Detecting Improvised Land-mines using Deep Neural Networks on GPR Image Dataset targeting FPGAs
2022 IEEE Nordic Circuits and Systems Conference (NorCAS)(2022)
摘要
Armed conflicts and guerrilla warfare in different geographical locations have caused great trouble to the communities living in respective territories and affected thousands of human lives in the past. Undiscovered land-mines and explosive devices buried in remote areas are still a big threat as most of such anti-personnel mines are hard to detect with conventional methods owing to their improvisational nature of build and assembly and lack of data. In this paper, a methodological workflow is presented to classify and detect such improvised explosive devices (IEDs) using machine learning by virtue of a Ground Penetrating Radar (GPR) based simulator-driven dataset of mines, while targeting Xilinx MPSoC FPGA device. For enabling on-field embedded deployment of machine learning models, certain deep convolutional neural network architectures and models are trained, quantized and compiled for Xilinx Deep-Learning Processing Unit (DPU) on a Zynq UltraScale+ MPSoC ZU3EG. Experiments yield a maximum hardware deployed model accuracy of 89.46%, maximum precision of 95.21% and a recall of 85.28% for InceptionV3. Whereas, MobileNet with a comparatively lower accuracy of 84.35%, provided the best latency of 7.94 ms for inferencing a single image on DPU as compared to InceptionV3 which required 27.03 ms. Such an embedded setup to detect aforementioned type of land-mines is intended to render the possibility of mobility e.g. either for hand-held devices with GPR sensors or similar arrangements on mobile platforms such as autonomous robots.
更多查看译文
关键词
Improvised Explosive Devices (IEDs),Land mines,Mine Simulation,Supervised Learning,Convolutional Neural Networks (CNNs),Residual Neural Networks,Hardware Prototyping,Field Programmable Gate Arrays (FPGAs),Ground Penetrating Radar (GPR),Deep-learning Processing Unit (DPU)
AI 理解论文
溯源树
样例
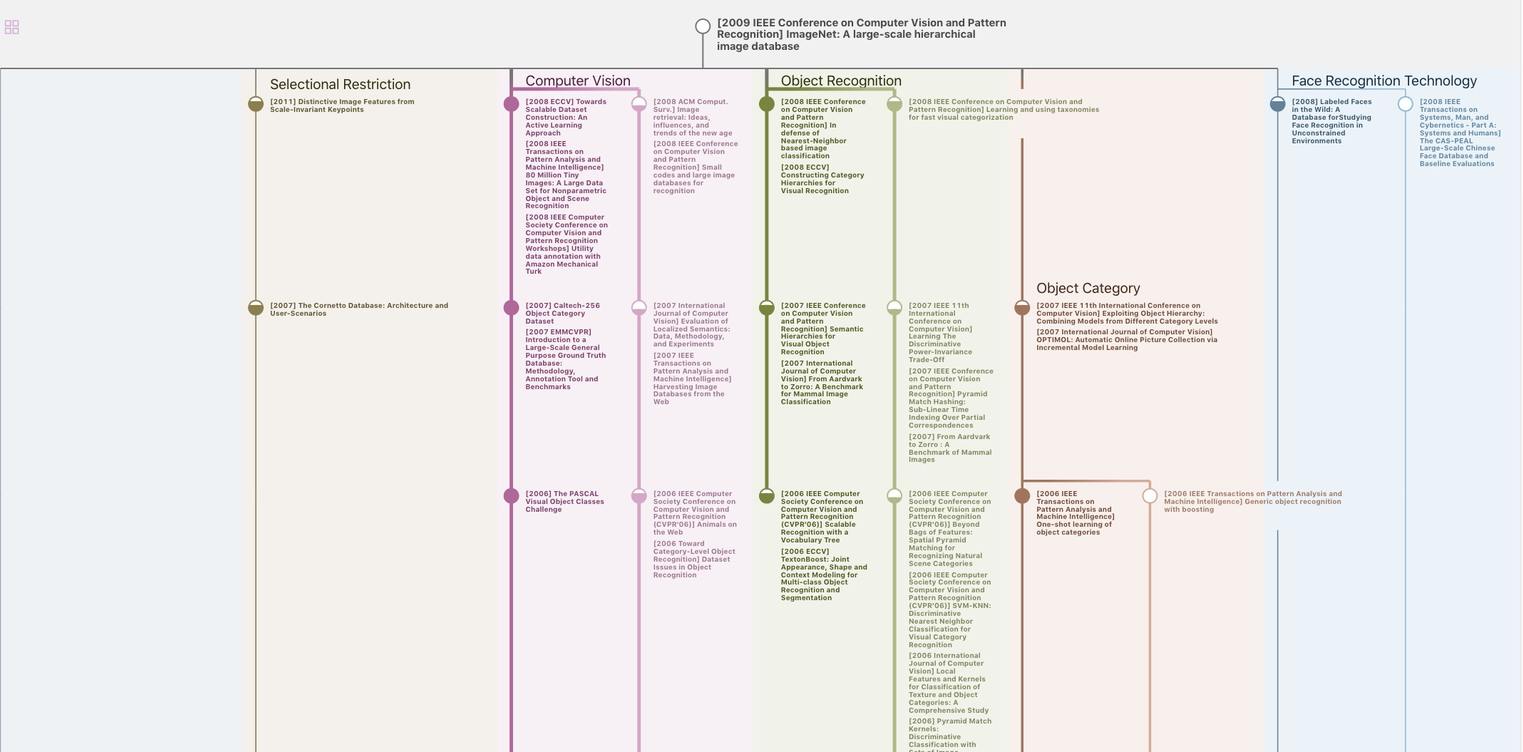
生成溯源树,研究论文发展脉络
Chat Paper
正在生成论文摘要