Neutron-induced Faults on CNN for Aerial Image Classification on SRAM-based FPGA Using Softcore GPU and HLS
2021 21th European Conference on Radiation and Its Effects on Components and Systems (RADECS)(2021)
摘要
This work evaluates neutron-induced soft errors in an image classification all-convolutional neural network implemented on an SRAM-based FPGA. We compare two implementations, an open-source general-purpose softcore GPU programmed in OpenCL language and a dedicated convolution engine obtained by high-level synthesis coded in C language. Results from a 14.1 MeV neutron irradiation have shown that the HLS architecture presents better reliability than the CNN running on the softcore GPU, due to optimized resources, while also reducing in six times the execution time and in five times the power consumption.
更多查看译文
关键词
Artificial neural networks,programmable logic,radiation effects,graphics processing units,high-level synthesis
AI 理解论文
溯源树
样例
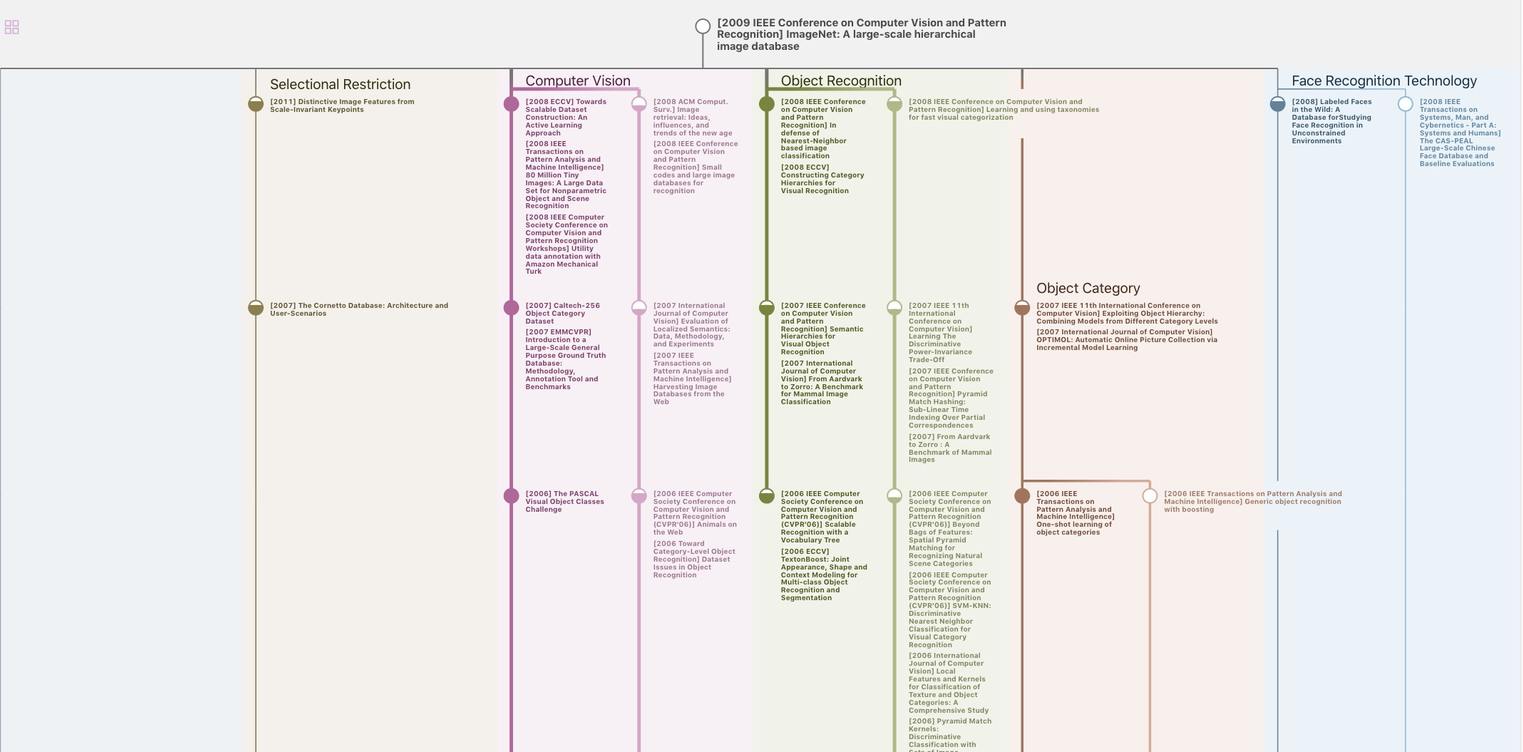
生成溯源树,研究论文发展脉络
Chat Paper
正在生成论文摘要