Change Detection in Moving-Camera Videos Using a Shift-Invariant Dissimilarity Metric
2022 10th European Workshop on Visual Information Processing (EUVIP)(2022)
摘要
This work addresses the problem of identifying anomalous objects in a cluttered environment using footage captured with moving cameras. The proposed method consists in the application of an edge-like feature extractor on the input images and in the learning of the space of non-anomalous features through deep autoencoder networks. Interestingly, this process is equivalent to employing a shift-invariant dissimilarity metric as an optimization target to the autoencoders. By the nature of the change detection task, it is necessary to collect and annotate large, diverse sets of anomalous conditions in order to train deep models in a supervised manner. The models trained in this proposal rely solely on anomalous-free data for parameter training, facilitating its application in a real-world scenario. The developed method was trained on the VDAO dataset, a challenging dataset with recordings of varied illumination situations. Unlike previous works using the same set, the autoencoder does not require the computationally expensive step of matching and registering a known reference frame to the tested frame that potentially contains an anomaly. Requiring not much more than a single network inference step, this proposal allows for real-time execution, even in systems with modest computational power. When tested against the VDAO200 dataset, consisting of 56 short excerpts of recordings of the VDAO scenes, this proposal matches the evaluation figures of the state-of-the-art algorithms, with measured DIS (distance to upper-left ROC corner) of 0.29 and an average precision score of 0.91.
更多查看译文
关键词
Anomaly detection,Video surveillance,Unsupervised learning,Deep learning,Manifold learning
AI 理解论文
溯源树
样例
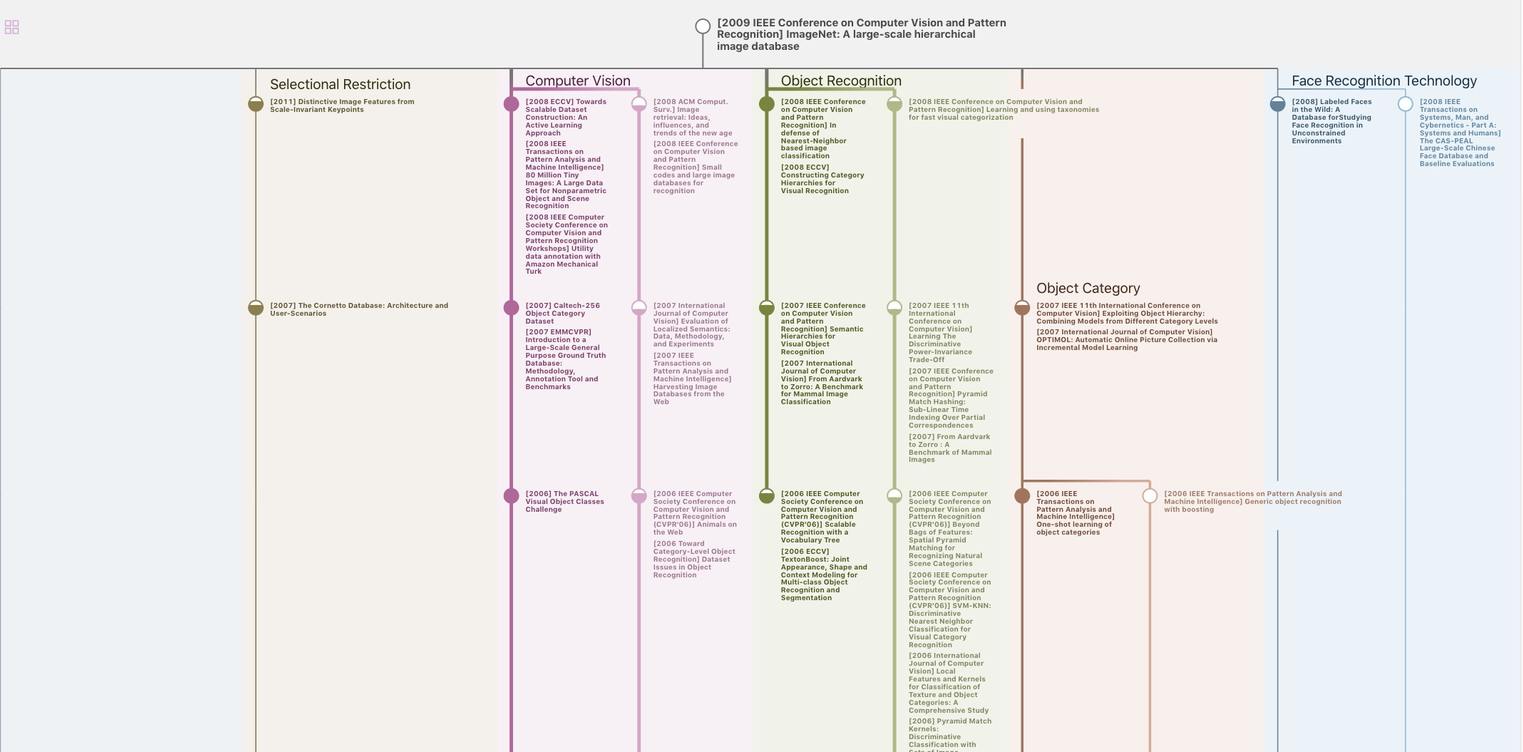
生成溯源树,研究论文发展脉络
Chat Paper
正在生成论文摘要