Facilitating Radar-Based Gesture Recognition With Self-Supervised Learning
2022 19th Annual IEEE International Conference on Sensing, Communication, and Networking (SECON)(2022)
摘要
With deep learning, millimeter-wave radar-based gesture recognition applications have achieved satisfactory results. However, most existing approaches highly rely on highquality labeled data, and they suffer from severe over-fitting when labeled data are scarce. To end this, we present RadarAE, a novel representation learning framework for radar sensing applications. RadarAE learns sophisticated representations from massive low-cost unlabeled radar data, which enables accurate gesture recognition with few labeled data. To achieve this goal, we first meticulously observe the characteristics of raw radar data and extract an effective feature, Spatio-Temporal Motion Map (STMM). Then we borrow the key principle of Masked Autoencoders (MAE), a self-supervised learning technique for images, and propose an MAE-like model to learn useful representations from STMM. To adapt RadarAE to radar sensing applications, we present a series of customization techniques, including data augmentation, optimized model structure, and adaptive pretraining method. With the learned high-level representations, gesture recognition models can achieve superior performance in few-shot scenarios. Experiment results show that our model can achieve 79.1%, 92.1%, 97.8%, and 99.5% recognition accuracy in the 1, 2, 4, and 8-shot scenarios, respectively, where x-shot refers to the number of labeled samples for each gesture type. The source codes and dataset are made publicly available
1
1
https://githuh.com/Ela-Boska/RadarAE.
更多查看译文
关键词
Human-Computer Interaction,Millimeter-Wave Radar,Gesture recognition,Self-Supervised Learning
AI 理解论文
溯源树
样例
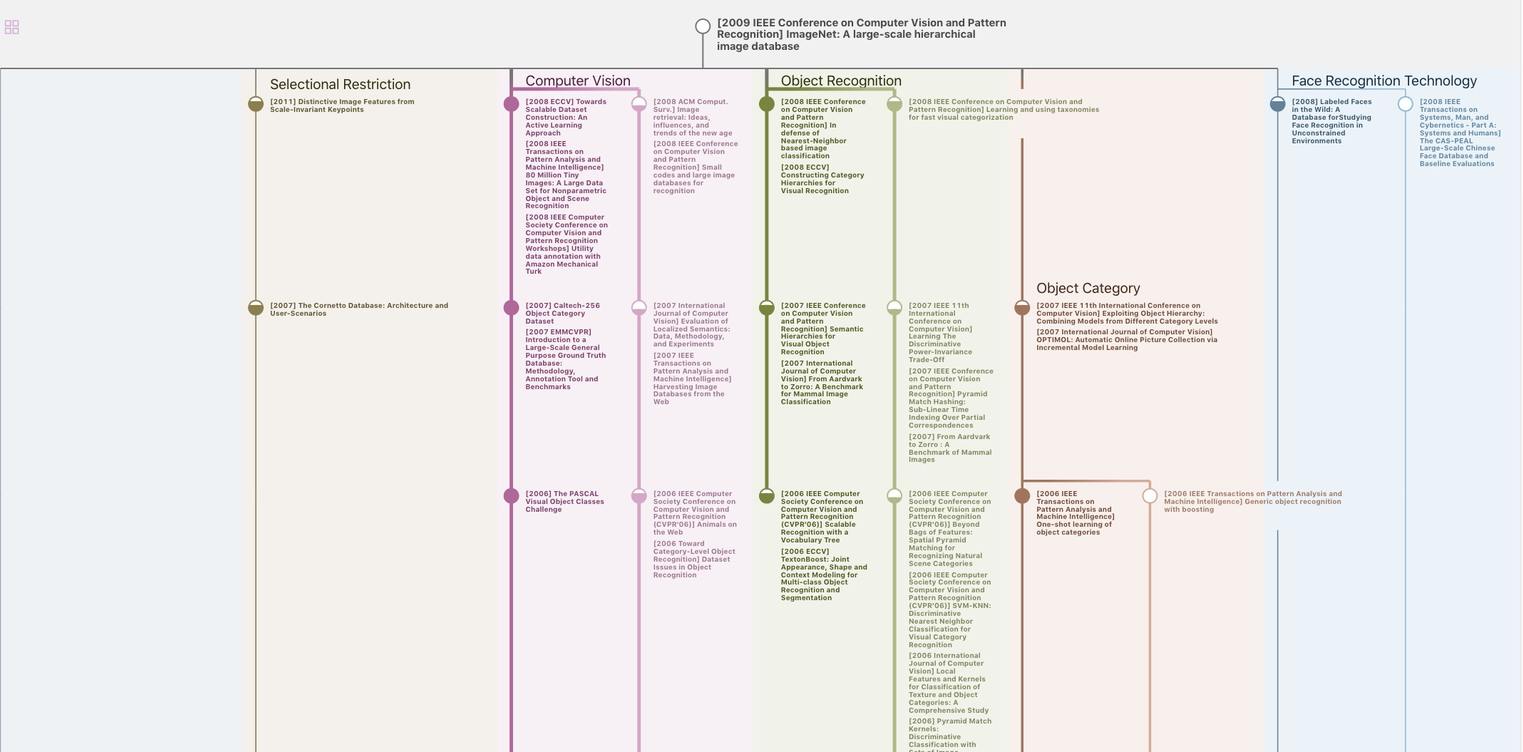
生成溯源树,研究论文发展脉络
Chat Paper
正在生成论文摘要