Noninformative Prior Weights for Dirichlet PDFs*
2022 IEEE International Conference on Multisensor Fusion and Integration for Intelligent Systems (MFI)(2022)
摘要
The noninformative prior weight W of a Dirichlet PDF (Probability Density Function) determines the balance between the prior probability and the influence of new observations on the posterior probability distribution. In this work, we propose a method for dynamically converging the weight W in a way that satisfies two constraints. The first constraint is that the prior Dirichlet PDF (i.e. in the absence of evidence) must always be uniform, which dictates that W = k where k is the cardinality of the domain. The second constraint is that the prior weight of large domains must not be so heavy that it prevents new observation evidence from having the expected influence over the shape of the Dirichlet PDF, which dictates that W quickly converges to a low constant C
W
in the presence of observation evidence, where typically C
W
= 2. In the case of a binary domain, the noninformative prior weight is normally set to W = 2, irrespective of the amount of evidence. In the case of a multidimensional domain with arbitrarily large cardinality k, the noninformative prior weight is initially equal to the domain cardinality k, but rapidly decreases to the constant convergence factor C
W
as the amount of evidence increases.
更多查看译文
关键词
probability density function,posterior probability distribution,Dirichlet PDF
AI 理解论文
溯源树
样例
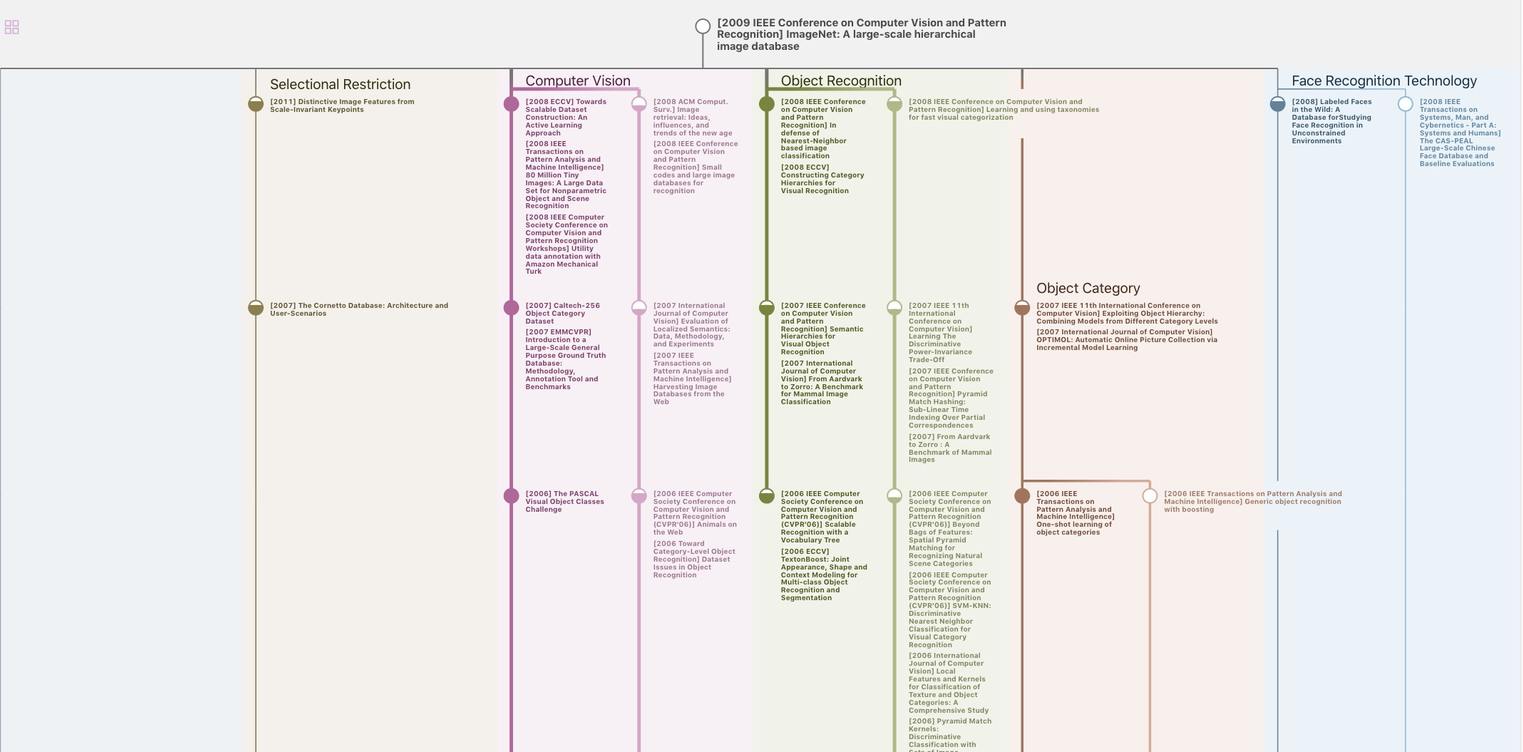
生成溯源树,研究论文发展脉络
Chat Paper
正在生成论文摘要