Occlusion-perturbed Deep Learning for Probabilistic Solar Forecasting via Sky Images
2022 IEEE Power & Energy Society General Meeting (PESGM)(2022)
摘要
Solar forecasting is shifting to the probabilistic paradigm due to the inherent uncertainty within the solar resource. Input uncertainty quantification is one of the widely-used and best-performing ways to model solar uncertainty. However, compared to other sources of inputs, such as numerical weather prediction models, pure sky image-based probabilistic solar forecasting lags behind. In this research, an occlusion-perturbed convolutional neural network, named the PSolarNet, is developed. The PSolarNet provides very short-term deterministic forecasts, forecast scenarios, and probabilistic forecasts of the global horizontal irradiance from sky image sequences. Case studies based on 6 years of open-source data show that the developed PSolarNet is able to generate accurate to-minute ahead deterministic forecasts with a 5.62% normalized root mean square error, realistic and diverse forecast scenarios with a 0.966 average correlation with the actual time series, and reliable and sharp probabilistic forecasts with a 2.77% normalized continuous ranked probability score.
更多查看译文
关键词
Deep learning,solar forecasting,sky image processing,Bayesian model averaging
AI 理解论文
溯源树
样例
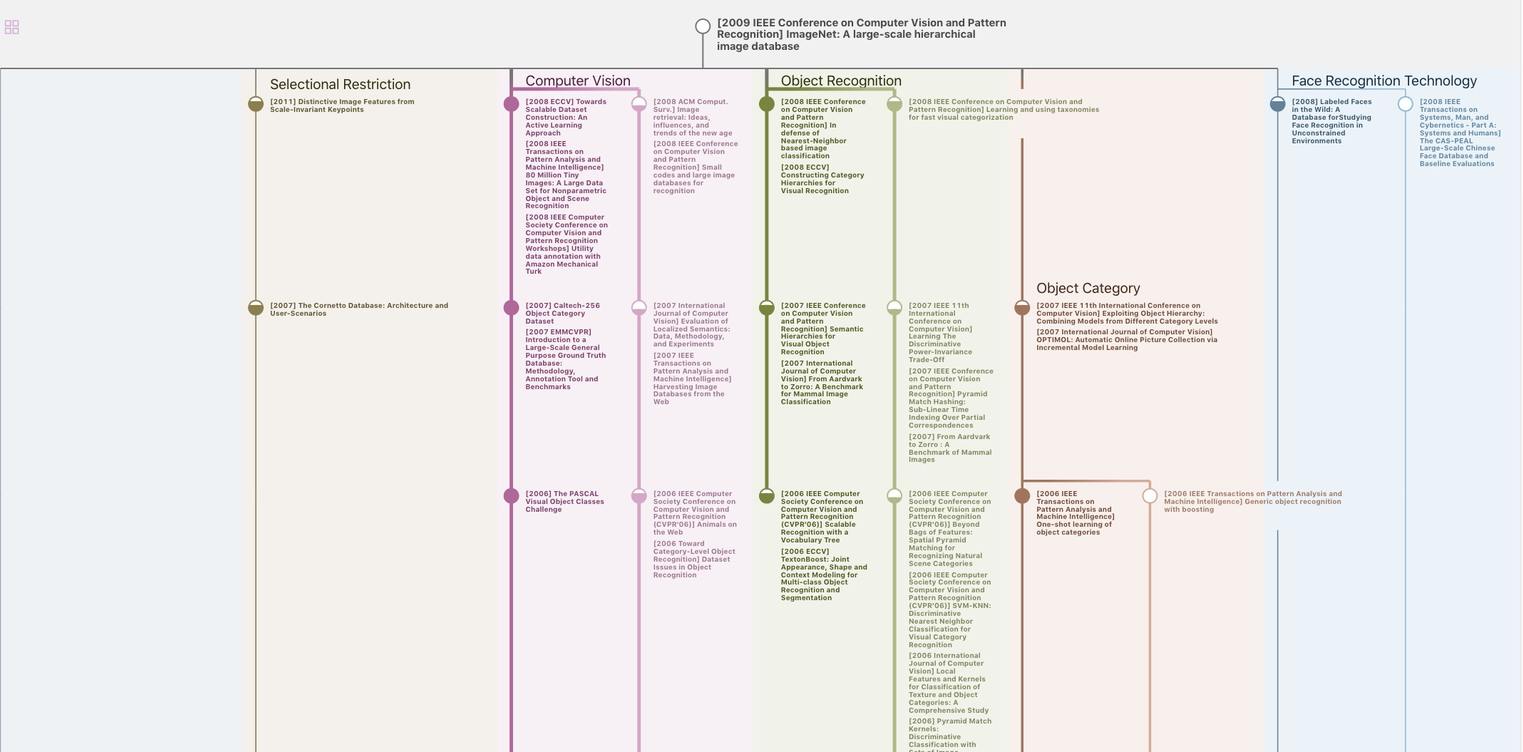
生成溯源树,研究论文发展脉络
Chat Paper
正在生成论文摘要