Energy-efficient Federated Learning via Stabilization-aware On-device Update Scaling
2022 19th Annual IEEE International Conference on Sensing, Communication, and Networking (SECON)(2022)
摘要
Federated learning is emerging as a major learning paradigm, which enables multiple devices to train a model col-laboratively and to keep the privacy of data. However, substantial computation-intensive iterations are performed on devices before the training completion, which incurs heavy consumption of the energy. Along with the stabilization of those model parameters being trained, such on-device training iterations are redundant gradually over time. Thus, we propose to scale the update results obtained from reduced iterations as the substitute for on-device training, based on current model status and device heterogeneity. We thus formulate a time-varying integer program, to minimize cumulative energy consumption over devices, subject to a long-term constraint regarding the model convergence. We then design a polynomial-time online algorithm upon system dynamics, which essentially balances the energy consumption and the model quality being trained. Via rigorous proofs, our approach only incurs sub linear regret, compared with its optimum, and ensures related model convergence. Extensive testbed experiments for real training confirm the superiority of our approach, over multiple alternatives, under various scenarios, decreasing at least 30.2% energy consumption, while preserving the accuracy of the model.
更多查看译文
关键词
on-device training iterations,time-varying integer program,cumulative energy consumption,polynomial-time online algorithm,energy-efficient federated learning,stabilization-aware on-device update,data privacy,computation-intensive iterations
AI 理解论文
溯源树
样例
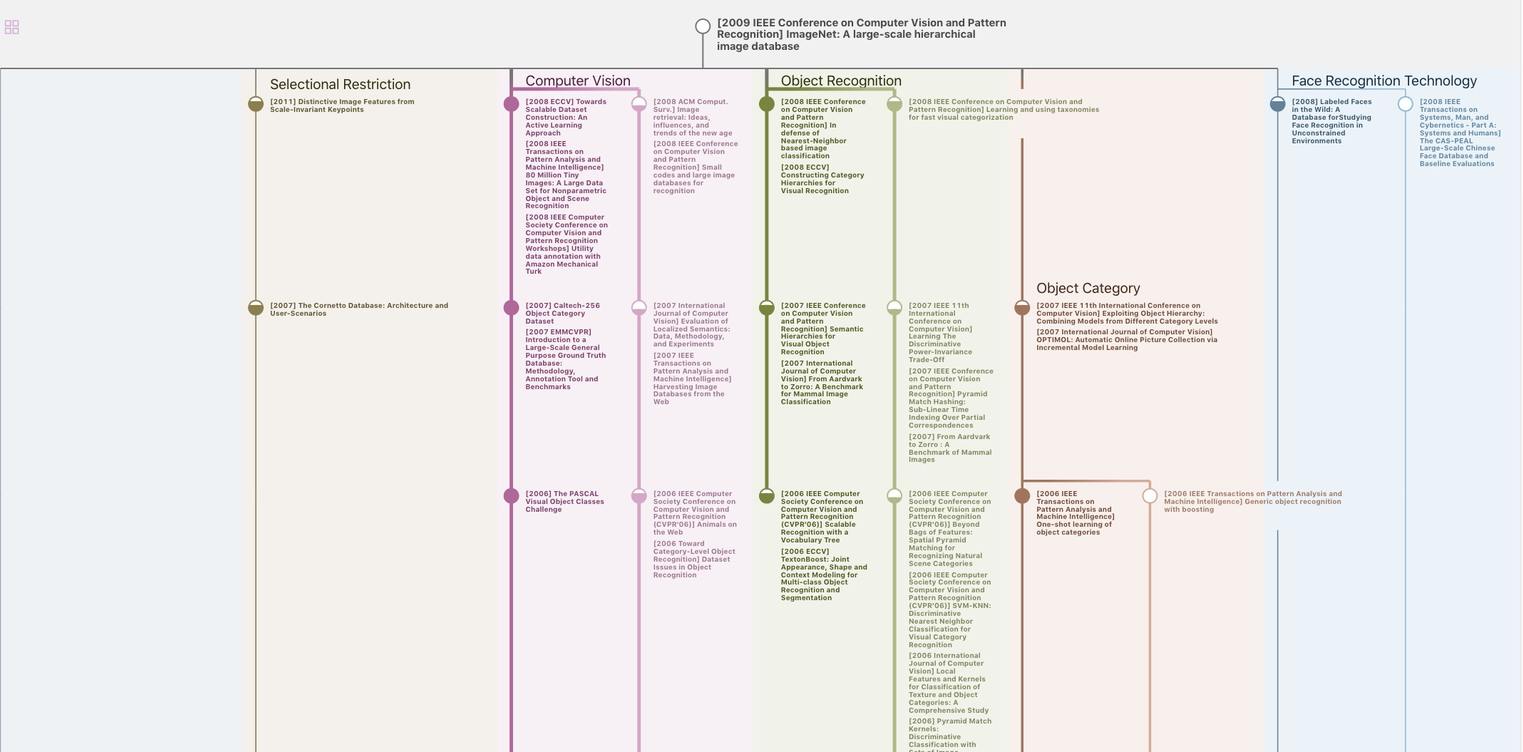
生成溯源树,研究论文发展脉络
Chat Paper
正在生成论文摘要