The Effect of Data Dimensionality on Neural Network Prunability
CoRR(2022)
摘要
Practitioners prune neural networks for efficiency gains and generalization improvements, but few scrutinize the factors determining the prunability of a neural network the maximum fraction of weights that pruning can remove without compromising the model's test accuracy. In this work, we study the properties of input data that may contribute to the prunability of a neural network. For high dimensional input data such as images, text, and audio, the manifold hypothesis suggests that these high dimensional inputs approximately lie on or near a significantly lower dimensional manifold. Prior work demonstrates that the underlying low dimensional structure of the input data may affect the sample efficiency of learning. In this paper, we investigate whether the low dimensional structure of the input data affects the prunability of a neural network.
更多查看译文
关键词
data dimensionality,neural network
AI 理解论文
溯源树
样例
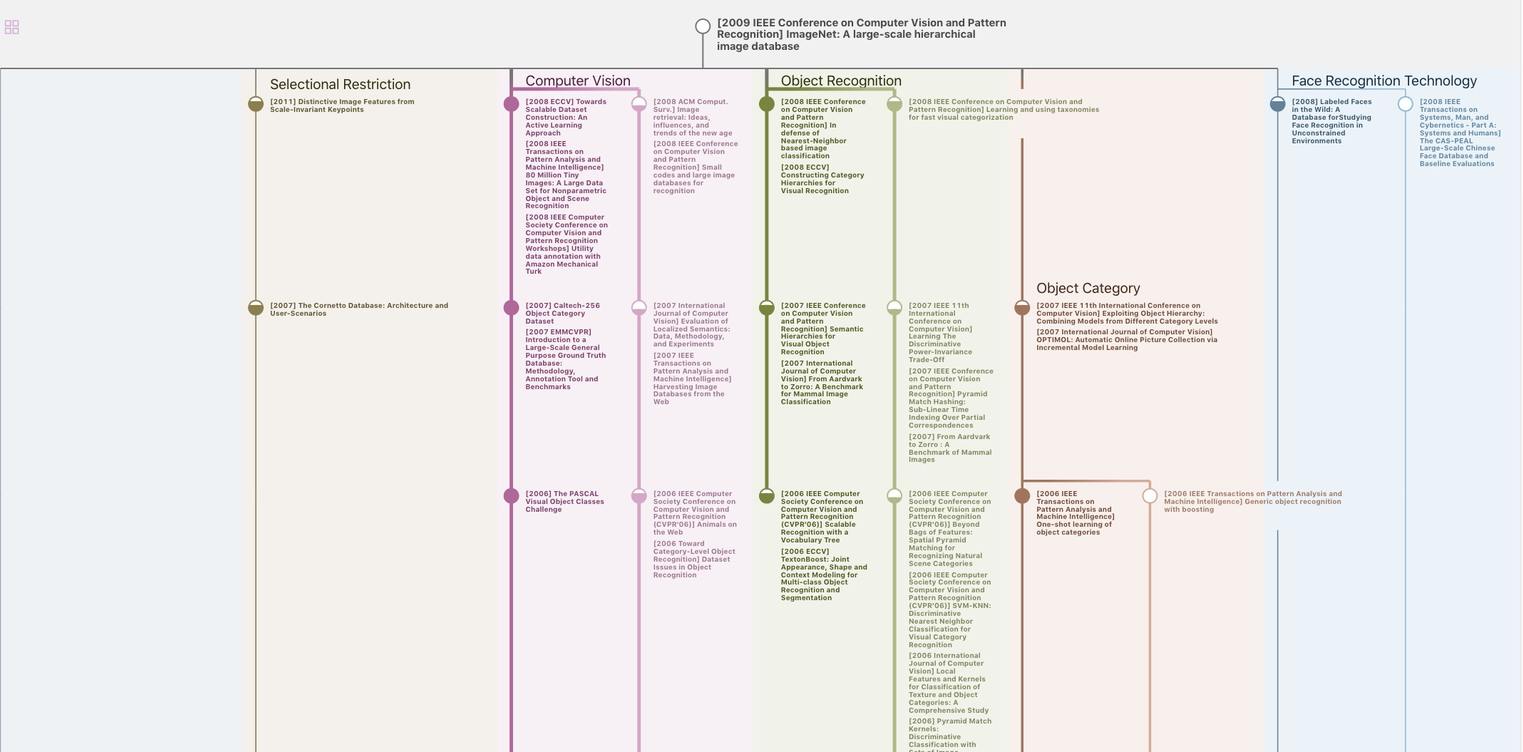
生成溯源树,研究论文发展脉络
Chat Paper
正在生成论文摘要