Scaling Language-Image Pre-Training via Masking
CVPR 2023(2023)
摘要
We present Fast Language-Image Pre-training (FLIP), a simple and more efficient method for training CLIP. Our method randomly masks out and removes a large portion of image patches during training. Masking allows us to learn from more image-text pairs given the same wall-clock time and contrast more samples per iteration with similar memory footprint. It leads to a favorable trade-off between accuracy and training time. In our experiments on 400 million image-text pairs, FLIP improves both accuracy and speed over the no-masking baseline. On a large diversity of downstream tasks, FLIP dominantly outperforms the CLIP counterparts trained on the same data. Facilitated by the speedup, we explore the scaling behavior of increasing the model size, data size, or training length, and report encouraging results and comparisons. We hope that our work will foster future research on scaling vision-language learning.
更多查看译文
关键词
language-image,pre-training
AI 理解论文
溯源树
样例
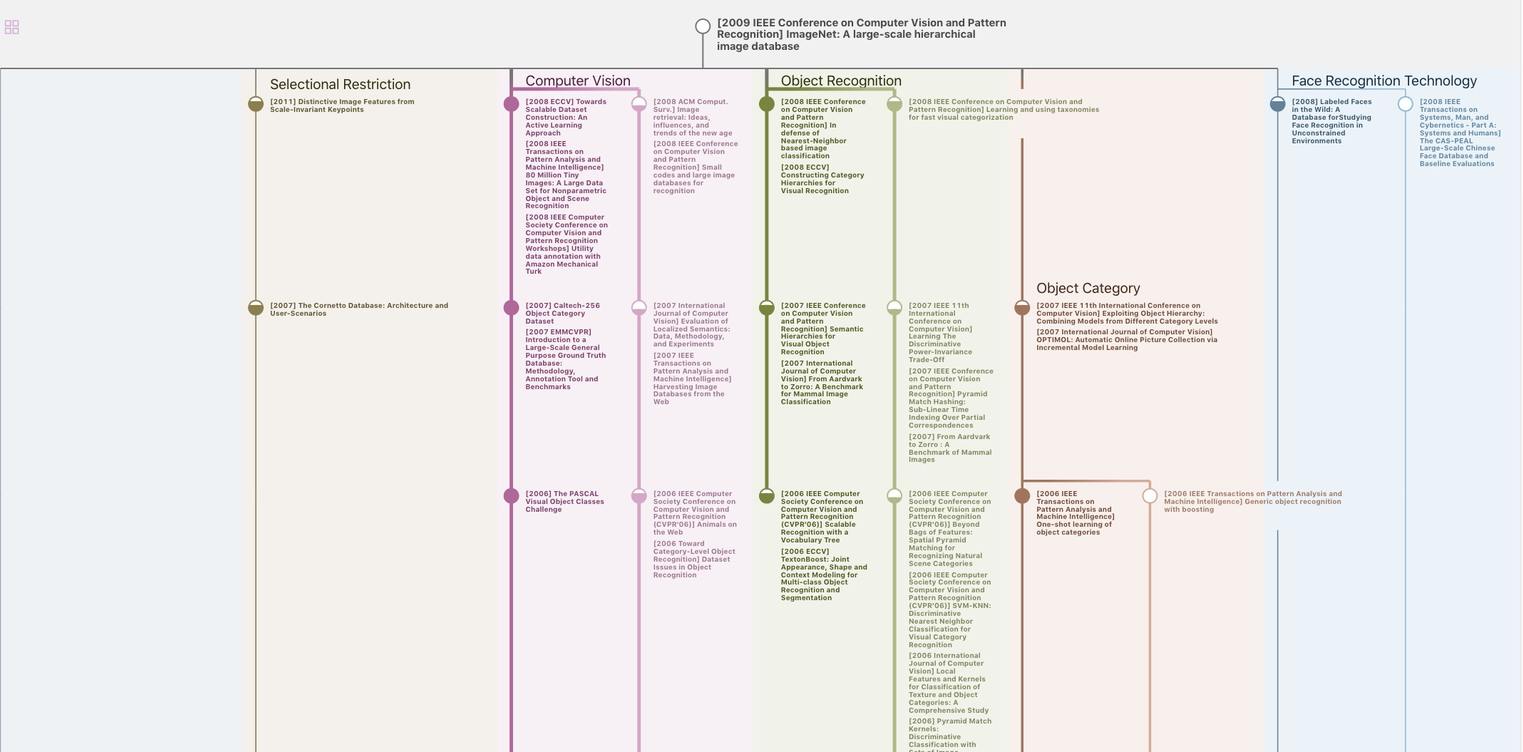
生成溯源树,研究论文发展脉络
Chat Paper
正在生成论文摘要