A Structure-Guided Effective and Temporal-Lag Connectivity Network for Revealing Brain Disorder Mechanisms
arxiv(2023)
摘要
Brain network provides important insights for the diagnosis of many brain disorders, and how to effectively model the brain structure has become one of the core issues in the domain of brain imaging analysis. Recently, various computational methods have been proposed to estimate the causal relationship (i.e., effective connectivity) between brain regions. Compared with traditional correlation-based methods, effective connectivity can provide the direction of information flow, which may provide additional information for the diagnosis of brain diseases. However, existing methods either ignore the fact that there is a temporal-lag in the information transmission across brain regions, or simply set the temporal-lag value between all brain regions to a fixed value. To overcome these issues, we design an effective temporal-lag neural network (termed ETLN) to simultaneously infer the causal relationships and the temporal-lag values between brain regions, which can be trained in an end-to-end manner. In addition, we also introduce three mechanisms to better guide the modeling of brain networks. The evaluation results on the Alzheimer's Disease Neuroimaging Initiative (ADNI) database demonstrate the effectiveness of the proposed method.
更多查看译文
关键词
Brain network,causal inference,temporal-lag estimation,brain disorder identification
AI 理解论文
溯源树
样例
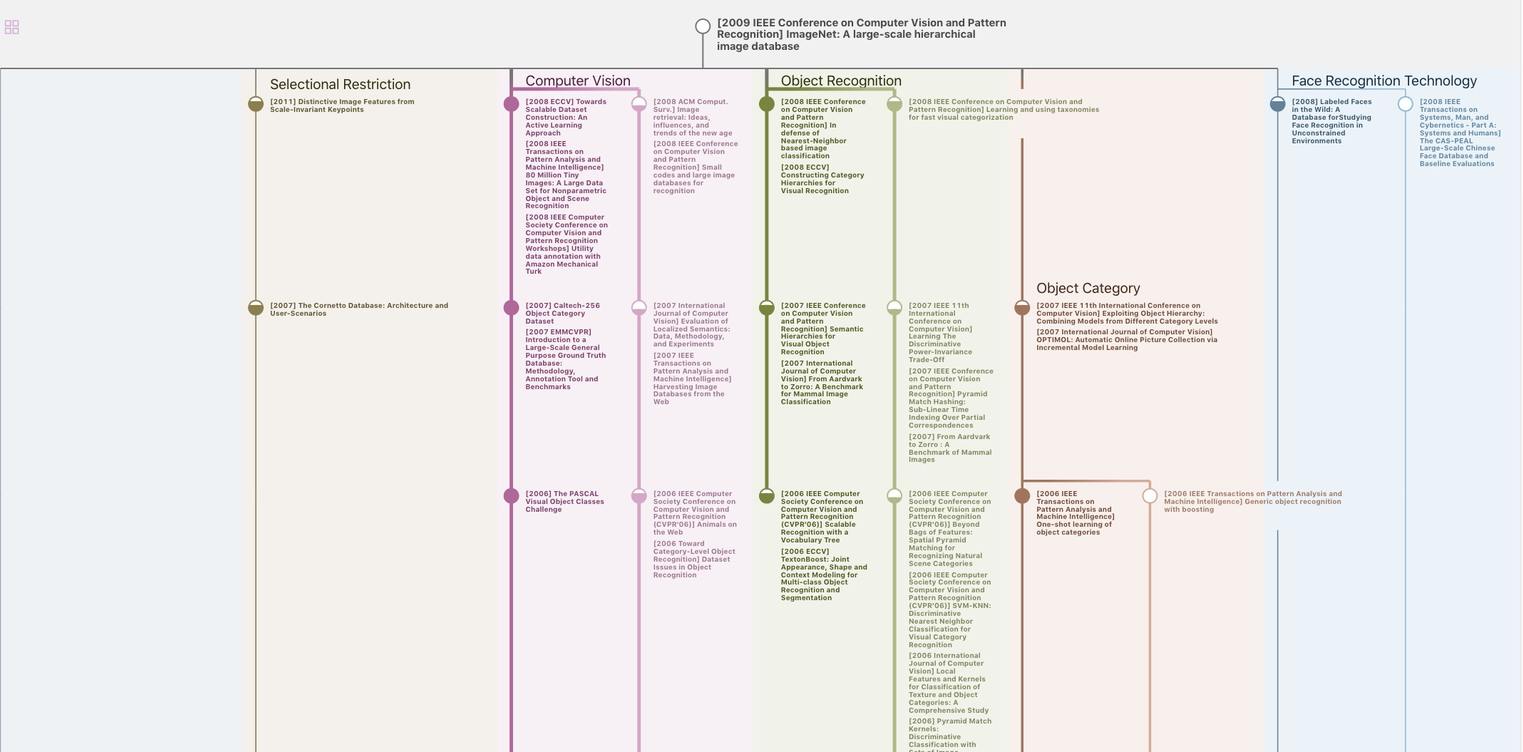
生成溯源树,研究论文发展脉络
Chat Paper
正在生成论文摘要