Enhanced Deep Neural Networks with Transfer Learning for Distribution LMP Considering Load and PV Uncertainties
Social Science Research Network(2022)
摘要
As the flexibility of generation and demand increases in distribution systems, the residential loads are emerging as a promising means to participate in demand response and the transactive energy market. Market pricing is an instrumental mechanism for the distribution system operator to exploit the full potential of the flexible resources. The distribution locational marginal price (DLMP) can be used to guide the residential load consumption. This type of market signal helps the distribution system operator to optimize the scheduling of all resources while satisfying related network constraints through a day-ahead market. However, solving the optimization problem for large-scale systems can be computationally expensive. To address the scalability and practicability limitations of the DLMP framework, a learning-based approach is proposed in this paper to complement the day-ahead distribution market framework. The proposed approach combines long short-term memory and transfer learning to develop deep neural network that can capture the spatial–temporal correlation of the input data. The model can determine the optimal DLMP for each node in a distribution system without the system parameters required to formulate the optimization problem. Testing results on IEEE 33-bus and 123-bus systems show that the proposed approach can generate a comparable DLMP against the optimization solutions.
更多查看译文
关键词
Distribution locational marginal price,Leaning-assisted power system operation,Long short-term memory,Demand response
AI 理解论文
溯源树
样例
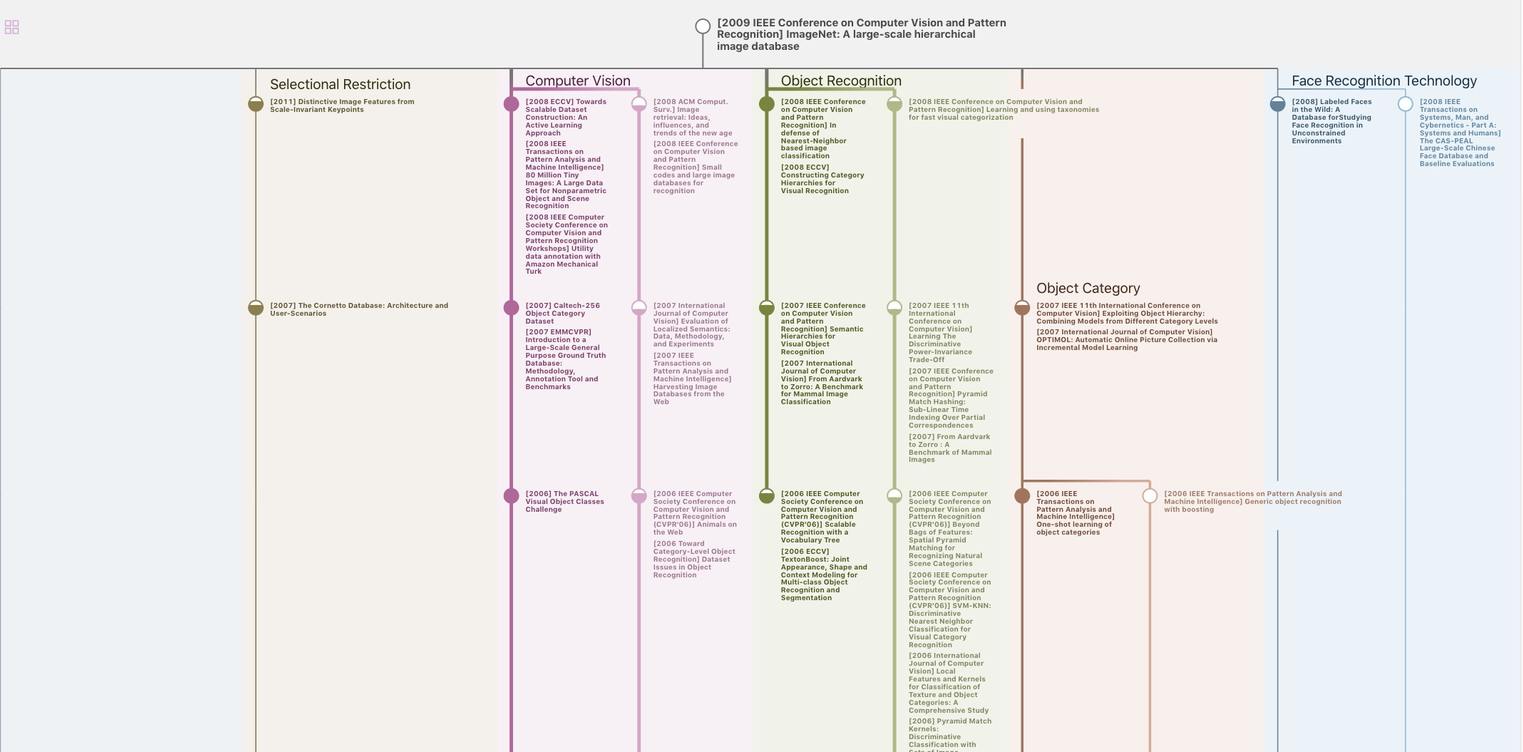
生成溯源树,研究论文发展脉络
Chat Paper
正在生成论文摘要