Entropy regularization methods for parameter space exploration
Information Sciences(2023)
摘要
Entropy regularization is an important approach to improve exploration and enhance pol-icy stability for reinforcement learning. However, in previous study, entropy regularization is applied only to action spaces. In this paper, we apply entropy regularization to parameter spaces. We use learnable noisy layers to parameterize the policy network to obtain a learn-able entropy. Also, we derive the expression for the entropy of the noisy parameter and an upper bound on the joint entropy. Based on these, we propose a model-free method named deep pseudo deterministic policy gradients based on entropy regularization (DPGER). This method maximizes the entropy of each noisy parameter in the early learning process to promote exploration, and minimizes the joint entropy of the noisy parameters in the later learning process to facilitate the formation of stable policies. We test our method on four Mujoco environments with five random seeds. The results show that our method brings better performance compared to previous methods. (c) 2022 Elsevier Inc. All rights reserved.
更多查看译文
关键词
Reinforcement learning,Entropy regularization,Exploration,Parameter spaces,Deterministic policy gradients
AI 理解论文
溯源树
样例
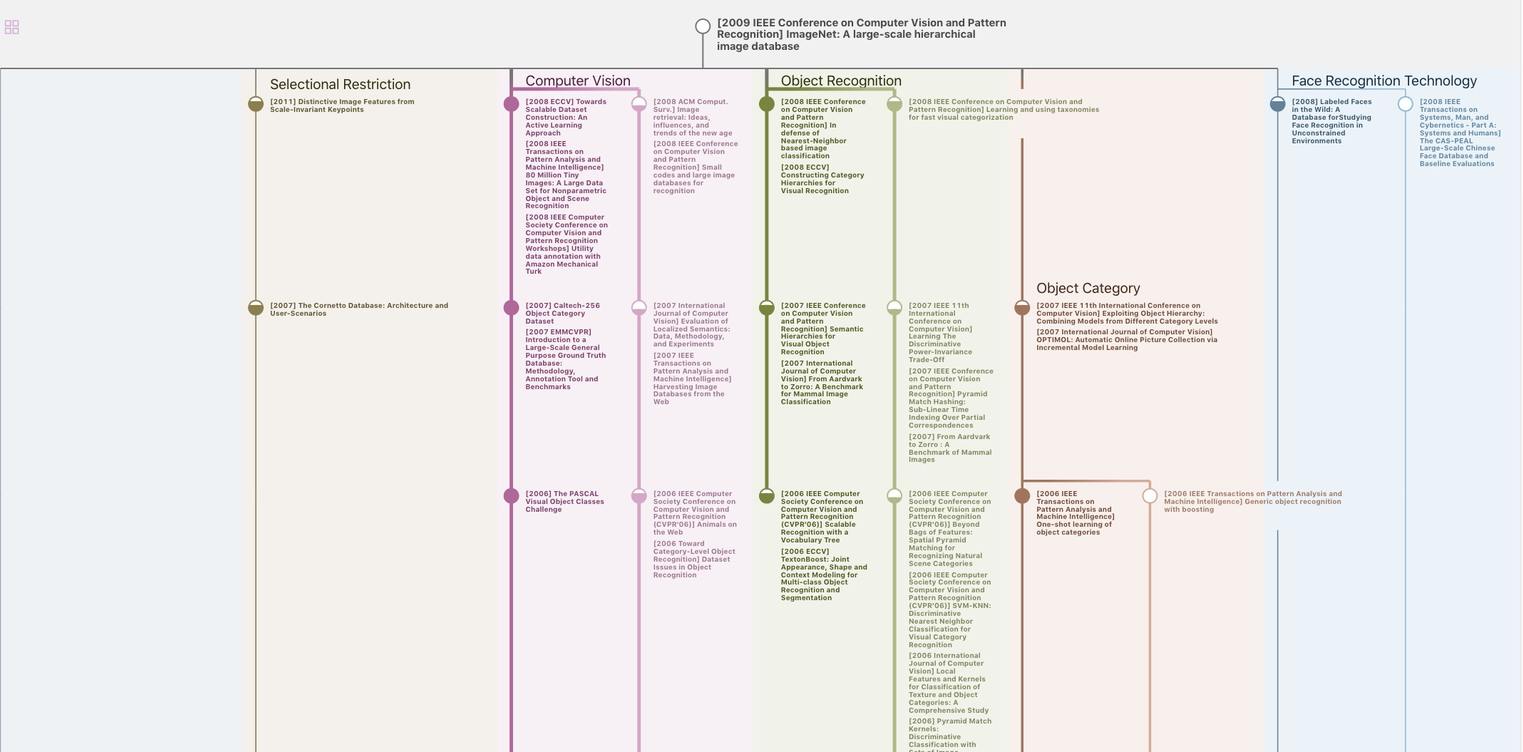
生成溯源树,研究论文发展脉络
Chat Paper
正在生成论文摘要