Optimizing CABAC architecture with prediction based context model prefetching
2022 IEEE 24th International Workshop on Multimedia Signal Processing (MMSP)(2022)
摘要
Context Adaptive Binary Arithmetic Coding (CABAC) is the entropy coding module widely used in recent video coding standards such as HEVC/H.265 and VVC/H.266. CABAC is a well-known throughput bottleneck due to its strong data dependencies. Because the required context model of the current bin often depends on the results of the previous bin, the context model cannot be prefetched early enough, and then costs pipeline stalls. To solve this problem, we propose a prediction-based context model prefetching strategy. If the prediction is correct, pipeline stalls can be eliminated, and the stalling cycles won't get worse with the wrong prediction. Moreover, the data interaction process between CABAC modules and the multi-stage pipeline structure are optimized to maximize the working frequency. The proposed pipeline architecture can reduce pipeline stalls and save up to 45.66% encoding time, the improved results show that it provides more significant gains in All Intra (AI) under low QP test conditions, which is better than the Random Access (RA) and Low Delay (LD) configuration. The highest hardware efficiency (Mbins/s Per k gates) is higher than the existing advanced pipeline architecture.
更多查看译文
关键词
HEVC,Entropy coding,CABAC,Video En-coder,Hardware Desig
AI 理解论文
溯源树
样例
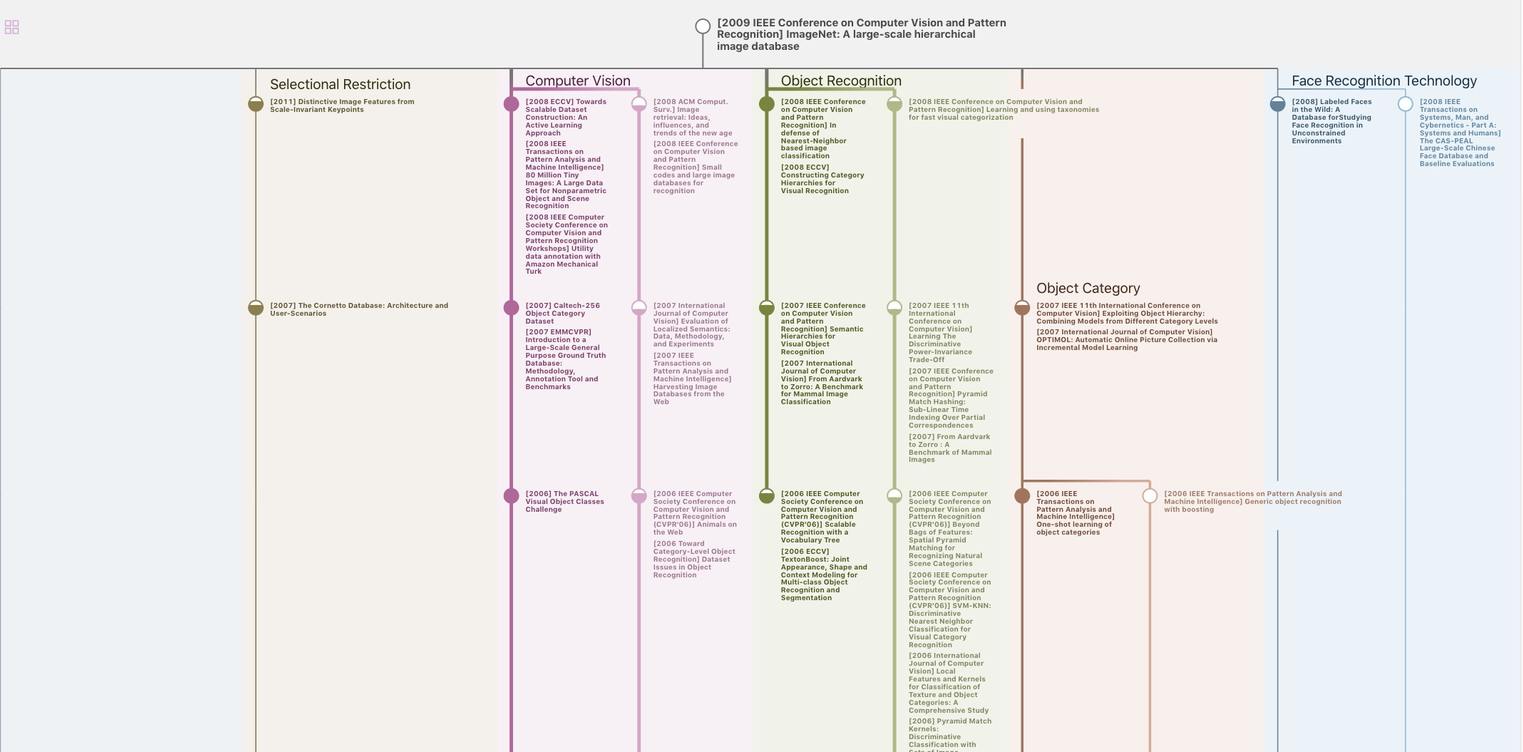
生成溯源树,研究论文发展脉络
Chat Paper
正在生成论文摘要