Neural-Network-Based Automated Synthesis of Transformer Matching Circuits for RF Amplifier Design
IEEE Transactions on Microwave Theory and Techniques(2022)
摘要
Rich experience and intuition play important roles in designing planar transformers (TFs) for contemporary radio frequency integrated circuits (RFICs). In general, RFIC designers have been heavily relying on multiple iterations of full electromagnetic (EM) simulations, which consumes much time and effort. Here, we propose an automated matching circuit synthesizer (AMCS) using neural networks (NNs). The proposed AMCS directly synthesizes a matching circuit combined with a TF throughout the entire design process, ranging from the desired performance to layout. In the AMCS, which is a “spec-to-layout” synthesizer, one NN returns physical parameters of matching circuits, and another NN estimates the electrical performance in two-port
$S$
-parameters from the desired impedances. Before the NNs are trained, input feature design is conducted to avoid the one-to-many problem, which cannot be well characterized with an inverse NN. This significantly reduces the time and effort for iterative circuit and EM simulations. The AMCS generated the matching circuit layouts for simple single-stage amplifiers operating at different frequencies up to 70 GHz or in different bandwidths of up to 32.5%. The estimated
$S$
-parameters of the amplifiers show good agreement with the EM simulation results.
更多查看译文
关键词
Automated synthesis,neural network (NN),radio frequency (RF) amplifiers,radio frequency integrated circuit (RFIC),transformer (TF)
AI 理解论文
溯源树
样例
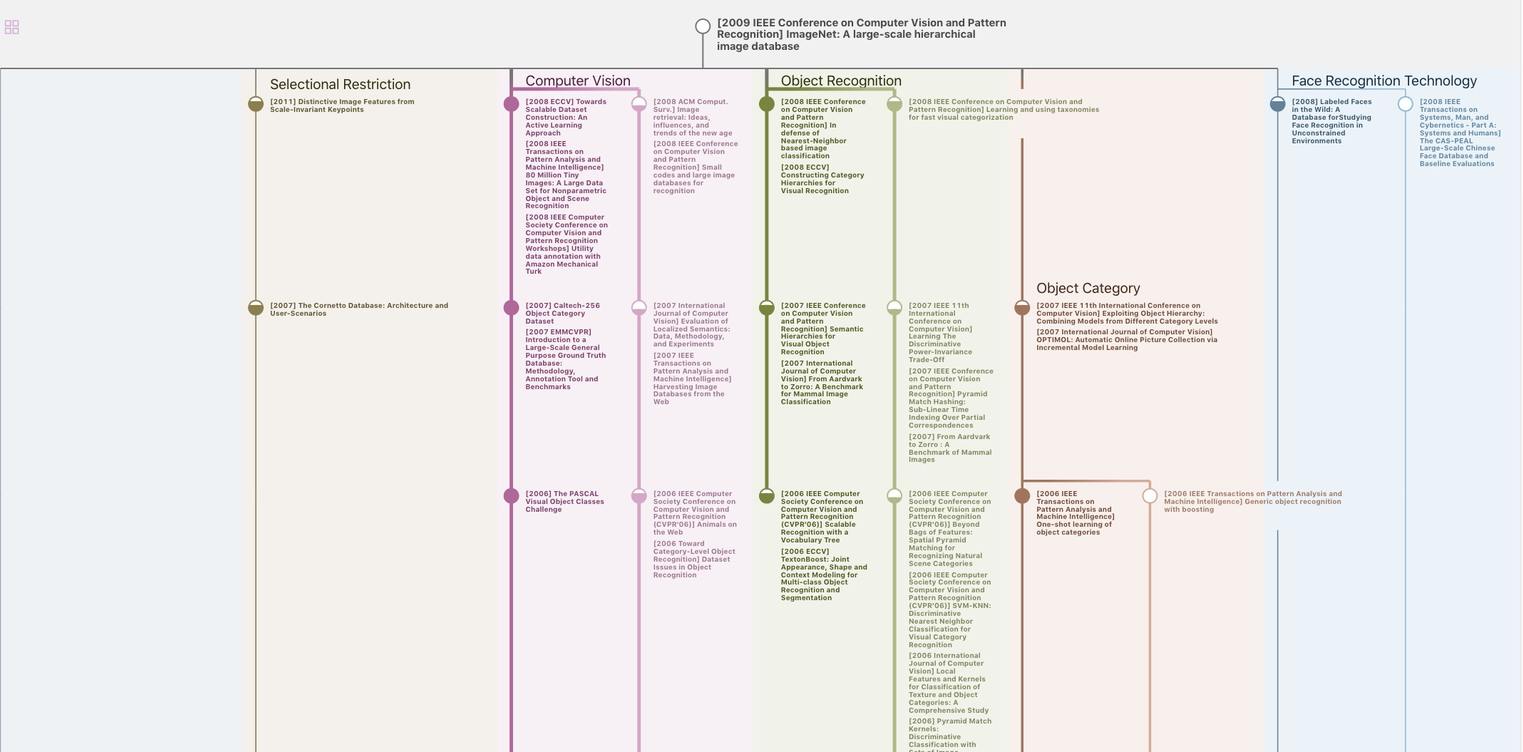
生成溯源树,研究论文发展脉络
Chat Paper
正在生成论文摘要