Perturbed Multiplicative Weights Update in Learning of Game
2022 International Conference on Machine Learning, Cloud Computing and Intelligent Mining (MLCCIM)(2022)
摘要
Multiplicative Weights Update (MWU) has been studied extensively due to many applications in machine learning, constrained optimization, and game theory. In the non-convex optimization problems, saddle points are the main obstruction to produce high-quality optimizers, i.e., the critical points that is not local or global optima. Recent works in optimization and machine learning has addressed the escaping saddle point problem in a promise way-adding perturbation to iterations. Despite the popularity of MWU and perturbed first-order methods, there is a problem remains unaddressed: is there a perturbed version of MWU algorithms so that the algorithm can escape saddle points efficiently. This paper focuses on a spherical framework for MWU and its application in building result on escaping saddle points. Combining with the recent development on escaping saddle points with perturbed Riemannian gradient descent, we derive a non-asymptotic convergence results for perturbed MWU.
更多查看译文
关键词
Machine Learning,Perturbed gradient descent,multiplicative weights update,escaping saddle points
AI 理解论文
溯源树
样例
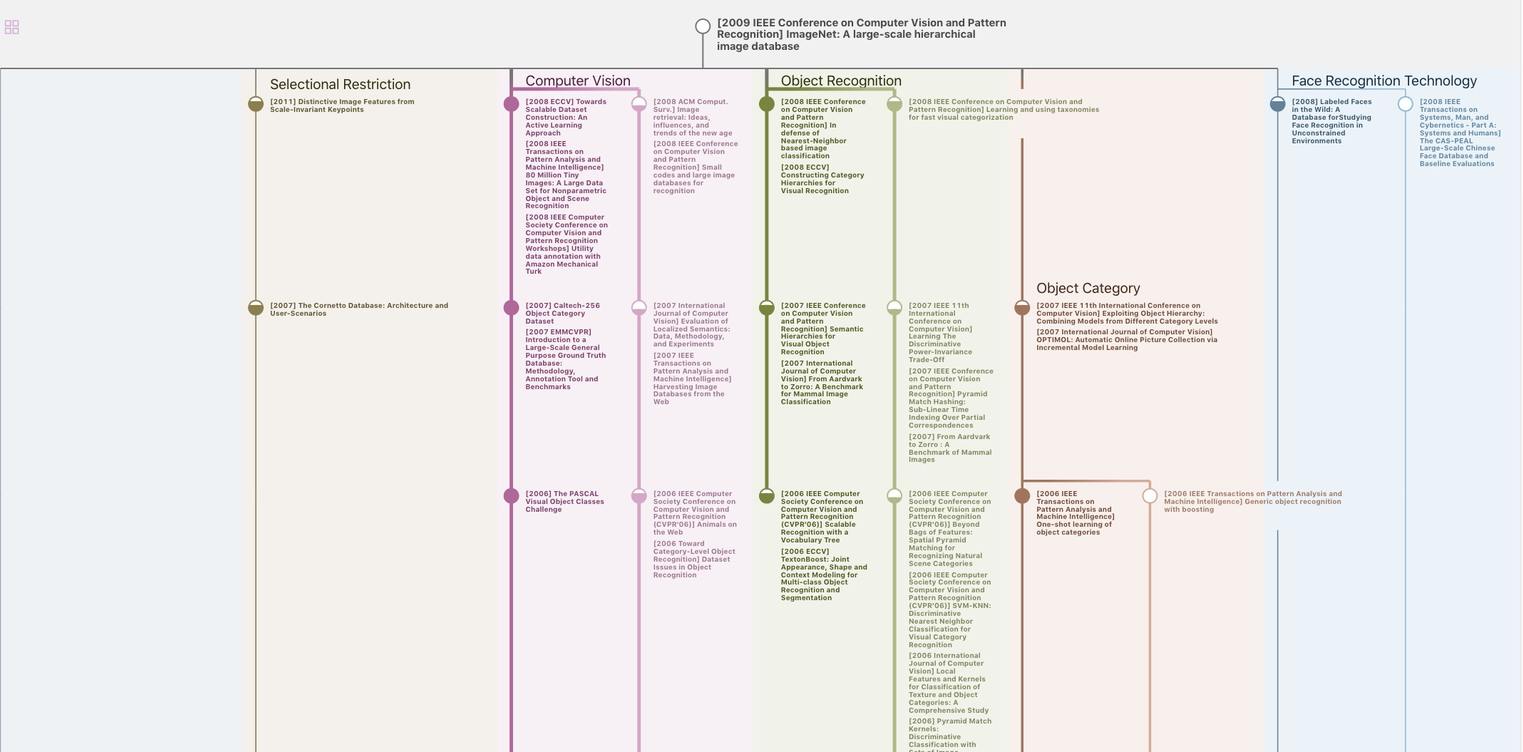
生成溯源树,研究论文发展脉络
Chat Paper
正在生成论文摘要