Machine Learning Models for Customer Churn Risk Prediction
2022 IEEE 13th Annual Ubiquitous Computing, Electronics & Mobile Communication Conference (UEMCON)(2022)
摘要
Customer churn analysis in the industry is an important area of research due to its effect on profitability of business, measuring customer satisfaction, figuring out product promotions, and creating marketing strategies. In this paper we predict the possibility of churn of a given customer by advanced machine learning pipelines. In particular we perform a comprehensive comparison of feature selection methods, oversampling methods, and three machine learning methods the Logistic Regression, Random Forest and Deep Neural Networks on customer churn dataset. The conclusions we reach include (1) Information Value features performed significantly better than Principal Component Analysis features, (2) data oversampling provides model consistency, and (3) Random Forest is superior to Logistic Regression and Deep Neural Networks in the datasets where the number of instances is few.
更多查看译文
关键词
deep neural networks,random forest,logistic regression,smote,adasyn,information value analysis
AI 理解论文
溯源树
样例
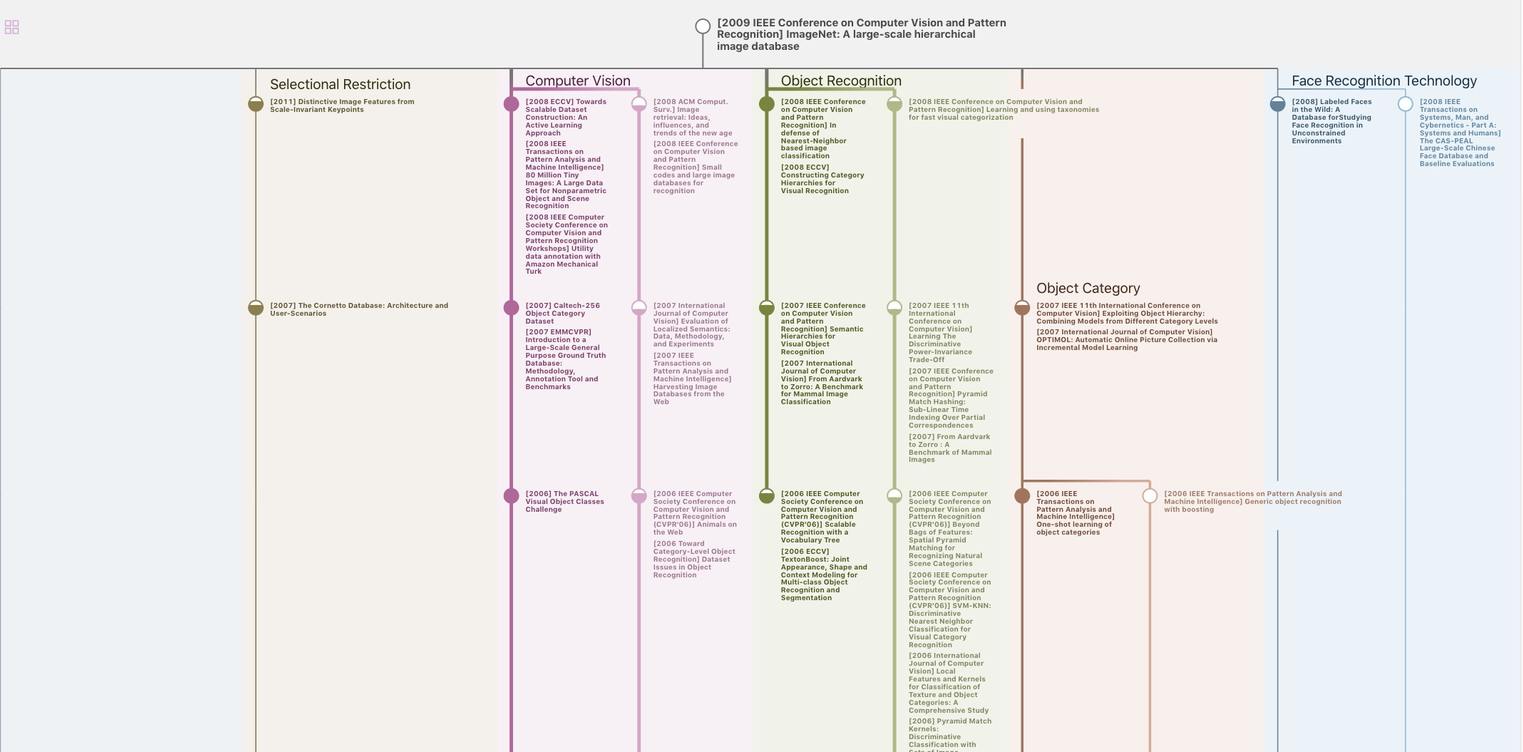
生成溯源树,研究论文发展脉络
Chat Paper
正在生成论文摘要