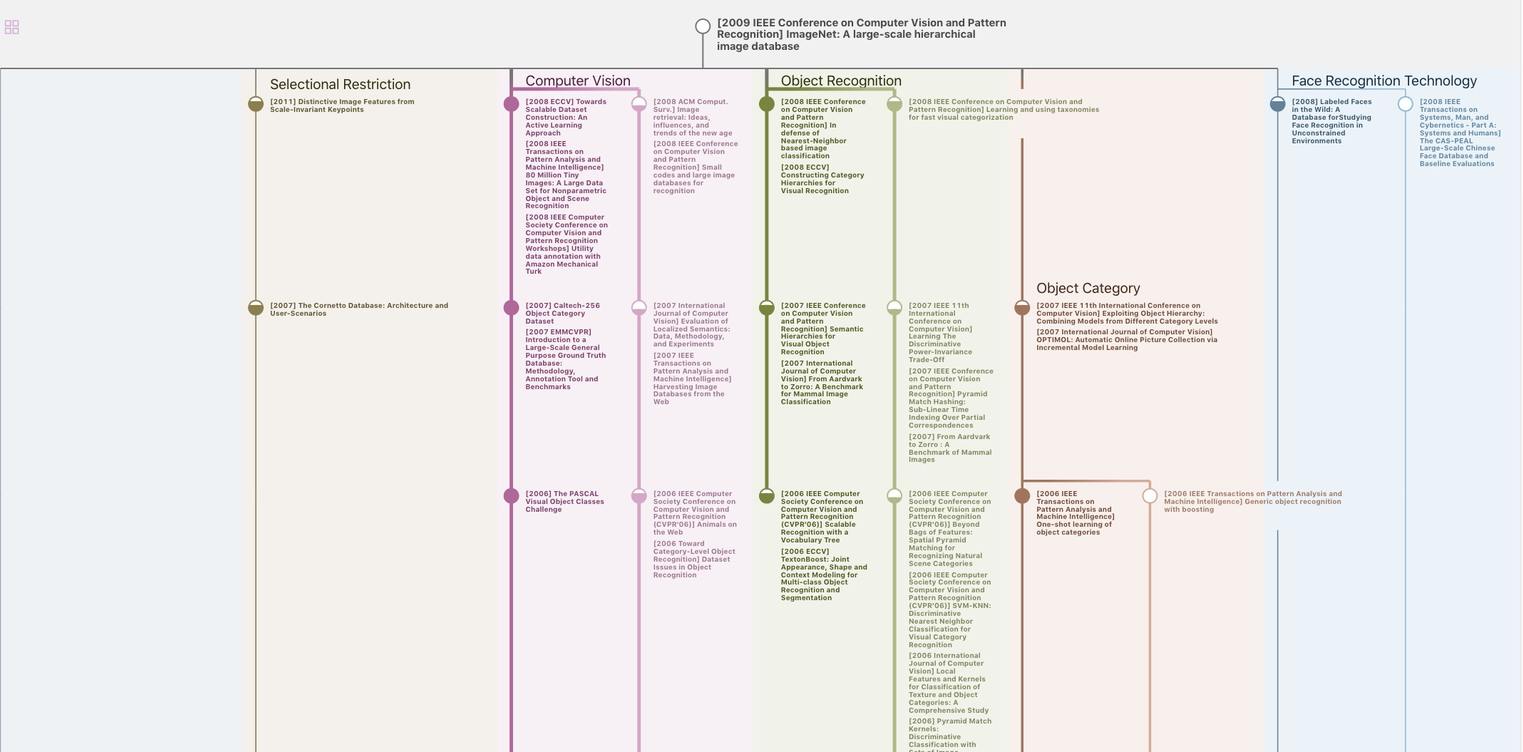
生成溯源树,研究论文发展脉络
Chat Paper
正在生成论文摘要