A Hardware/Software Co-Design Vision for Deep Learning at the Edge
IEEE Micro(2022)
摘要
The growing popularity of edgeAI requires novel solutions to support the deployment of compute-intense algorithms in embedded devices. In this article, we advocate for a holistic approach, where application-level transformations are jointly conceived with dedicated hardware platforms. We embody such a stance in a strategy that employs ensemble-based algorithmic transformations to increase robustness and accuracy in convolutional neural networks, enabling the aggressive quantization of weights and activations. Opportunities offered by algorithmic optimizations are then harnessed in domain-specific hardware solutions, such as the use of multiple ultra-low-power processing cores, the provision of shared acceleration resources, the presence of independently power-managed memory banks, and voltage scaling to ultra-low levels, greatly reducing (up to 60% in our experiments) energy requirements. Furthermore, we show that aggressive quantization schemes can be leveraged to perform efficient computations directly in memory banks, adopting in-memory computing solutions. We showcase that the combination of parallel in-memory execution and aggressive quantization leads to more than 70% energy and latency gains compared to baseline implementations.
更多查看译文
关键词
Optimization,Quantization (signal),Convolutional neural networks,Hardware,In-memory computing,Edge computing,Robustness,Artificial intelligence,Deep learning
AI 理解论文
溯源树
样例
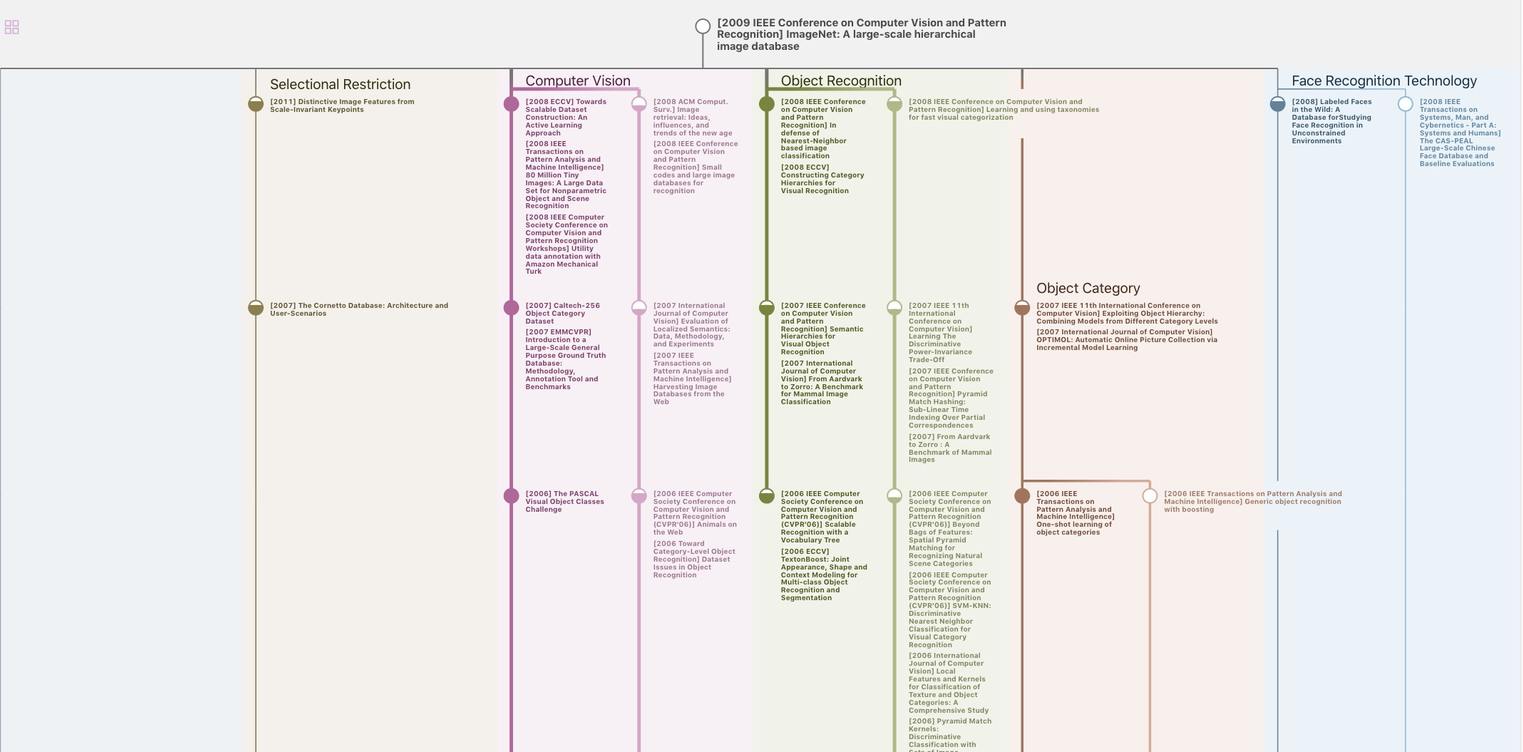
生成溯源树,研究论文发展脉络
Chat Paper
正在生成论文摘要