Automatic Terminology Extraction and Ranking for Feature Modeling
2022 IEEE 30th International Requirements Engineering Conference (RE)(2022)
摘要
Requirements terminology defines and unifies key specialized and/or technical concepts of the software system, which is significant for understanding the application domain in requirements engineering (RE). However, manual terminology extraction from natural language requirements is laborious and expensive, especially with large scale requirements specifications. In this paper, we aim to employ natural language processing (NLP) techniques and machine learning (ML) algorithms to automatically extract and rank the requirements terms to support high-level feature modeling. To this end, we propose an automatic framework composed of noun phrase identification technique for requirements terms extraction and TextRank combined with semantic similarity for terms ranking. The final ranked terms are organized as a hierarchy, which can be used to help name elements when performing feature modeling. In the quantitative evaluation, our extraction method performs better than three baseline methods in recall with comparable precision. Moreover, our adapted TextRank algorithm can rank more relevant terms at the top positions in terms of average precision compared with most baselines. An illustrative example on the smart home domain further shows the usefulness of our framework in aiding elements naming during feature modeling. The research results suggest that proper adoption and adaption of NLP and ML techniques according to the characteristics of specific RE task could provide automation support for problem domain understanding.
更多查看译文
关键词
terminology extraction,TextRank,text mining,requirements analysis,feature modeling
AI 理解论文
溯源树
样例
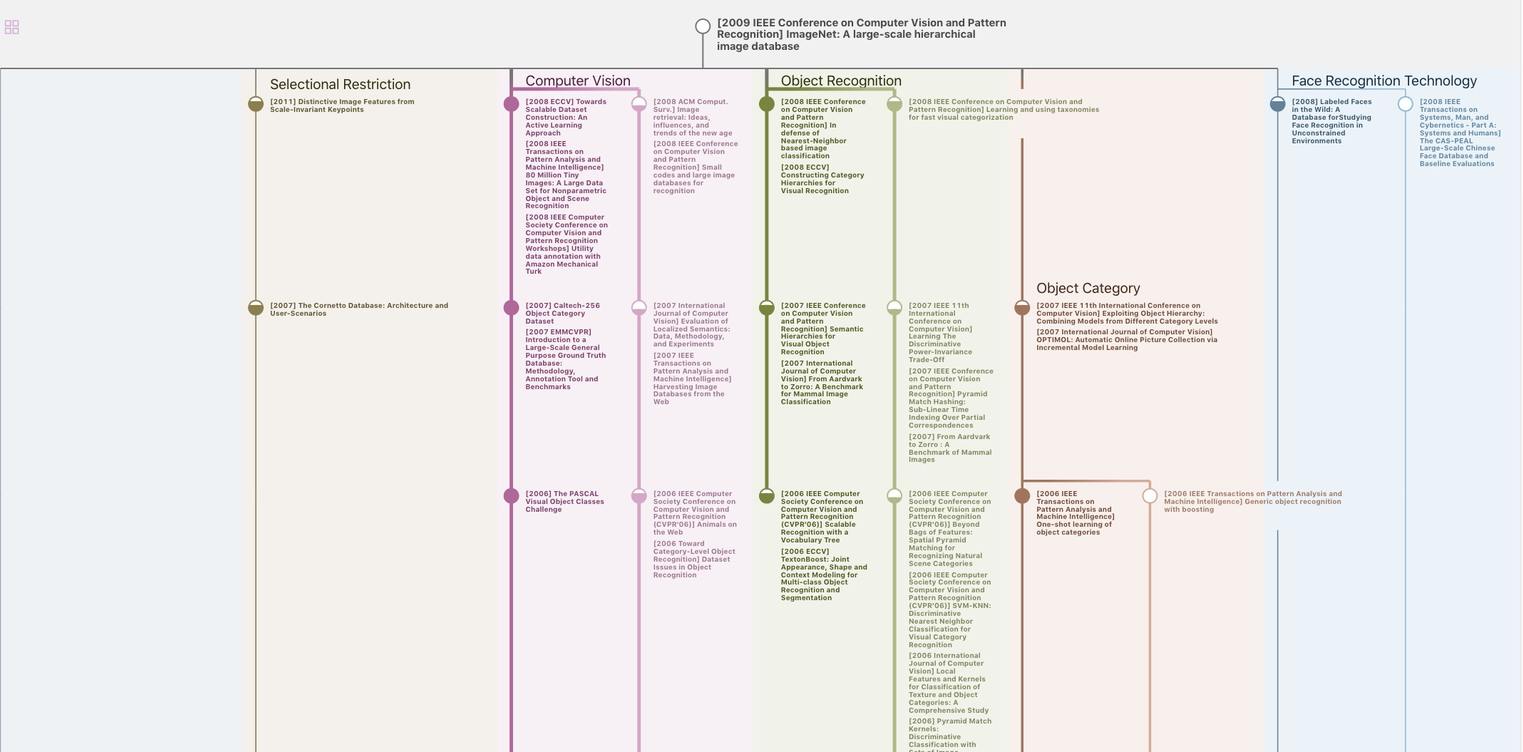
生成溯源树,研究论文发展脉络
Chat Paper
正在生成论文摘要