Mountain Snow Depth Retrieval from Optical and Passive Microwave Remote Sensing using Machine Learning
IEEE Geoscience and Remote Sensing Letters(2022)
摘要
Snow depth (SD) or snow water equivalent in mountainous regions is crucial for hydrology, water resources management, meteorological, and climate research. Remote sensing can be used for SD monitoring on a regional scale or global scale. However, the spaceborne remote sensing of SD in the mountain is challenging because of the sensor sensitivity and spatial resolution problems. Recently, the time series Sentinel-1 is used for SD retrieval in mountains, which shows encouraging accuracy. In this study, an algorithm to estimate the SD in mountainous regions using optical and passive microwave remote sensing observations is proposed, which can be applied in periods before and after the launch of Sentinel-1. The optical and passive microwave remote sensing observations and the Sentinel-1 derived SD in 2016-2021 are used to train the SD retrieval algorithm using the Extreme gradient boosting (XGBoost) machine learning algorithm. Validations are performed using the cross-validation method and independent in situ SD data. The cross-validation shows a correlation coefficient of 0.81 and a mean absolute error (MAE) of 0.17 m. The correlation coefficient and MAE of predicted SD and in situ SD in 2002-2016 are 0.61 and 0.33 m, respectively, which shows significantly higher accuracy compared with AMSR-E/AMSR2 SD products. The site dependence of the machine learning method is also discussed. The machine learning-based SD retrieval presented in this study can be applied to mountains globally in the optical and passive microwave remote sensing era.
更多查看译文
关键词
Machine learning,passive microwave,SAR,snow depth (SD)
AI 理解论文
溯源树
样例
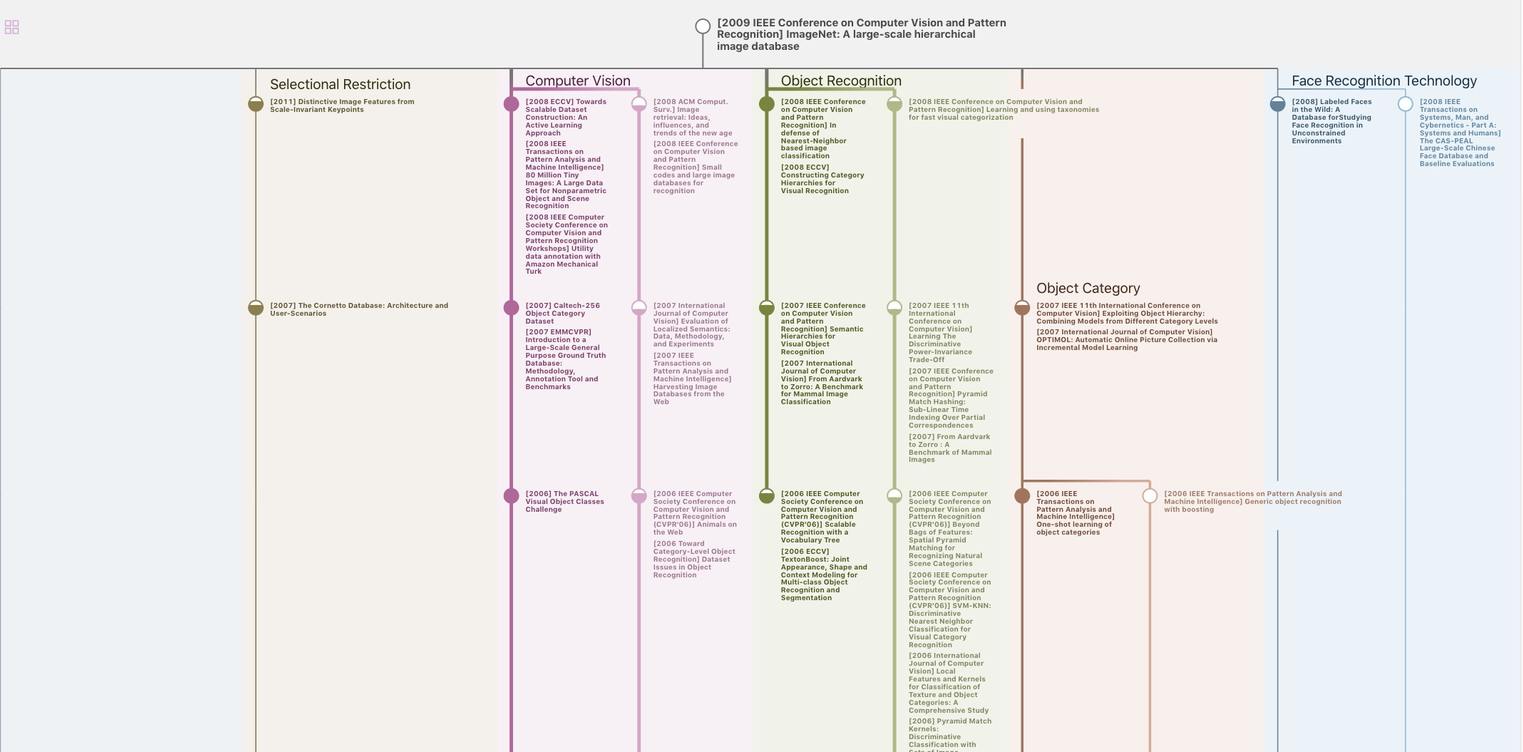
生成溯源树,研究论文发展脉络
Chat Paper
正在生成论文摘要