A Comparative Analysis of Deep Learning Methods for Emotion Recognition using Physiological Signals for Robot-Based Intervention Studies
2022 Medical Technologies Congress (TIPTEKNO)(2022)
摘要
Robot-based intervention systems can be more pros-perous if they recognize the patients' and children's emotions and modify the interaction scenarios considering these emotions to increase their engagement. This study aims to develop an emotion recognition model that uses users' physiological signals, which is planned to be integrated into robot-based intervention studies. Deep learning methods can be used to develop emotion recognition models using physiological signals, which can be collected from different biofeedback sensors. In this study, a comparative analysis of deep learning methods for emotion recog-nition using physiological signals for robot-based intervention studies are performed. Convolutional Neural networks (CNN) and Long Short-Term Memory (LSTM) networks are employed to analyze and classify the physiological data (blood volume pulse (BVP), skin temperature (ST), and skin conductance (SC)). Furthermore, the effects of different hyperparameters (filter size, number of filters, and dropout) on the classification performance of the emotion recognition models are analyzed. It has been found that the best performance among the proposed models is the hybrid model CLS (hybrid network with Support Vector Machine (SVM) classifier), where the filter size is 3X3 with the BVP sensor (59.18%) in the pleasant-unpleasant (PU) classification. Furthermore, the hybrid model Dec-CLS contains 3 convolutional layers with 32, 16, and 8 3X3 filters, respectively, result in the highest performance (67.46%) with the BVP sensor in NU (neutral- unpleasant) classification.
更多查看译文
关键词
sensor fusion,emotion recognition,robot-based intervention system,physiological signal,deep learning
AI 理解论文
溯源树
样例
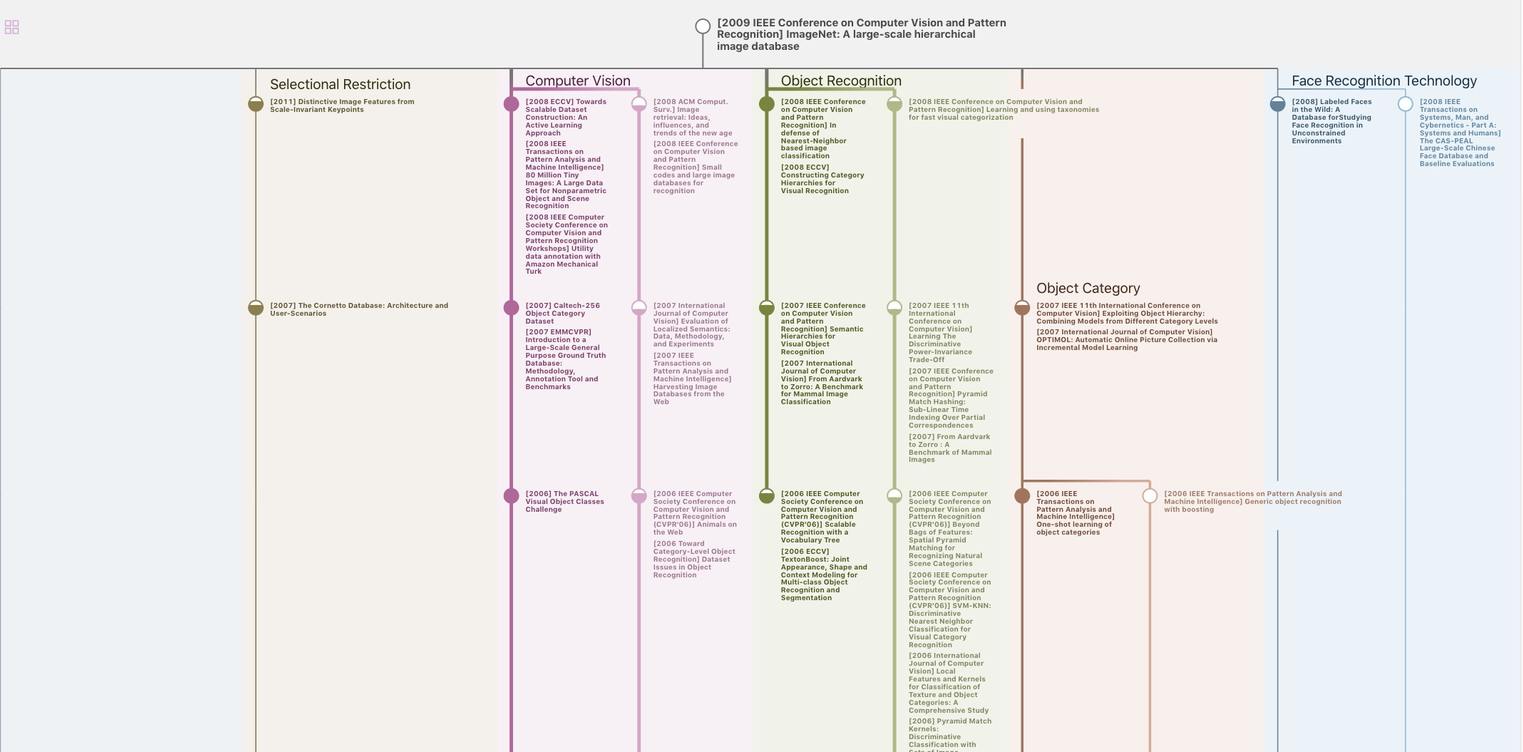
生成溯源树,研究论文发展脉络
Chat Paper
正在生成论文摘要