Robust multi-outcome regression with correlated covariate blocks using fused LAD-lasso
arxiv(2022)
摘要
Lasso is a popular and efficient approach to simultaneous estimation and variable selection in high-dimensional regression models. In this paper, a robust LAD-lasso method for multiple outcomes is presented that addresses the challenges of non-normal outcome distributions and outlying observations. Measured covariate data from space or time, or spectral bands or genomic positions often have natural correlation structure arising from measuring distance between the covariates. The proposed multi-outcome approach includes handling of such covariate blocks by a group fusion penalty, which encourages similarity between neighboring regression coefficient vectors by penalizing their differences for example in sequential data situation. Properties of the proposed approach are first illustrated by extensive simulations, and secondly the method is applied to a real-life skewed data example on retirement behavior with heteroscedastic explanatory variables.
更多查看译文
关键词
correlated covariate blocks,regression,multi-outcome,lad-lasso
AI 理解论文
溯源树
样例
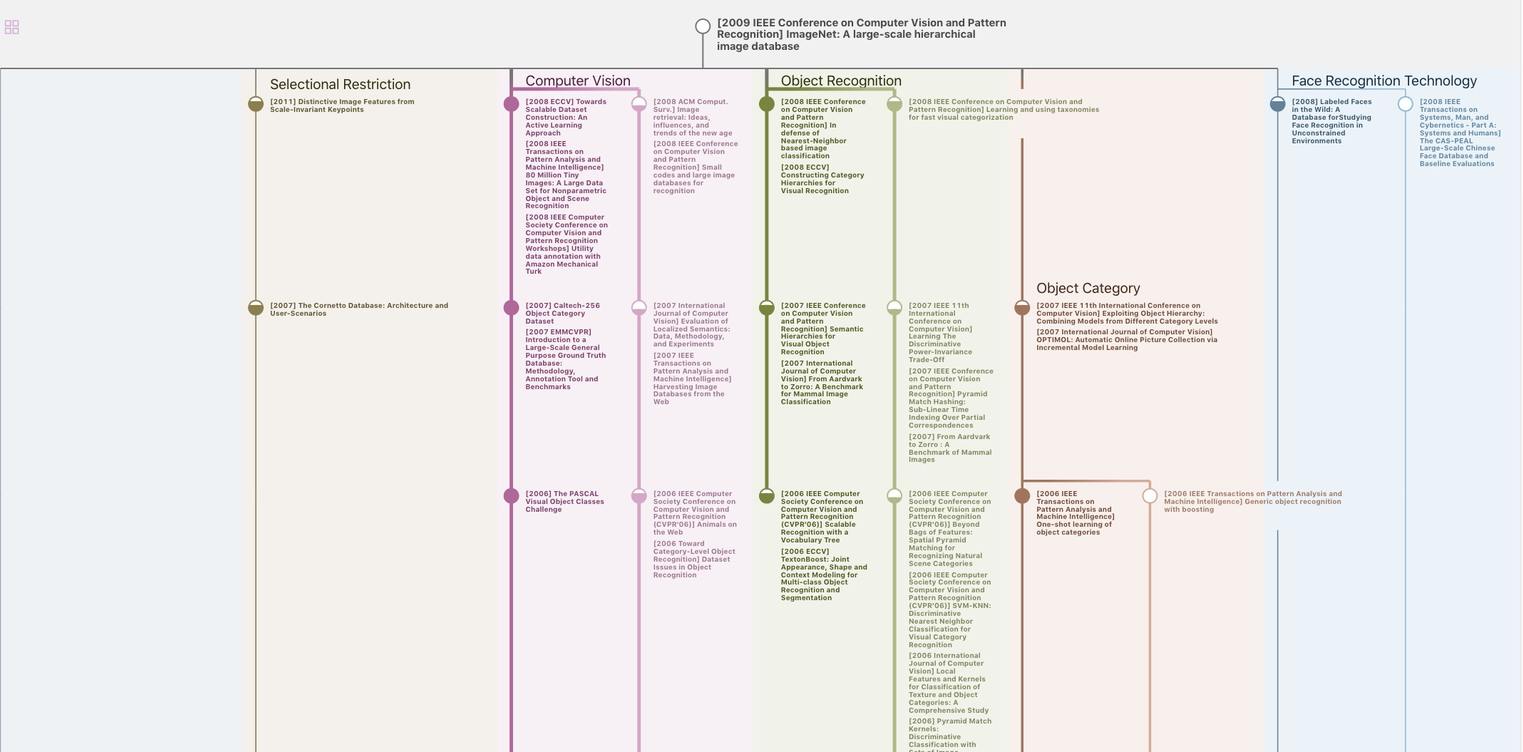
生成溯源树,研究论文发展脉络
Chat Paper
正在生成论文摘要