Data Booleanization for Energy Efficient On-Chip Learning using Logic Driven AI
2022 International Symposium on the Tsetlin Machine (ISTM)(2022)
摘要
The incorporation of Machine Learning (ML) models into smart devices and wearables has increased design focus on scalability and energy efficiency considerations. To transition towards on-device training, there must be a balance between data compaction to reduce ML complexity and data significance to maintain high learning efficacy. In this paper we examine a novel approach towards low-energy booleanization (i.e. Boolean encoding of raw data, which is different from binarization with positional significance used for encoding raw data) for a recently proposed machine learning method — the Tsetlin Machine. The approach in this paper offers a unique synergy between quantile binning for initial data encoding and logic minimization for significance-driven compaction of the dataset. Through this approach, the paper demonstrates how energy-frugal Tsetlin Machine models can be created with, for some datasets, up to 38.8x less energy consumption per datapoint during training. The proposed method offers significantly better energy efficiency with similar performance when compared with Binary Neural Network counterparts.
更多查看译文
关键词
Tsetlin machine,machine learning,quantile binning,booleanization,logic minimization
AI 理解论文
溯源树
样例
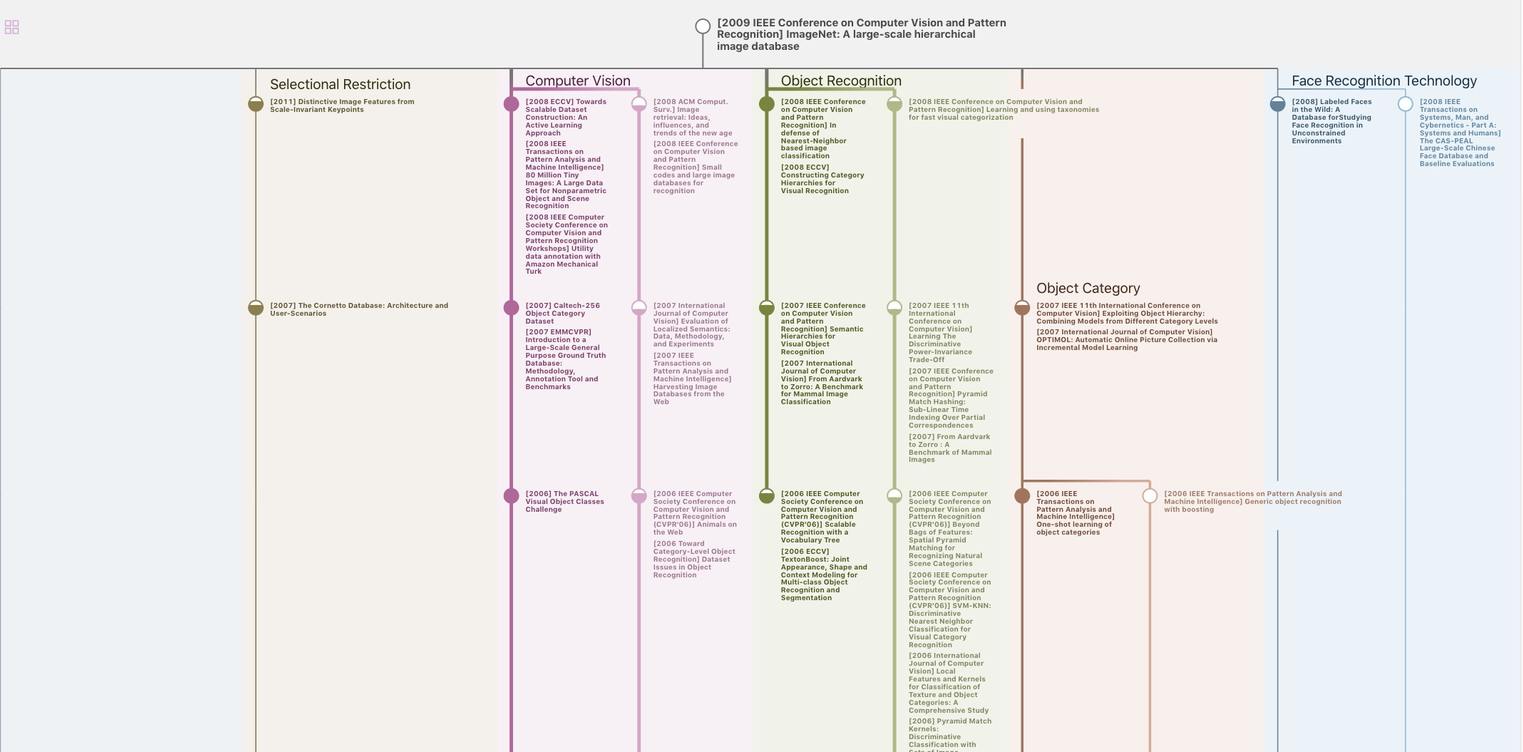
生成溯源树,研究论文发展脉络
Chat Paper
正在生成论文摘要