Efficient Backbone Architecture Search for Stereo Depth Estimation in Autonomous Driving
2022 IEEE 25th International Conference on Intelligent Transportation Systems (ITSC)(2022)
摘要
Recent advances in AutoML have extended Neural Architecture Search (NAS) beyond image classification to optimize dense prediction tasks. However, the existing works are inappropriate to search efficient backbone for deep learning based stereo matching, because their search spaces are not custom-designed according to the inherent requirements of the pixel-wise depth prediction. This paper proposes a differentiable architecture search specific for efficient stereo network backbone. In particular, the proposed method jointly optimizes the micro-architecture and the macro-architecture to search distinct cell structures and adaptive low-level features for stereo network backbone. The target architecture can be found within 3 GPU days using gradient-based optimization. The evaluation results on stereo datasets demonstrate that, by simply replacing the hand-crafted feature extraction with the searched backbone in a vanilla framework, the proposed network obtains much better disparity accuracy than the designs using existing NAS methods, and even achieves comparable performance compared with the state-of-the-art stereo networks that integrate various elaborate modules. Hence, the proposed NAS method is an efficient way to automate the stereo network architecture engineering.
更多查看译文
关键词
microarchitecture,macro-architecture,distinct cell structures,low-level features,target architecture,stereo datasets,hand-crafted feature extraction,searched backbone,existing NAS methods,state-of-the-art stereo networks,stereo network architecture engineering,efficient backbone Architecture Search,stereo depth estimation,autonomous driving,Neural Architecture Search,image classification,dense prediction tasks,deep learning,stereo matching,search spaces,pixel-wise depth prediction,differentiable architecture search specific,efficient stereo network backbone
AI 理解论文
溯源树
样例
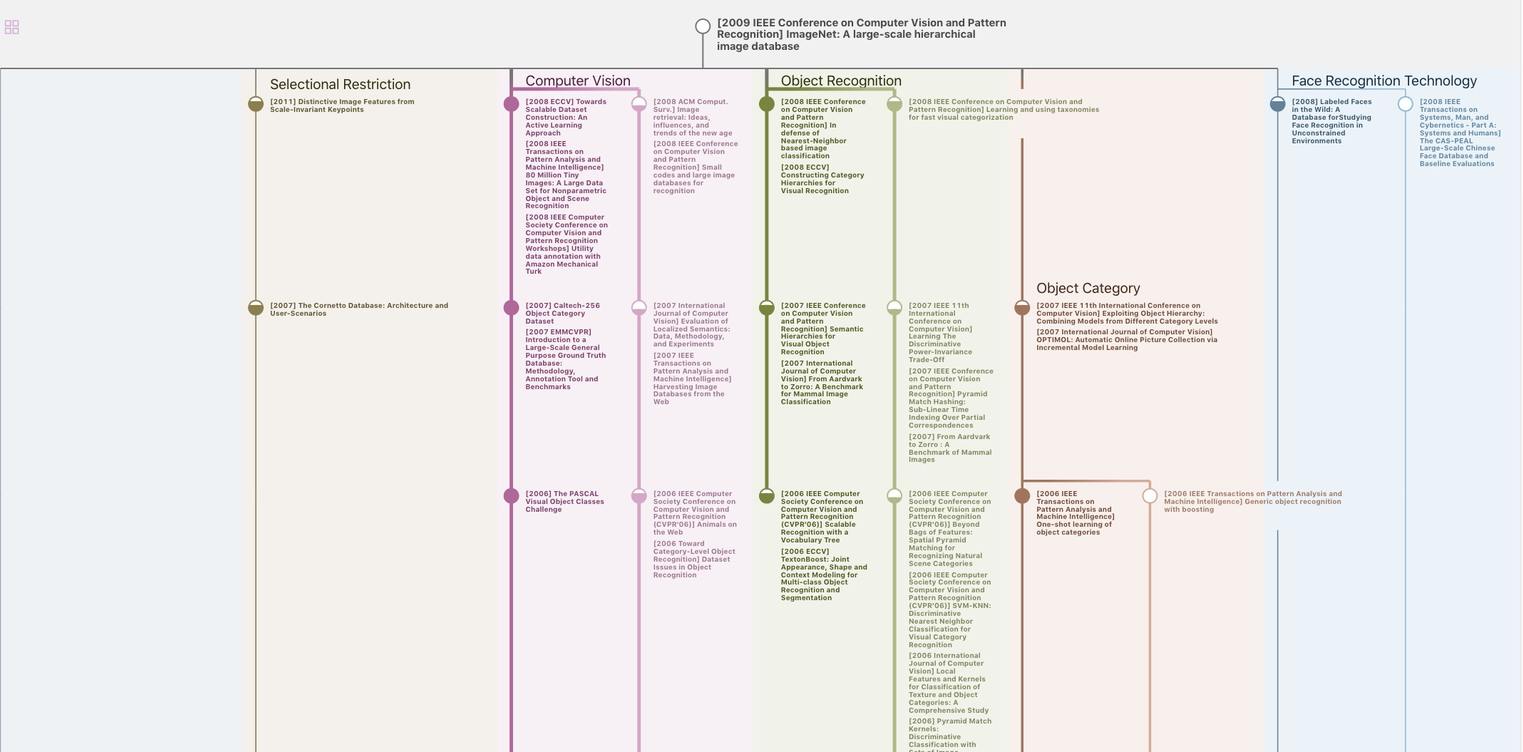
生成溯源树,研究论文发展脉络
Chat Paper
正在生成论文摘要