GH-Feat: Learning Versatile Generative Hierarchical Features from GANs
IEEE transactions on pattern analysis and machine intelligence(2022)
摘要
Recent years witness the tremendous success of generative adversarial networks (GANs) in synthesizing photo-realistic images. GAN generator learns to compose realistic images and reproduce the real data distribution. Through that, a hierarchical visual feature with multi-level semantics spontaneously emerges. In this work we investigate that such a generative feature learned from image synthesis exhibits great potentials in solving a wide range of computer vision tasks, including both generative ones and more importantly discriminative ones. We first train an encoder by considering the pretrained StyleGAN generator as a learned loss function. The visual features produced by our encoder, termed as Generative Hierarchical Features (GH-Feat), highly align with the layer-wise GAN representations, and hence describe the input image adequately from the reconstruction perspective. Extensive experiments support the versatile transferability of GH-Feat across a range of applications, such as image editing, image processing, image harmonization, face verification, landmark detection, layout prediction, image retrieval, etc. We further show that, through a proper spatial expansion, our developed GH-Feat can also facilitate fine-grained semantic segmentation using only a few annotations. Both qualitative and quantitative results demonstrate the appealing performance of GH-Feat.
更多查看译文
关键词
Feature learning,generative adversarial network,generative representation,image editing
AI 理解论文
溯源树
样例
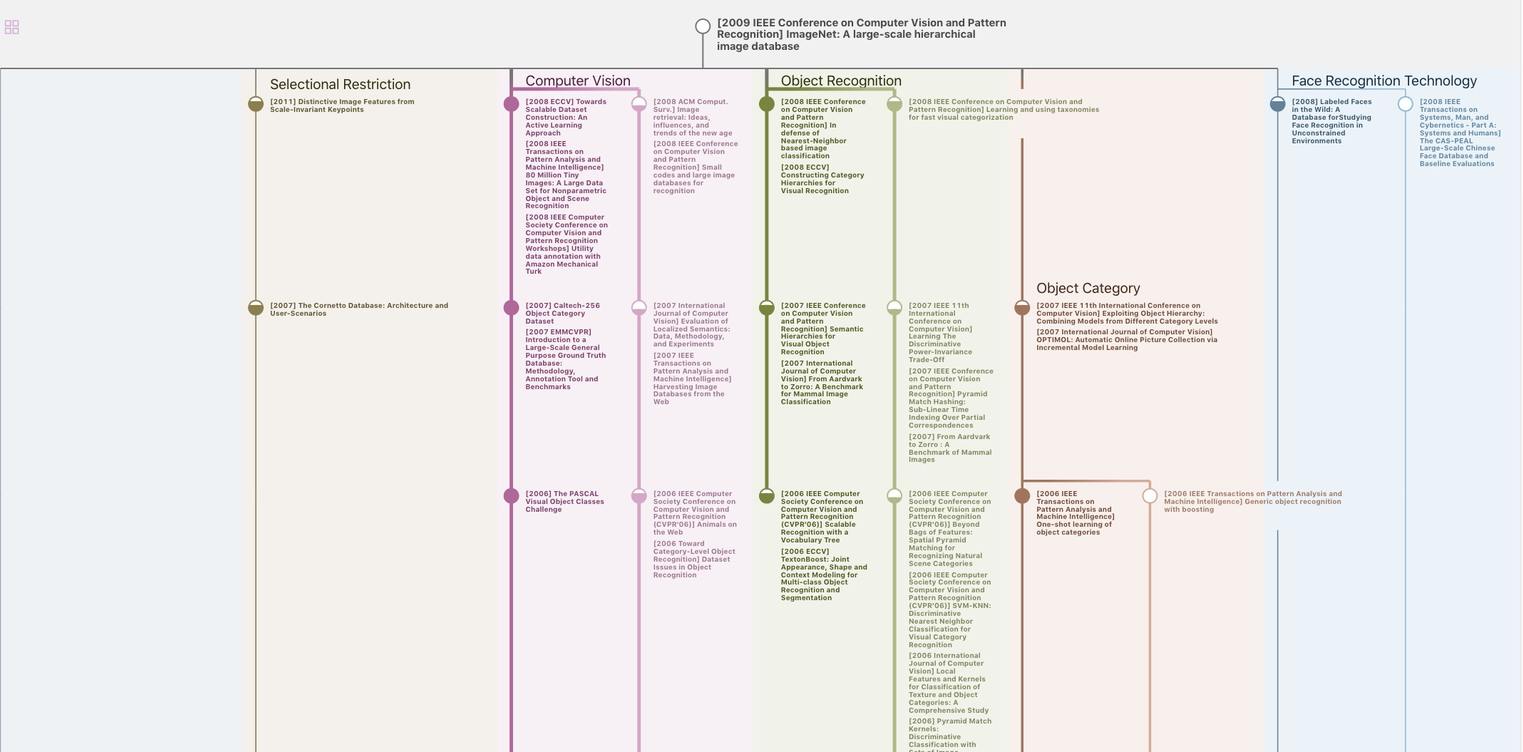
生成溯源树,研究论文发展脉络
Chat Paper
正在生成论文摘要