Learning High Dimensional Multi-response Linear Models with Non-oracular Quantum Search
2022 IEEE International Conference on Quantum Computing and Engineering (QCE)(2022)
摘要
This paper studies linear regression models for high dimensional multi-response data with a hybrid quantum computing algorithm. We propose an intuitively appealing estimation method based on identifying the linearly independent columns in the coefficient matrix. Our method relaxes the low rank constraint in the existing literature and allows the rank to diverge with dimensions. The linearly independent columns are selected by a novel non-oracular quantum search (NQS) algorithm which is significantly faster than classical search methods implemented on electronic computers. Besides, NQS achieves a near optimal computational complexity as existing quantum search algorithms but does not require any oracle information of the solution state. We prove the proposed estimation procedure enjoys desirable theoretical properties. Intensive numerical experiments are also conducted to demonstrate the finite sample performance of the proposed method, and a comparison is made with some popular competitors. The results show that our method outperforms all of the alternative methods under various circumstances.
更多查看译文
关键词
high dimensional data,Grover’s algorithm,multi-response linear model,non-oracular quantum search,quantum machine learning
AI 理解论文
溯源树
样例
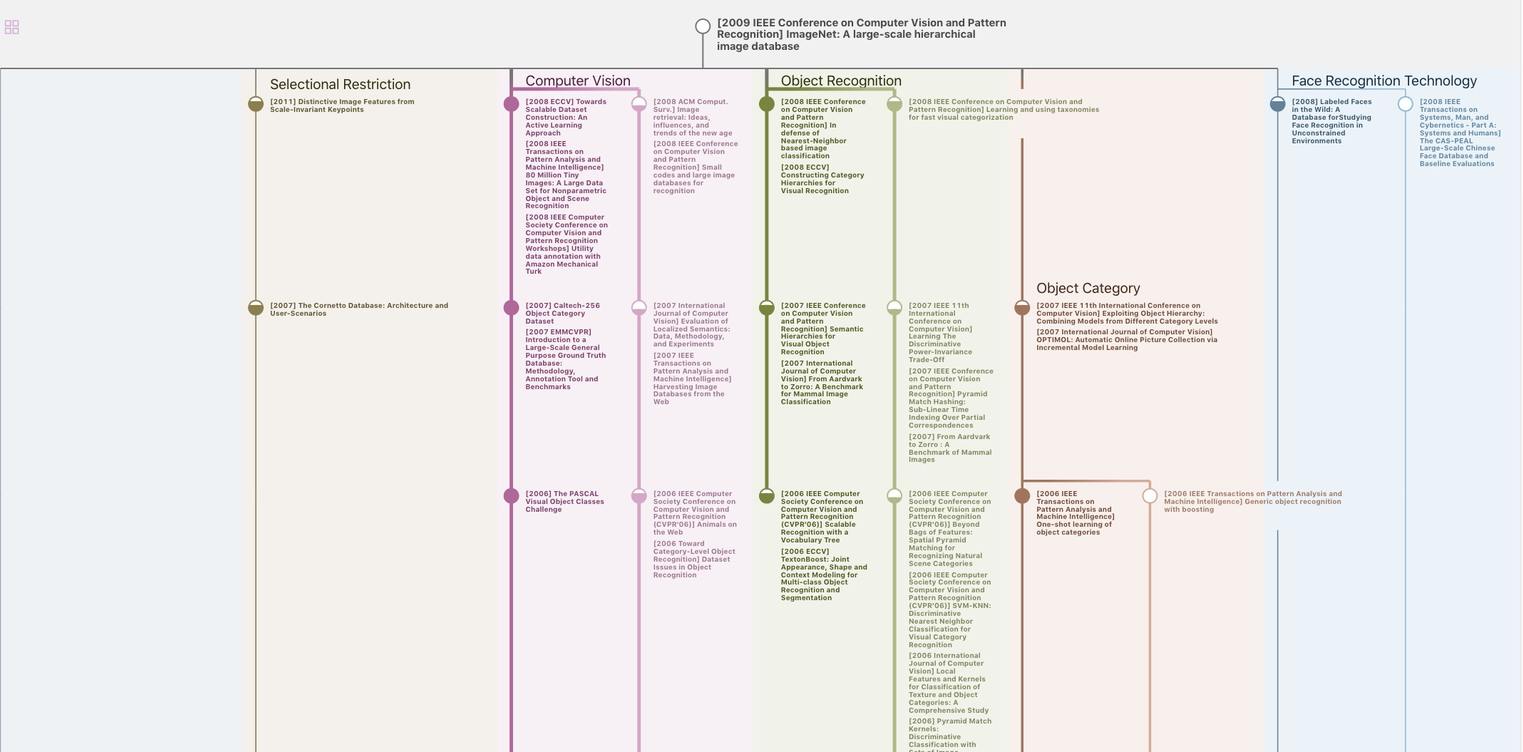
生成溯源树,研究论文发展脉络
Chat Paper
正在生成论文摘要