Astronomical Objects Classification by Convolutional Neural Network Algorithms Layers
2022 New Trends in Signal Processing (NTSP)(2022)
摘要
Our work focuses on application of modern experimental Machine Learning (ML) algorithms toward the space objects classification. Two types of data are analyzed, frame objects present on the astronomical Flexible Image Transport System (FITS) frames and space objects’ light curves, which could be considered as a footprint for given object. In our work we will present ML algorithm used for recognition of frame objects present in the FITS frames by using their specific shape. Presented algorithm is a Convolutional Neural Network (CNN) of 9 layers. The input to the network is a small 50x50 image which must contain only one object for the network to correctly classify it. This could later be used as subnet in region-based CNN after finding regions of interest in full FITS image. Additionally, we present results of applying CNN neural network based on ResNet architecture to classify light curves to categories based on their shape. For deep learning we used primarily public catalogue light curves of selected populations of upper stages e.g., Falcon 9, Atlas Centaur 5, Delta 4 which usually contain simpler features in their photometric series. The modeling software Blender was also used to generate synthetic light curves for training purposes. Algorithm can identify correctly more than 84% of tested objects. In near future we plan to extend the algorithm to identify more complex objects such as box-wing and single box-wing satellites.
更多查看译文
关键词
light curves,frame objects,classification,machine learning
AI 理解论文
溯源树
样例
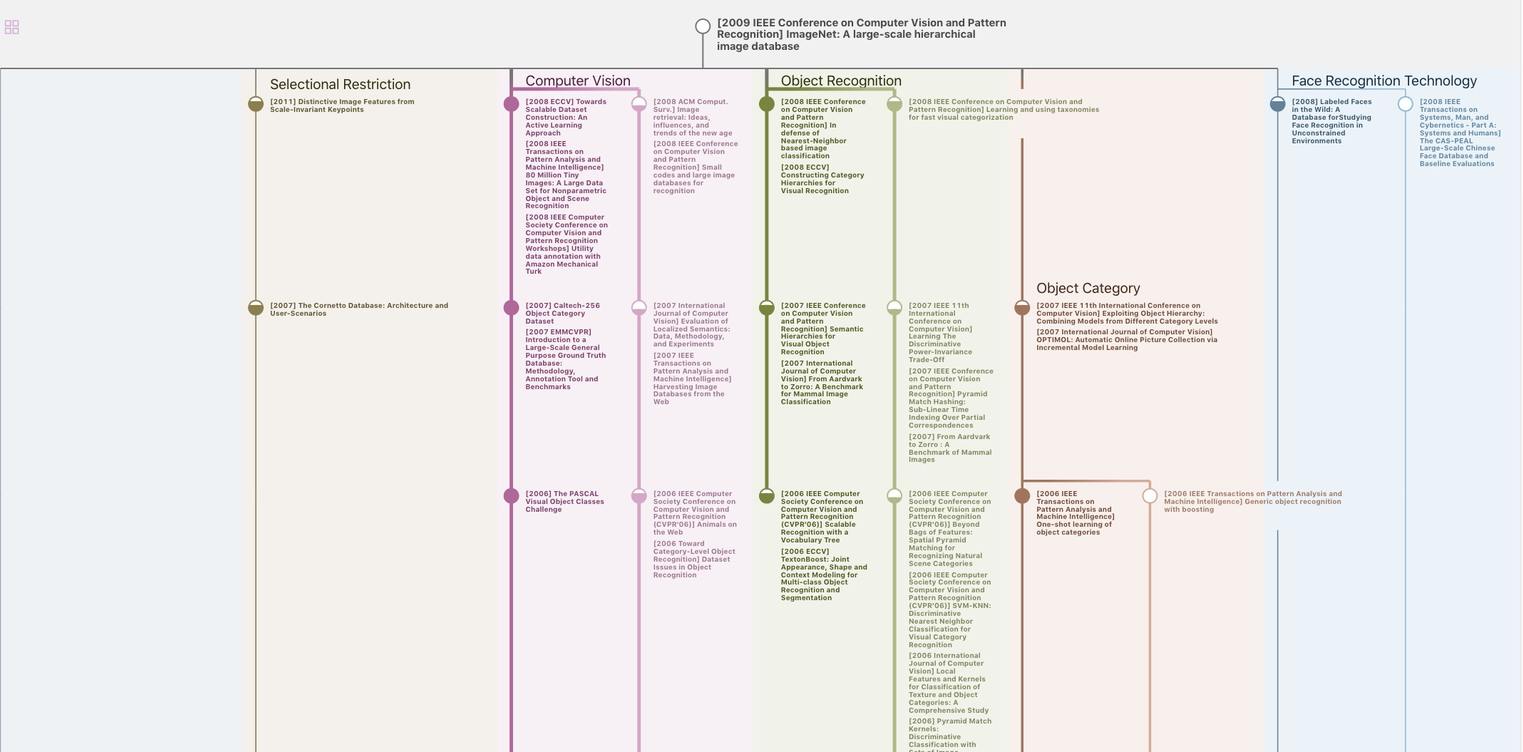
生成溯源树,研究论文发展脉络
Chat Paper
正在生成论文摘要