On Leveraging FemtoClouds for Federated Learning
IEEE Internet of Things Magazine(2022)
摘要
The massive amount of data generated by mobile/IoT devices worldwide has been both a motivation and a key enabler for Machine Learning (ML)-based applications. Cloud and Edge platforms have been traditionally leveraged as underlying systems where data for ML applications is processed using “Centralized ML.” Federated Learning (FL) was introduced as an alternative solution that provides comparable accuracy, to centralized-ML, while maintaining user privacy. FL allows training data on its source device and only model updates can leave the device for learning aggregation at the Cloud/Edge. However, current FL solutions have limitations that originate from the training process and current system implementations. Hence, in this paper, we propose leveraging FemtoClouds (i.e. ensembles of co-located mobile/IoT devices) as a complementary system to existing FL approaches. We discuss and assess the benefits that FemtoClouds introduce for both the FL training algorithms, and end devices. These benefits include improvements in training accuracy, convergence speed, training time, and energy efficiency. We finally discuss challenges and future research directions that need to be addressed to realize the benefits of Femto Clouds-based FL.
更多查看译文
关键词
federated learning,femtoclouds
AI 理解论文
溯源树
样例
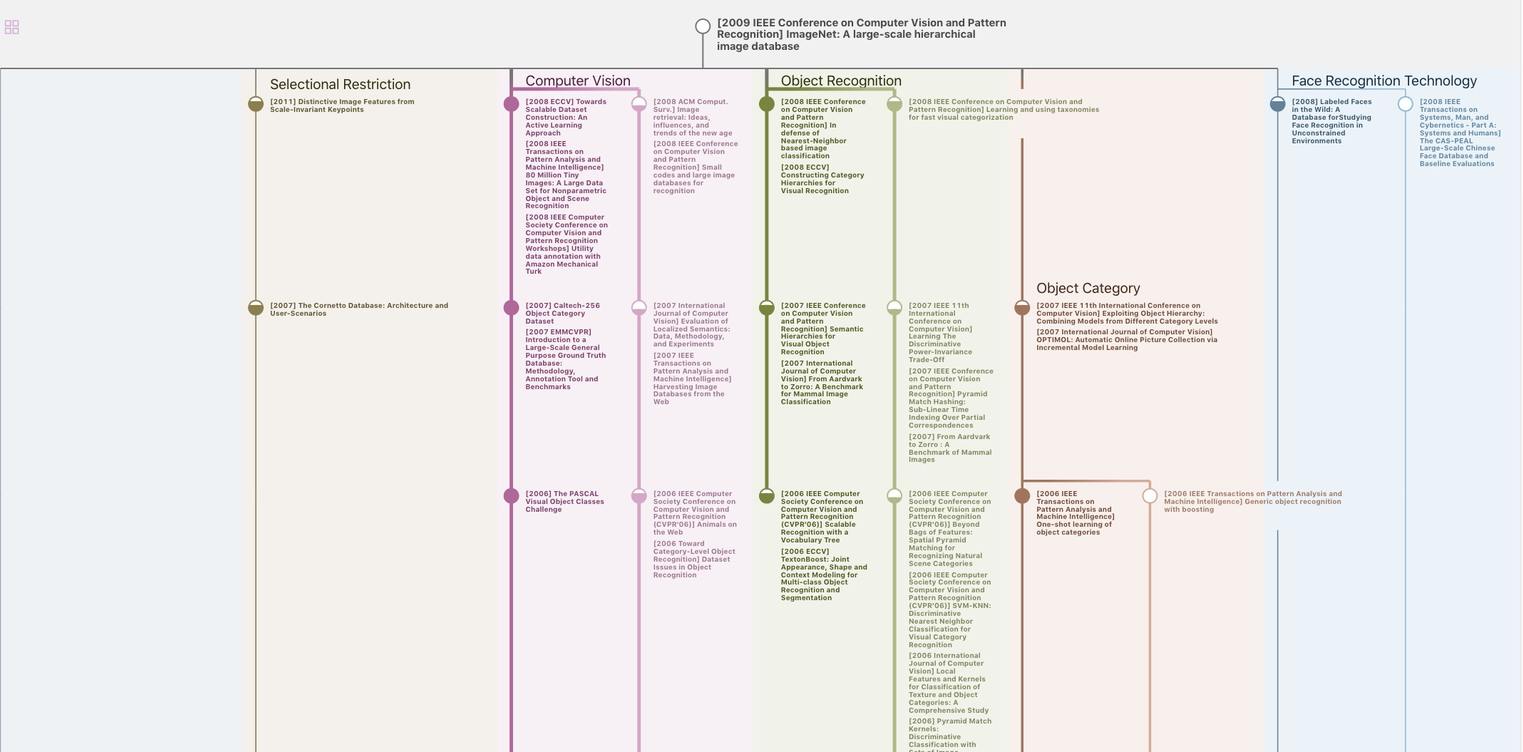
生成溯源树,研究论文发展脉络
Chat Paper
正在生成论文摘要